Learning to Augment for Casual User Recommendation
International World Wide Web Conference(2022)
摘要
ABSTRACT Users who come to recommendation platforms are heterogeneous in activity levels. There usually exists a group of core users who visit the platform regularly and consume a large body of content upon each visit, while others are casual users who tend to visit the platform occasionally and consume less each time. As a result, consumption activities from core users often dominate the training data used for learning. As core users can exhibit different activity patterns from casual users, recommender systems trained on historical user activity data usually achieve much worse performance on casual users than core users. To bridge the gap, we propose a model-agnostic framework L2Aug to improve recommendations for casual users through data augmentation, without sacrificing core user experience. L2Aug is powered by a data augmentor that learns to generate augmented interaction sequences, in order to fine-tune and optimize the performance of the recommendation system for casual users. On four real-world public datasets, L2Aug outperforms other treatment methods and achieves the best sequential recommendation performance for both casual and core users. We also test L2Aug in an online simulation environment with real-time feedback to further validate its efficacy, and showcase its flexibility in supporting different augmentation actions.
更多查看译文
关键词
Recommendation Systems, Data Augmentation, Policy Learning
AI 理解论文
溯源树
样例
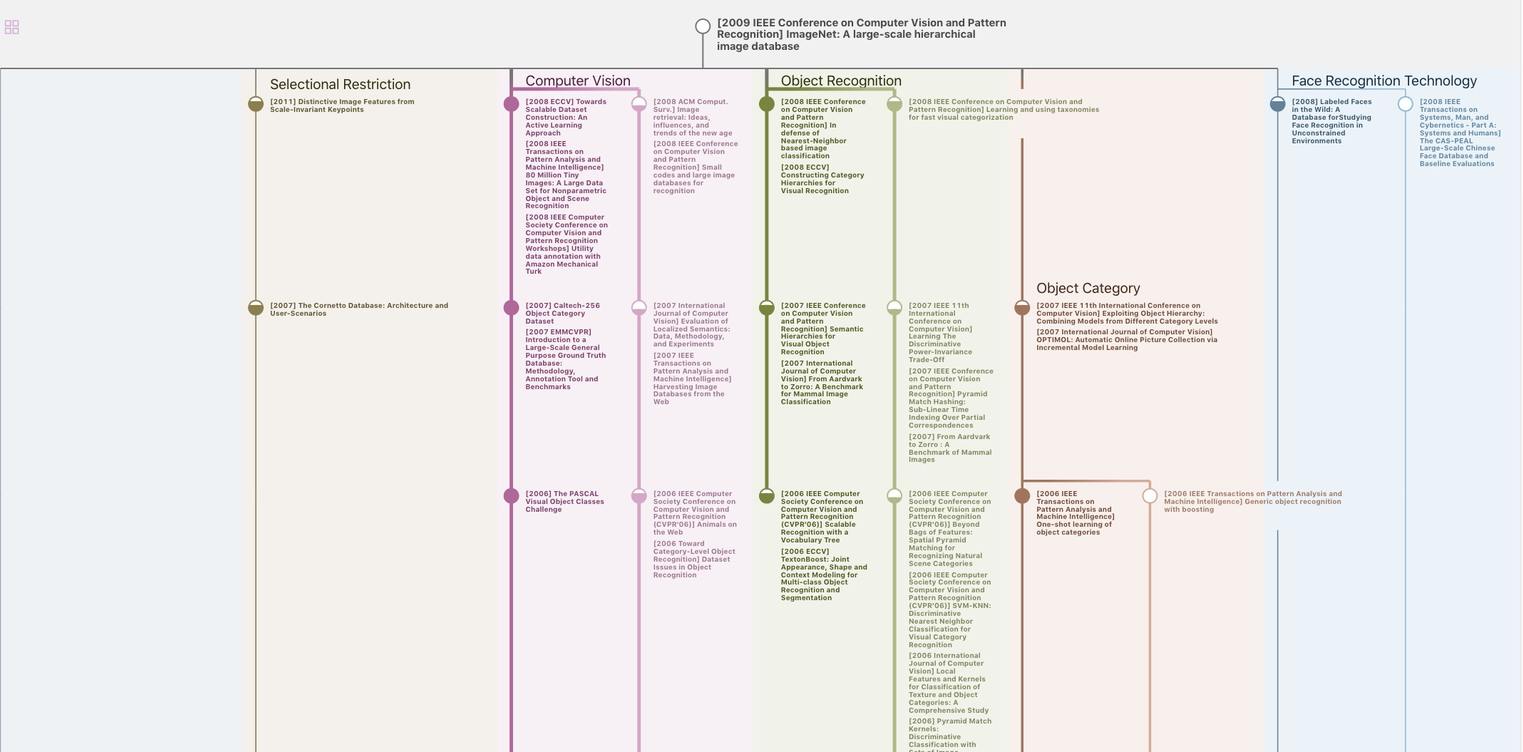
生成溯源树,研究论文发展脉络
Chat Paper
正在生成论文摘要