Provable concept learning for interpretable predictions using variational autoencoders
arxiv(2022)
摘要
In safety-critical applications, practitioners are reluctant to trust neural networks when no interpretable explanations are available. Many attempts to provide such explanations revolve around pixel-based attributions or use previously known concepts. In this paper we aim to provide explanations by provably identifying \emph{high-level, previously unknown ground-truth concepts}. To this end, we propose a probabilistic modeling framework to derive (C)oncept (L)earning and (P)rediction (CLAP) -- a VAE-based classifier that uses visually interpretable concepts as predictors for a simple classifier. Assuming a generative model for the ground-truth concepts, we prove that CLAP is able to identify them while attaining optimal classification accuracy. Our experiments on synthetic datasets verify that CLAP identifies distinct ground-truth concepts on synthetic datasets and yields promising results on the medical Chest X-Ray dataset.
更多查看译文
关键词
interpretable predictions,provable concept,learning
AI 理解论文
溯源树
样例
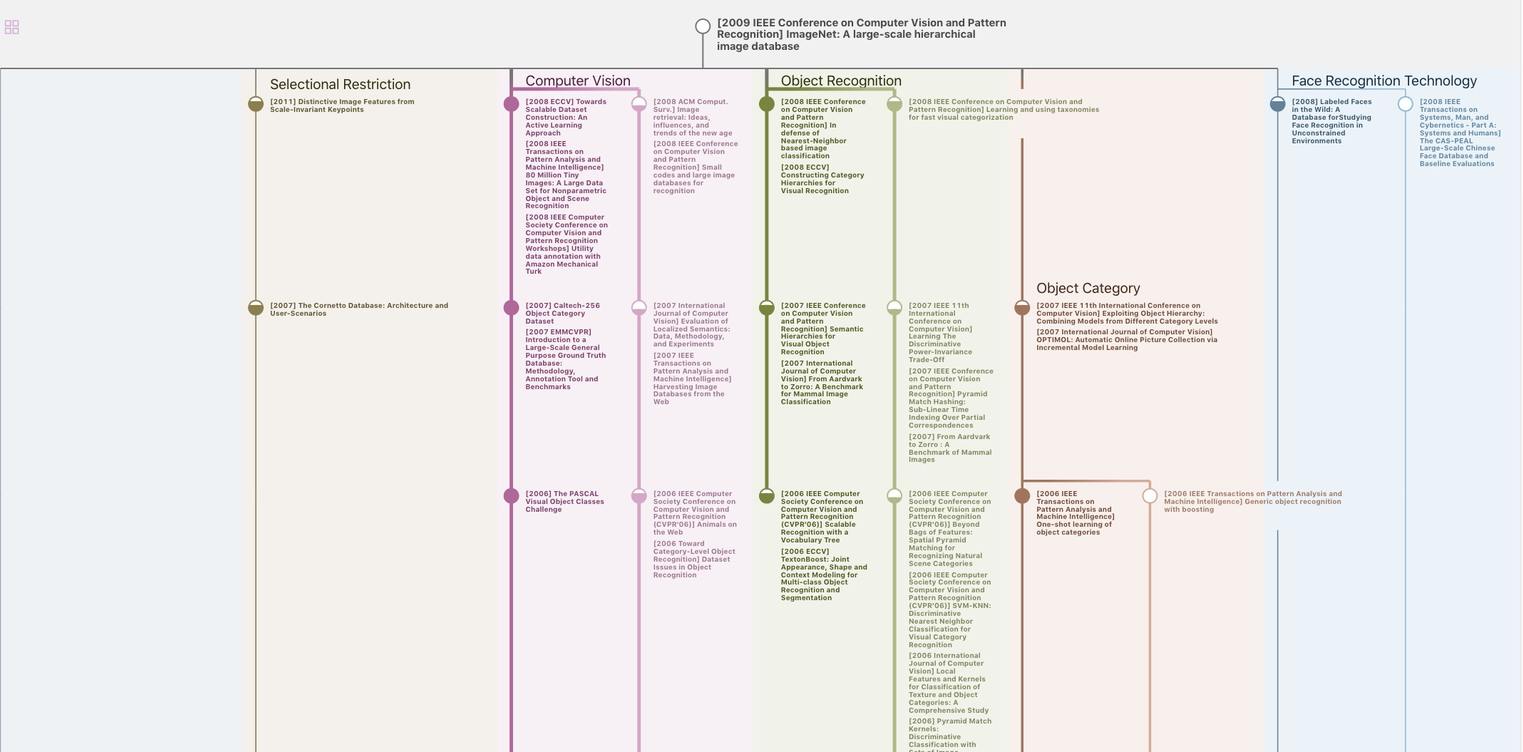
生成溯源树,研究论文发展脉络
Chat Paper
正在生成论文摘要