Model Reduction Through Progressive Latent Space Pruning in Deep Active Inference
FRONTIERS IN NEUROROBOTICS(2022)
摘要
Although still not fully understood, sleep is known to play an important role in learning and in pruning synaptic connections. From the active inference perspective, this can be cast as learning parameters of a generative model and Bayesian model reduction, respectively. In this article, we show how to reduce dimensionality of the latent space of such a generative model, and hence model complexity, in deep active inference during training through a similar process. While deep active inference uses deep neural networks for state space construction, an issue remains in that the dimensionality of the latent space must be specified beforehand. We investigate two methods that are able to prune the latent space of deep active inference models. The first approach functions similar to sleep and performs model reduction post hoc. The second approach is a novel method which is more similar to reflection, operates during training and displays "aha" moments when the model is able to reduce latent space dimensionality. We show for two well-known simulated environments that model performance is retained in the first approach and only diminishes slightly in the second approach. We also show that reconstructions from a real world example are indistinguishable before and after reduction. We conclude that the most important difference constitutes a trade-off between training time and model performance in terms of accuracy and the ability to generalize, via minimization of model complexity.
更多查看译文
关键词
active inference, free energy, deep learning, model reduction, generative modeling
AI 理解论文
溯源树
样例
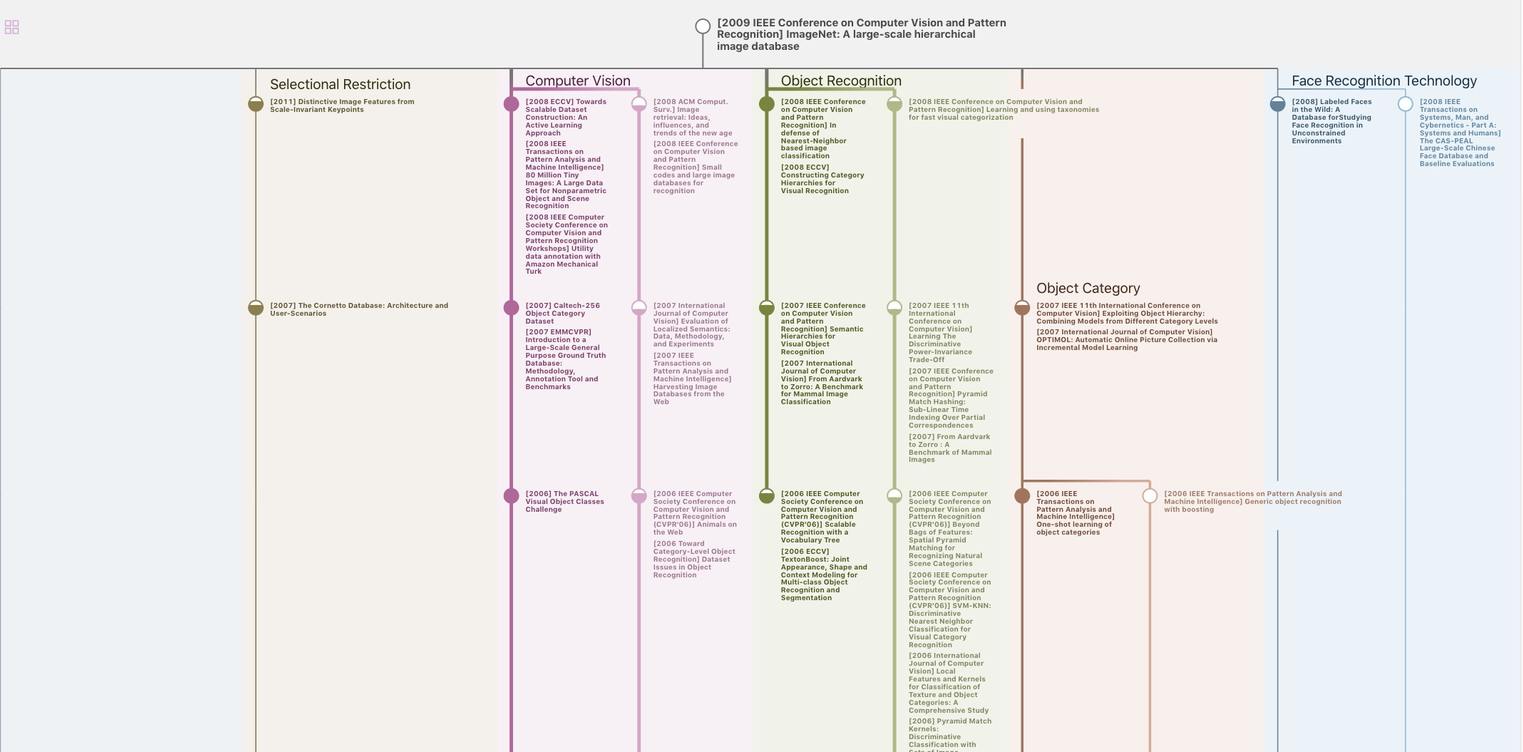
生成溯源树,研究论文发展脉络
Chat Paper
正在生成论文摘要