Fully connected autoencoder and convolutional neural network with attention-based method for inferring disease-related lncRNAs
BRIEFINGS IN BIOINFORMATICS(2022)
摘要
Since abnormal expression of long noncoding RNAs (lncRNAs) is often closely related to various human diseases, identification of disease-associated lncRNAs is helpful for exploring the complex pathogenesis. Most of recent methods concentrate on exploiting multiple kinds of data related to lncRNAs and diseases for predicting candidate disease-related lncRNAs. These methods, however, failed to deeply integrate the topology information from the meta-paths that are composed of lncRNA, disease and microRNA (miRNA) nodes. We proposed a new method based on fully connected autoencoders and convolutional neural networks, called ACLDA, for inferring potential disease-related lncRNA candidates. A heterogeneous graph that consists of lncRNA, disease and miRNA nodes were firstly constructed to integrate similarities, associations and interactions among them. Fully connected autoencoder-based module was established to extract the low-dimensional features of lncRNA, disease and miRNA nodes in the heterogeneous graph. We designed the attention mechanisms at the node feature level and at the meta-path level to learn more informative features and meta-paths. A module based on convolutional neural networks was constructed to encode the local topologies of lncRNA and disease nodes from multiple meta-path perspectives. The comprehensive experimental results demonstrated ACLDA achieves superior performance than several state-of-the-art prediction methods. Case studies on breast, lung and colon cancers demonstrated that ACLDA is able to discover the potential disease-related lncRNAs.
更多查看译文
关键词
topology learning based on meta-paths, meta-path level attention mechanism, node feature level attention mechanism, low-dimensional representation learning, lncRNA-disease association prediction
AI 理解论文
溯源树
样例
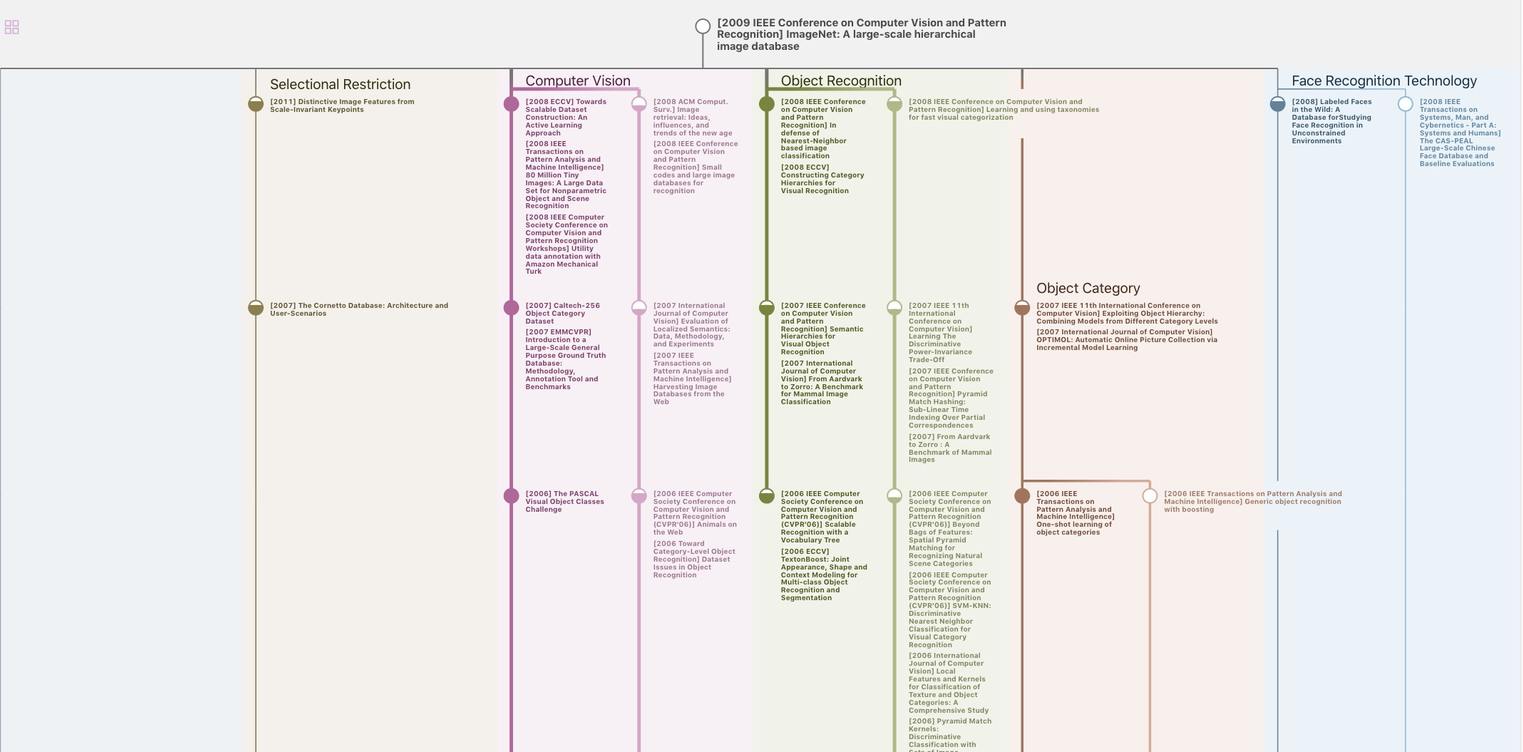
生成溯源树,研究论文发展脉络
Chat Paper
正在生成论文摘要