SHAIL: Safety-Aware Hierarchical Adversarial Imitation Learning for Autonomous Driving in Urban Environments
2023 IEEE INTERNATIONAL CONFERENCE ON ROBOTICS AND AUTOMATION, ICRA(2023)
摘要
Designing a safe and human-like decision-making system for an autonomous vehicle is a challenging task. Generative imitation learning is one possible approach for automating policy-building by leveraging both real-world and simulated decisions. Previous work that applies generative imitation learning to autonomous driving policies focuses on learning a low-level controller for simple settings. However, to scale to complex settings, many autonomous driving systems combine fixed, safe, optimization-based low-level controllers with high-level decision-making logic that selects the appropriate task and associated controller. In this paper, we attempt to bridge this gap in complexity by employing Safety-Aware Hierarchical Adversarial Imitation Learning (SHAIL), a method for learning a high-level policy that selects from a set of low-level controller instances in a way that imitates low-level driving data on-policy. We introduce an urban roundabout simulator that controls non-ego vehicles using real data from the Interaction dataset. We then demonstrate empirically that even with simple controller options, our approach can produce better behavior than previous approaches in driver imitation that have difficulty scaling to complex environments. Our implementation is available at https://github.com/sisl/InteractionImitation.
更多查看译文
关键词
associated controller,automating policy-building,autonomous driving policies,autonomous driving systems,autonomous vehicle,complex settings,decision-making system,driver imitation,driving data,generative imitation learning,high-level decision-making logic,high-level policy,imitates low-level,low-level controller instances,safe optimization-based low-level controllers,Safety-Aware Hierarchical Adversarial Imitation Learning,SHAIL,simple controller options,simulated decisions
AI 理解论文
溯源树
样例
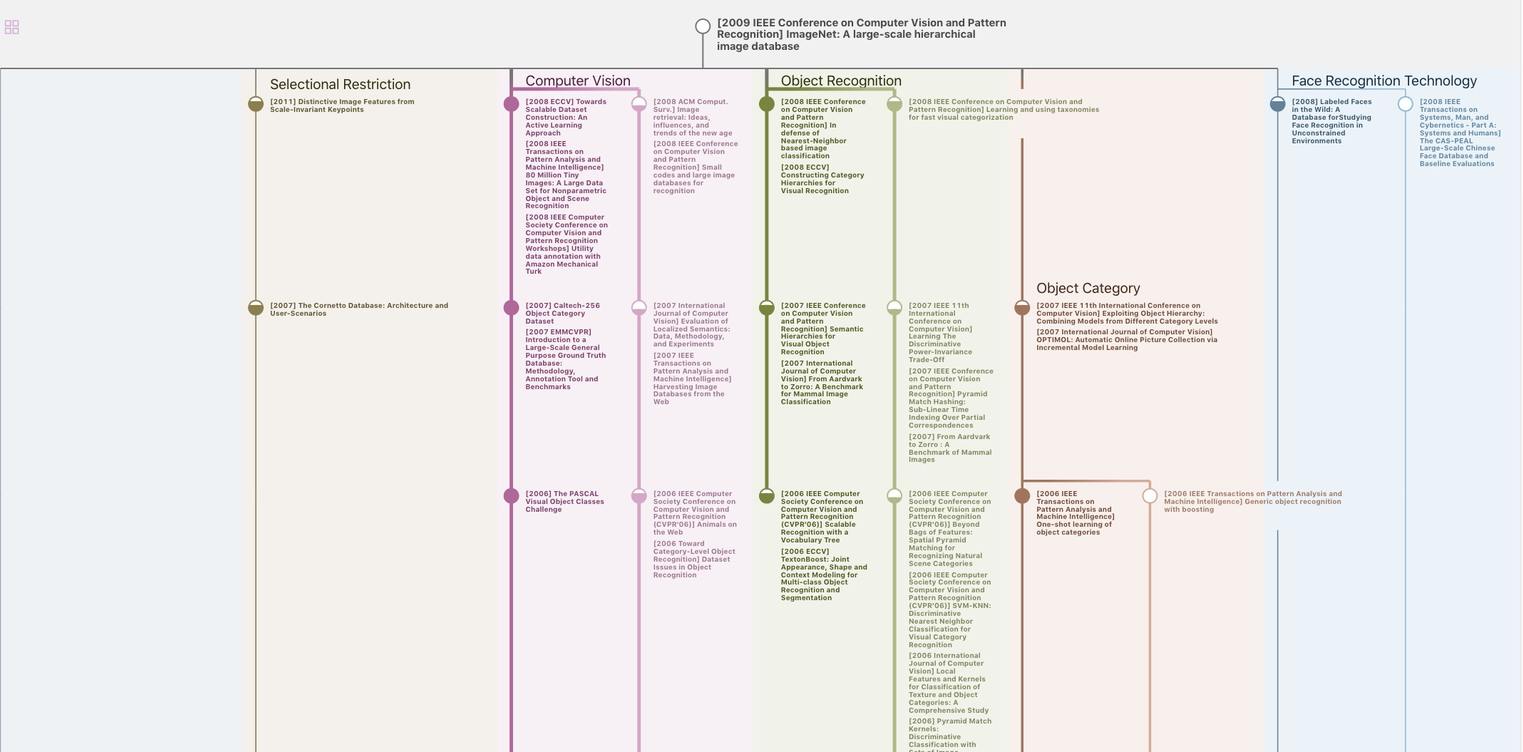
生成溯源树,研究论文发展脉络
Chat Paper
正在生成论文摘要