Double sampling and semiparametric methods for informatively missing data
arxiv(2022)
Abstract
Missing data arise almost ubiquitously in applied settings, and can pose a substantial threat to the validity of statistical analyses. In the context of comparative effectiveness research, such as in large observational databases (e.g., those derived from electronic health records), outcomes may be missing not at random with respect to measured covariates. In this setting, we propose a double sampling method, in which outcomes are obtained via intensive follow-up on a subsample of subjects for whom data were initially missing. We describe assumptions under which the joint distribution of confounders, treatment, and outcome is identified under this design, and derive efficient estimators of the average treatment effect under a nonparametric model, as well as a model assuming outcomes were initially missing at random. We compare these in simulations to an approach that adaptively selects an estimator based on evidence of violation of the missing at random assumption. We also show that the proposed double sampling design can be extended to handle arbitrary coarsening mechanisms, and derive consistent, asymptotically normal, and nonparametric efficient estimators of any smooth full data functional of interest, and prove that these estimators often are multiply robust.
MoreTranslated text
AI Read Science
Must-Reading Tree
Example
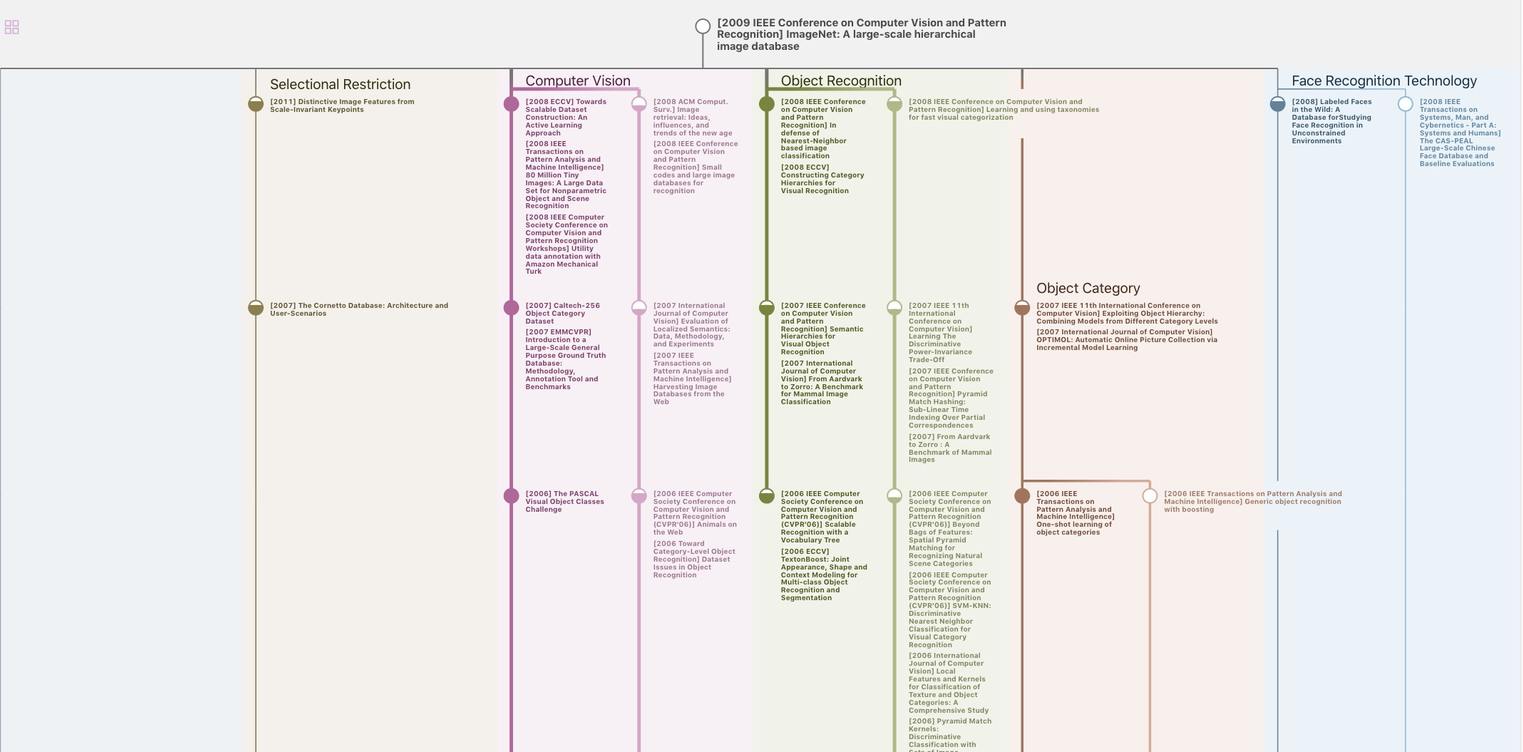
Generate MRT to find the research sequence of this paper
Chat Paper
Summary is being generated by the instructions you defined