Methods for Combining Probability and Nonprobability Samples Under Unknown Overlaps
arXiv (Cornell University)(2022)
摘要
Nonprobability (convenience) samples are increasingly sought to stabilize estimations for one or more population variables of interest that are performed using a randomized survey (reference) sample by increasing the effective sample size. Estimation of a population quantity derived from a convenience sample will typically result in bias since the distribution of variables of interest in the convenience sample is different from the population. A recent set of approaches estimates conditional (on sampling design predictors) inclusion probabilities for convenience sample units by specifying reference sample-weighted pseudo likelihoods. This paper introduces a novel approach that derives the propensity score for the observed sample as a function of conditional inclusion probabilities for the reference and convenience samples as our main result. Our approach allows specification of an exact likelihood for the observed sample. We construct a Bayesian hierarchical formulation that simultaneously estimates sample propensity scores and both conditional and reference sample inclusion probabilities for the convenience sample units. We compare our exact likelihood with the pseudo likelihoods in a Monte Carlo simulation study.
更多查看译文
关键词
nonprobability samples,probability
AI 理解论文
溯源树
样例
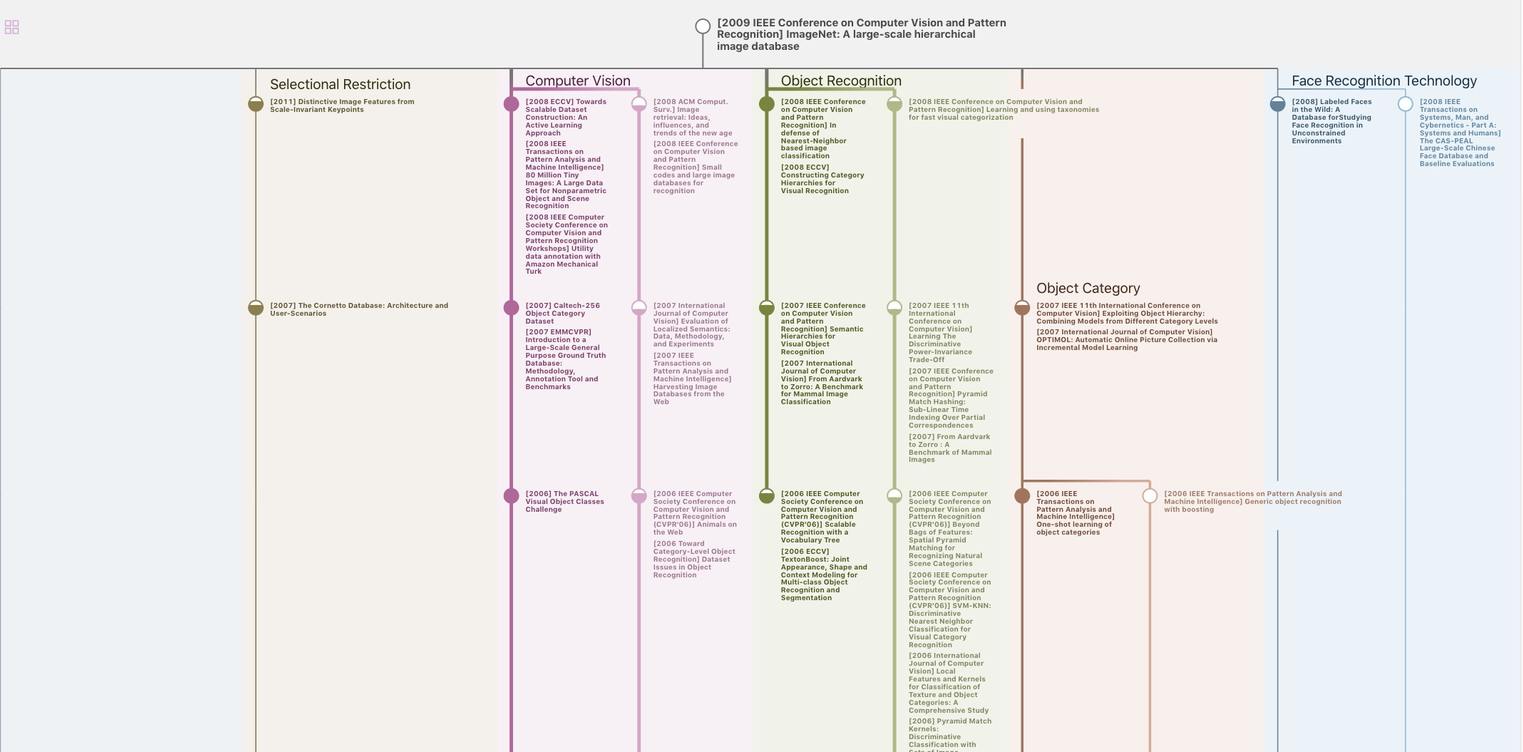
生成溯源树,研究论文发展脉络
Chat Paper
正在生成论文摘要