Information theory characteristics improve the prediction of lithium response in bipolar disorder patients using an SVM classifier
bioRxiv(2022)
摘要
Bipolar disorder (BD) is a mood disorder with a high morbidity and death rate. Lithium (Li), a prominent mood stabilizer, is fully effective in roughly 30% of BD patients. The remaining patients respond partially or do not respond at all. Another drug used to treat BD patients is valproate (VPA). Plenty of efforts has been made to understand how these drugs affect the patients’ neurons. We have performed electrophysiological recordings in patient-derived dentate gyrus (DG) granule neurons for three groups: control individuals, BD patients who respond to Li treatment (LR), and BD patients who do not respond to Li treatment (NR). The recordings were analyzed by the statistical tools of modern information theory, which enabled us to recognize new relationships between the electrophysiological features. These added features included the entropy of several electrophysiological measurements and the mutual information between different types of electrophysiological measurements. Information theory features provided further knowledge about the distribution of the electrophysiological entities, which improved basic classification schemes. These newly added features enabled a significant improvement in our ability to distinguish the BD patients from the control individuals (an improvement from 60% accuracy to 74% accuracy) and the Li responders from the non-responders in the BD population using Support Vector Machine (SVM) classification algorithms (an improvement from 81% accuracy to 99% accuracy). These new tools showed that LR neurons are less distinguishable from control neurons after Li treatment but not after VPA treatment, whereas NR neurons become more distinguishable from control neurons after Li treatment.
### Competing Interest Statement
The authors have declared no competing interest.
更多查看译文
关键词
lithium response,bipolar disorder patients,information theory characteristics,svm
AI 理解论文
溯源树
样例
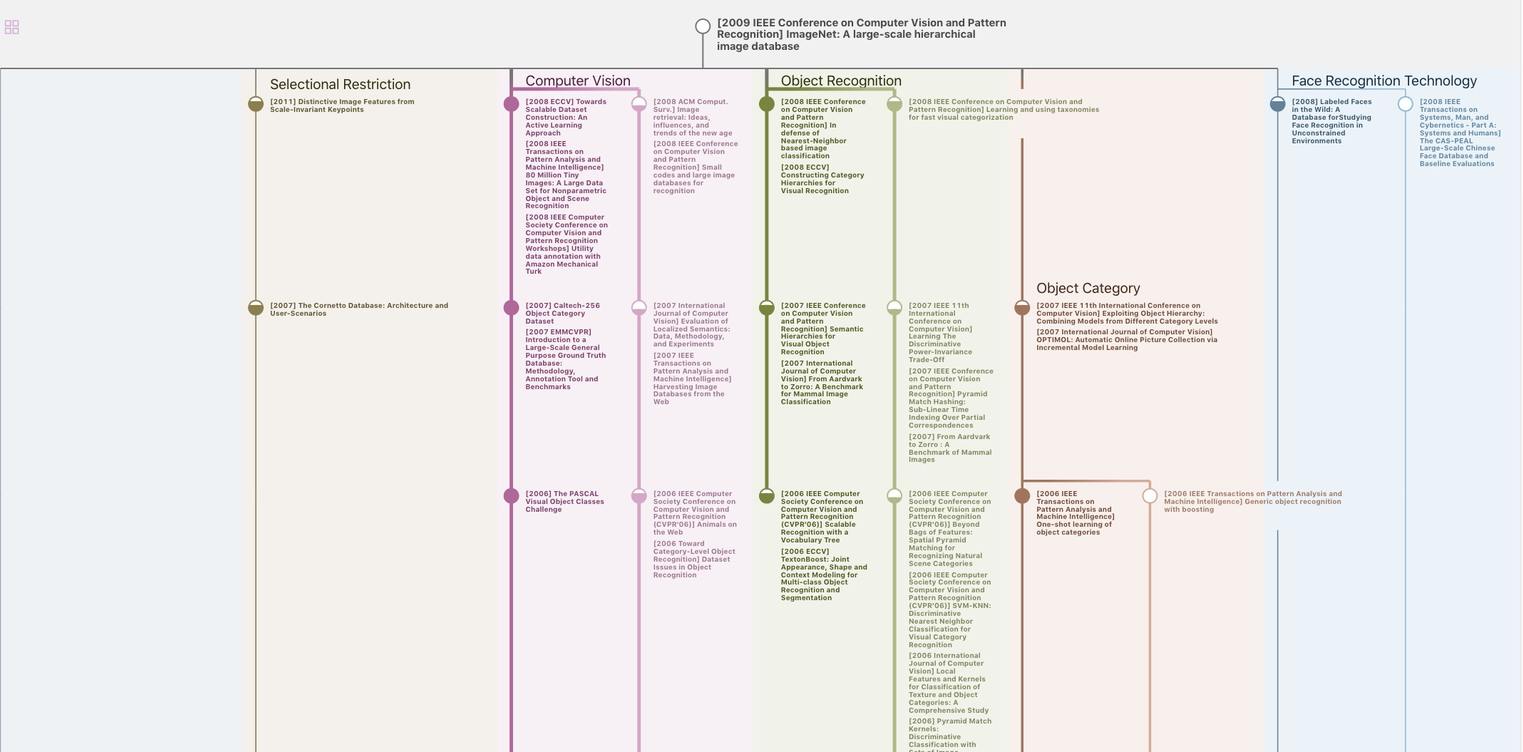
生成溯源树,研究论文发展脉络
Chat Paper
正在生成论文摘要