Behavioral uncertainty quantification for data-driven control
arxiv(2022)
摘要
This paper explores the problem of uncertainty quantification in the behavioral setting for data-driven control. Building on classical ideas from robust control, the problem is regarded as that of selecting a metric which is best suited to a data-based description of uncertainties. Leveraging on Willems' fundamental lemma, restricted behaviors are viewed as subspaces of fixed dimension, which may be represented by data matrices. Consequently, metrics between restricted behaviors are defined as distances between points on the Grassmannian, i.e., the set of all subspaces of equal dimension in a given vector space. A new metric is defined on the set of restricted behaviors as a direct finite-time counterpart of the classical gap metric. The metric is shown to capture parametric uncertainty for the class of autoregressive (AR) models. Numerical simulations illustrate the value of the new metric with a data-driven mode recognition and control case study.
更多查看译文
关键词
behavioral uncertainty quantification,data matrices,data-driven control,data-driven mode recognition,fixed dimension,fundamental lemma,gap metric,parametric uncertainty,restricted behaviors,robust control
AI 理解论文
溯源树
样例
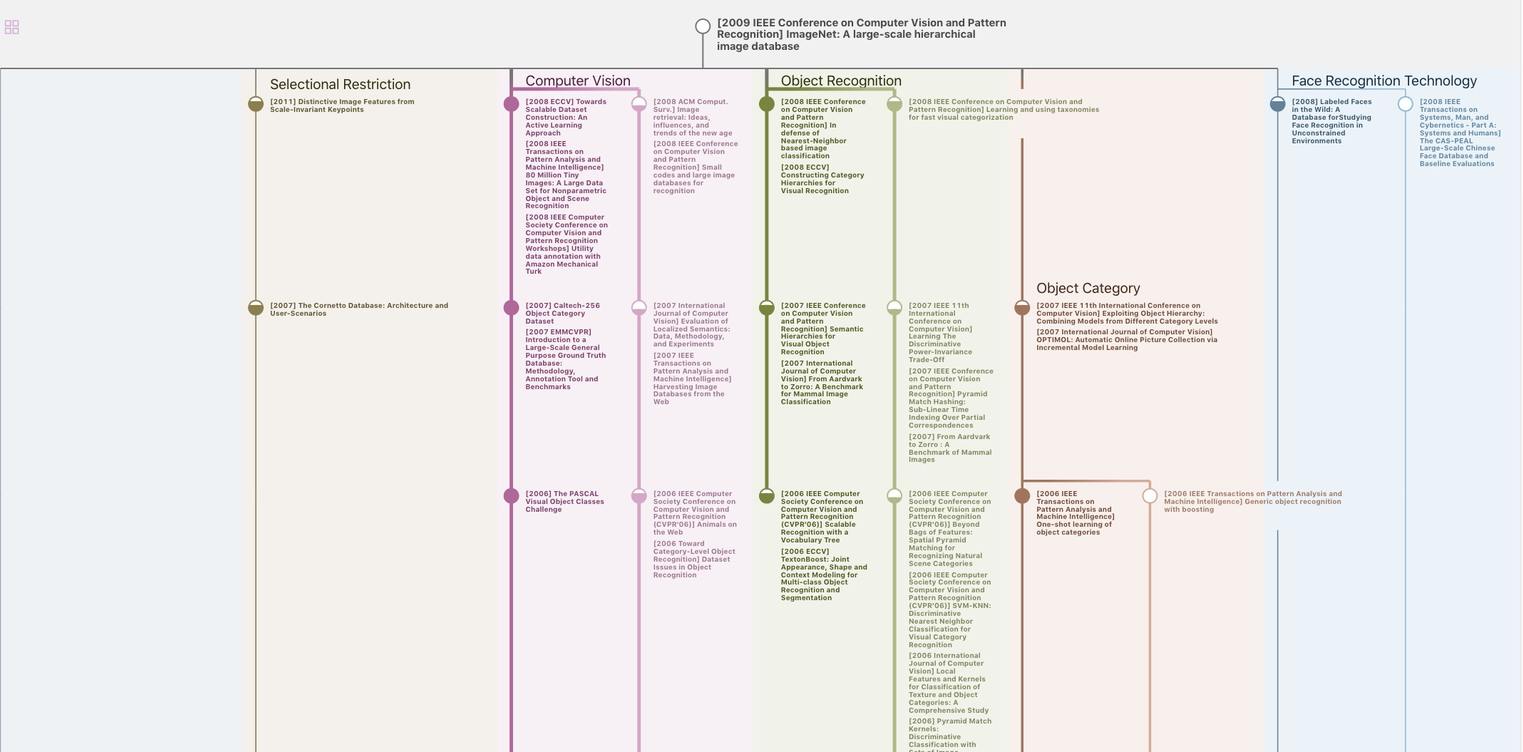
生成溯源树,研究论文发展脉络
Chat Paper
正在生成论文摘要