Influence of Color Spaces for Deep Learning Image Colorization
HANDBOOK OF MATHEMATICAL MODELS AND ALGORITHMS IN COMPUTER VISION AND IMAGING: Mathematical Imaging and Vision(2022)
摘要
Colorization is a process that converts a grayscale image into a colored one that looks as natural as possible. Over the years this task has received a lot of attention. Existing colorization methods rely on different color spaces: RGB, YUV, Lab, etc. In this chapter, we aim to study their influence on the results obtained by training a deep neural network, to answer the following question: "Is it crucial to correctly choose the right color space in deep learning-based colorization?" First, we briefly summarize the literature and, in particular, deep learning-based methods. We then compare the results obtained with the same deep neural network architecture with RGB, YUV, and Lab color spaces. Qualitative and quantitative analysis do not conclude similarly on which color space is better. We then show the importance of carefully designing the architecture and evaluation protocols depending on the types of images that are being processed and their specificities: strong/small contours, few/many objects, recent/archive images.
更多查看译文
关键词
Image colorization,Deep learning,Color spaces
AI 理解论文
溯源树
样例
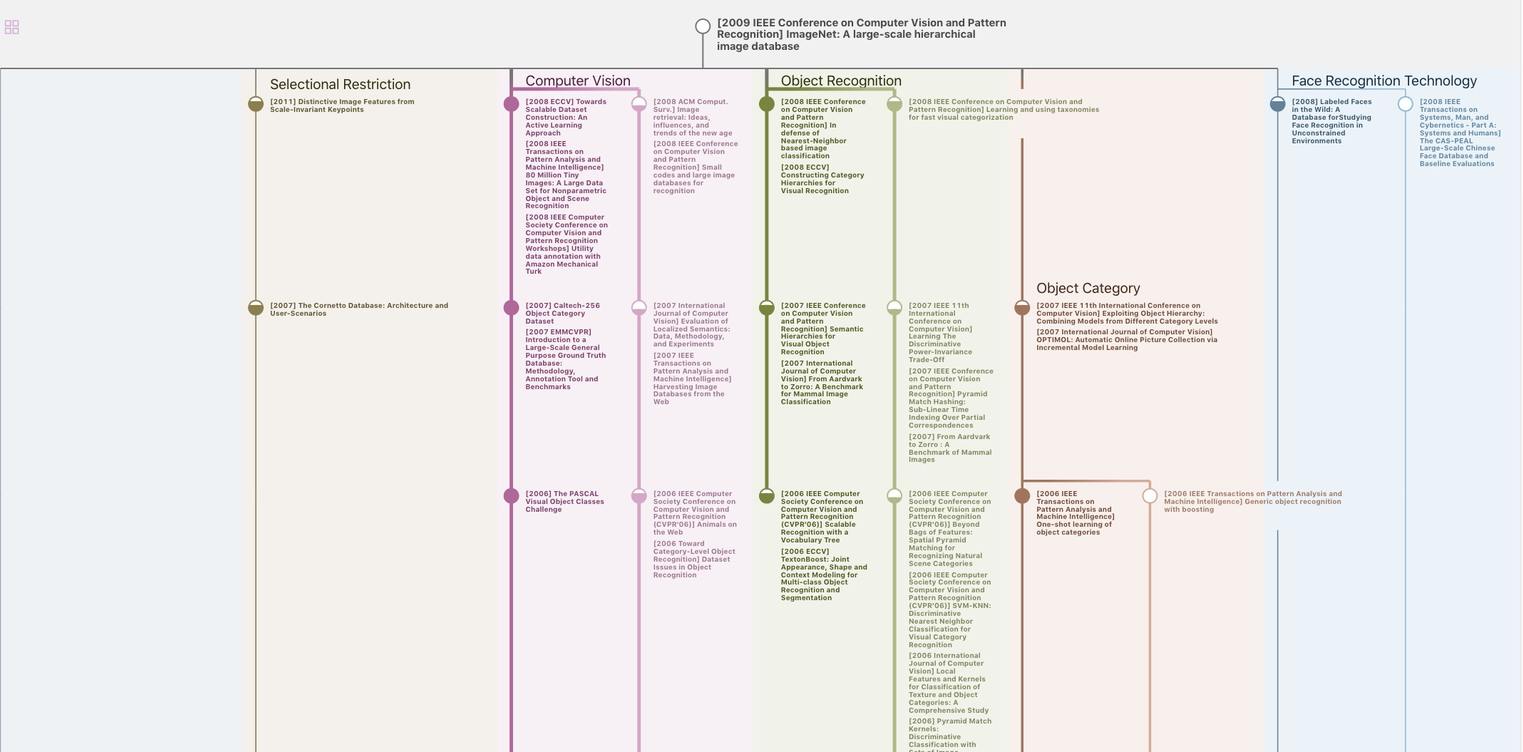
生成溯源树,研究论文发展脉络
Chat Paper
正在生成论文摘要