LISA: Learning Implicit Shape and Appearance of Hands
IEEE Conference on Computer Vision and Pattern Recognition(2022)
Abstract
This paper proposes a do-it-all neural model of human hands, named LISA. The model can capture accurate hand shape and appearance, generalize to arbitrary hand sub-jects, provide dense surface correspondences, be reconstructed from images in the wild, and can be easily an-imated. We train LISA by minimizing the shape and appearance losses on a large set of multi-view RGB image se-quences annotated with coarse 3D poses of the hand skele-ton. For a 3D point in the local hand coordinates, our model predicts the color and the signed distance with respect to each hand bone independently, and then combines the per-bone predictions using the predicted skinning weights. The shape, color, and pose representations are disentangled by design, enabling fine control of the selected hand param-eters. We experimentally demonstrate that LISA can ac-curately reconstruct a dynamic hand from monocular or multi-view sequences, achieving a noticeably higher qual-ity of reconstructed hand shapes compared to baseline approaches. Project page: https://www.iri.upc.edu/people/ecorona/lisa/.
MoreTranslated text
Key words
Face and gestures, 3D from single images, Pose estimation and tracking
AI Read Science
Must-Reading Tree
Example
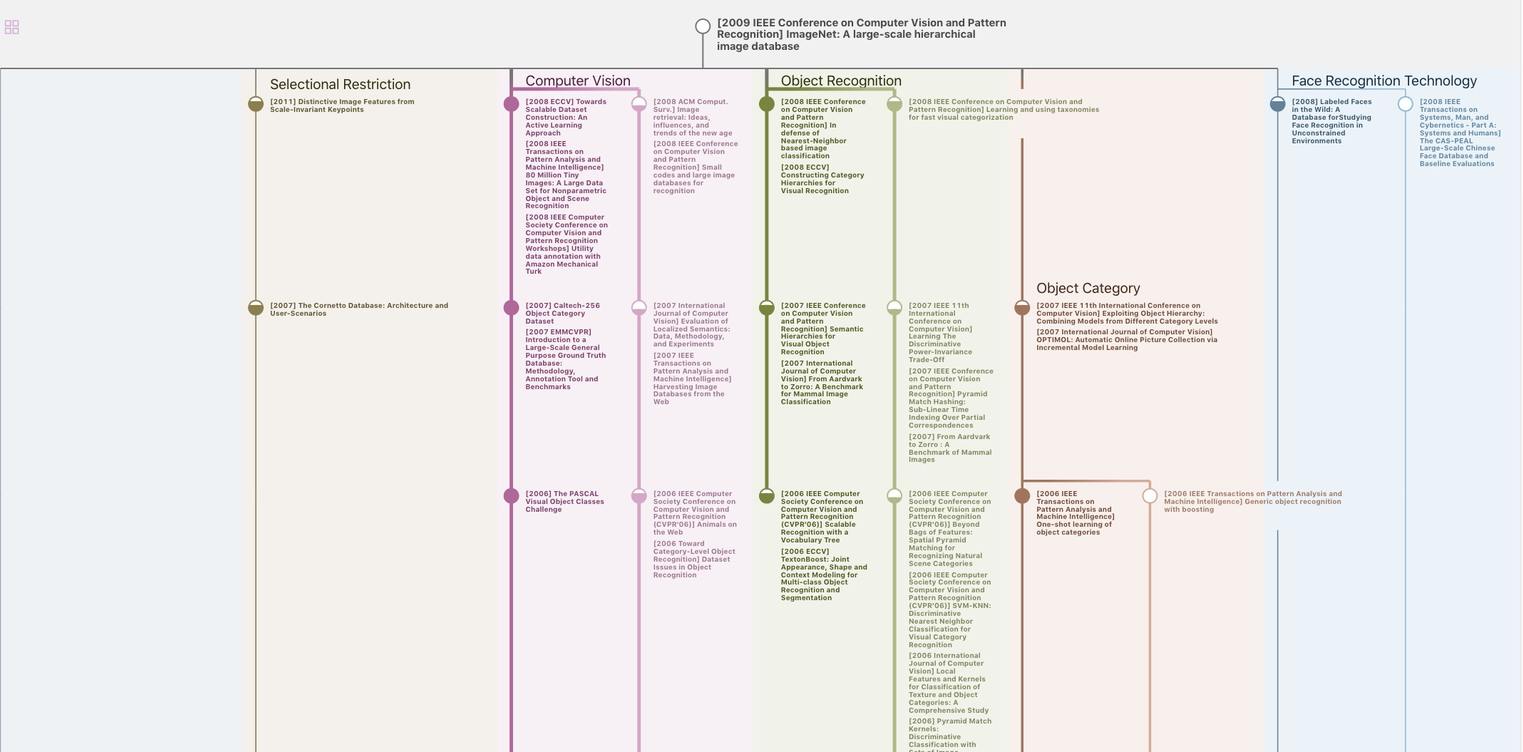
Generate MRT to find the research sequence of this paper
Chat Paper
Summary is being generated by the instructions you defined