Style-Based Global Appearance Flow for Virtual Try-On
IEEE Conference on Computer Vision and Pattern Recognition(2022)
Abstract
Image-based virtual try-on aims to fit an in-shop garment into a clothed person image. To achieve this, a key step is garment warping which spatially aligns the target garment with the corresponding body parts in the person image. Prior methods typically adopt a local appearance flow estimation model. They are thus intrinsically susceptible to difficult body poses/occlusions and large mis-alignments between person and garment images (see Fig. 1). To overcome this limitation, a novel global appearance flow estimation model is proposed in this work. For the first time, a StyleGAN based architecture is adopted for appearance flow estimation. This enables us to take advantage of a global style vector to encode a whole-image context to cope with the aforementioned challenges. To guide the StyleGAN flow generator to pay more attention to local garment deformation, a flow refinement module is introduced to add local context. Experiment results on a popular virtual tryon benchmark show that our method achieves new state-of-the-art performance. It is particularly effective in a ‘in-the-wild’ application scenario where the reference image is full-body resulting in a large mis-alignment with the garment image (Fig. 1 Top). Code is available at: https://github.com/SenHe/Flow-Style-VTON.
MoreTranslated text
Key words
Image and video synthesis and generation, Vision applications and systems
AI Read Science
Must-Reading Tree
Example
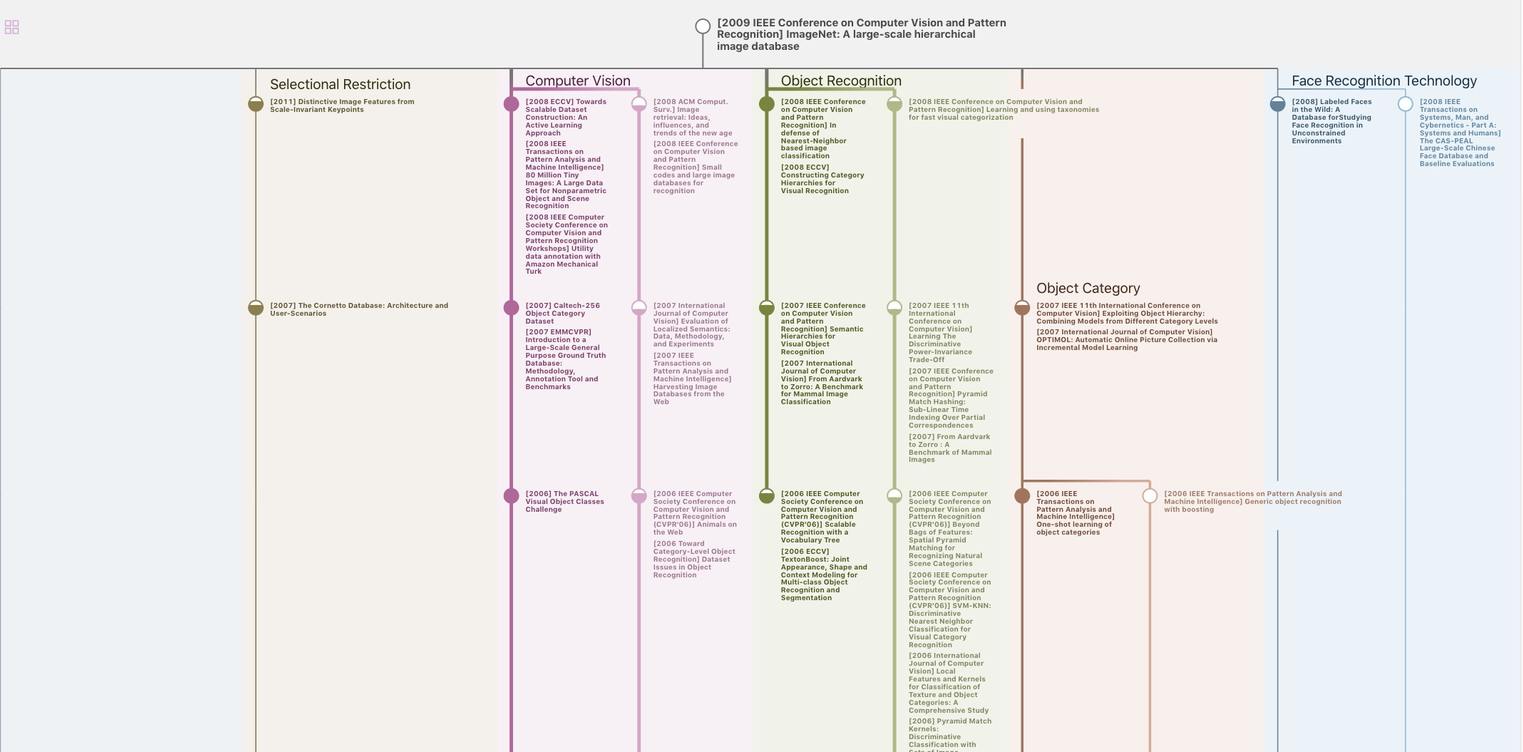
Generate MRT to find the research sequence of this paper
Chat Paper
Summary is being generated by the instructions you defined