Deep learning for the rare-event rational design of 3D printed multi-material mechanical metamaterials
COMMUNICATIONS MATERIALS(2022)
Abstract
Emerging multi-material 3D printing techniques enables the rational design of metamaterials with not only complex geometries but also arbitrary distributions of multiple materials within those geometries, yielding unique combinations of elastic properties. However, discovering the rare designs that lead to highly unusual combinations of material properties, such as double-auxeticity and high elastic moduli, remains a non-trivial crucial task. Here, we use computational models and deep learning algorithms to identify rare-event designs. In particular, we study the relationship between random distributions of hard and soft phases in three types of planar lattices and the resulting mechanical properties of the two-dimensional networks. By creating a mapping from the space of design parameters to the space of mechanical properties, we are able to reduce the computational time required for evaluating each design to ≈2.4 × 10 −6 s, and to make the process of evaluating different designs highly parallelizable. We then select ten designs to be 3D printed, mechanically test them, and characterize their behavior using digital image correlation to validate the accuracy of our computational models. Our simulation results show that our deep learning-based algorithms can accurately predict the mechanical behavior of the different designs and that our modeling results match experimental observations.
MoreTranslated text
Key words
deep learning,rare-event,multi-material
AI Read Science
Must-Reading Tree
Example
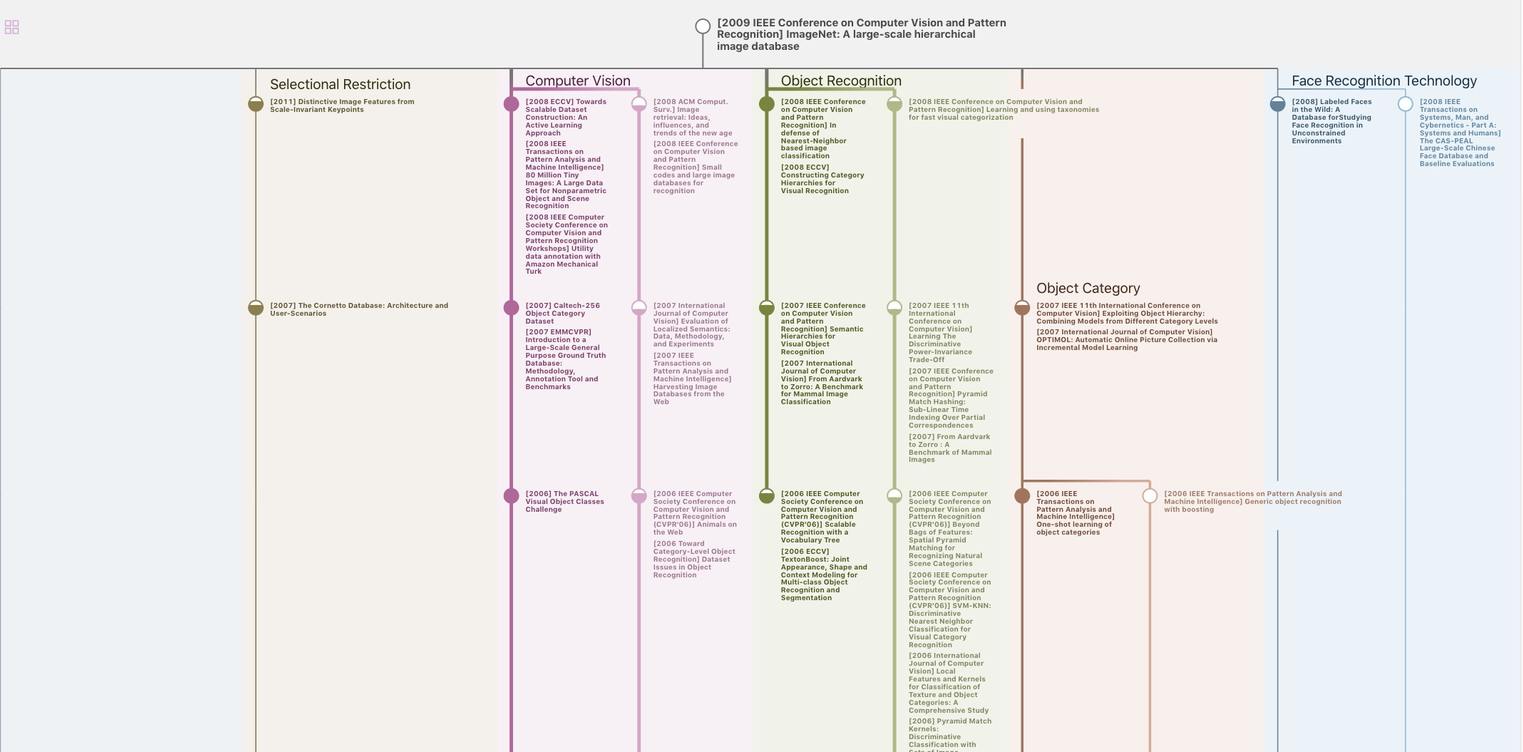
Generate MRT to find the research sequence of this paper
Chat Paper
Summary is being generated by the instructions you defined