Data-Driven Forgetting and Discount Factors for Vehicle Speed Forecasting in Ecological Adaptive Cruise Control
JOURNAL OF DYNAMIC SYSTEMS MEASUREMENT AND CONTROL-TRANSACTIONS OF THE ASME(2022)
摘要
This paper investigates temporal correlations in human driving behavior using real-world driving to improve speed forecasting accuracy. These correlations can point to a measurement weighting function with two parameters: a forgetting factor for past speed measurements that the vehicle itself drove with, and a discount factor for the speeds of vehicles ahead based on information from vehicle-to-vehicle communication. The developed weighting approach is applied to a vehicle speed predictor using polynomial regression, a prediction method well-known in the literature. The performance of the developed approach is then assessed in both real-world and simulated traffic scenarios for accuracy and robustness. The new weighting method is applied to an ecological adaptive cruise control system, and its influence is analyzed on the prediction accuracy and the performance of the ecological adaptive cruise control in an electric vehicle powertrain model. The results show that the new prediction method improves energy saving from the eco-driving by up to 4.7% compared to a baseline least-square-based polynomial regression. This is a 10% improvement over the constant speed/acceleration model, a conventional speed predictor.
更多查看译文
关键词
Eco-Driving,Cooperative Adaptive Cruise Control,Vehicle Dynamics Model,Reinforcement Learning,Travel Behavior
AI 理解论文
溯源树
样例
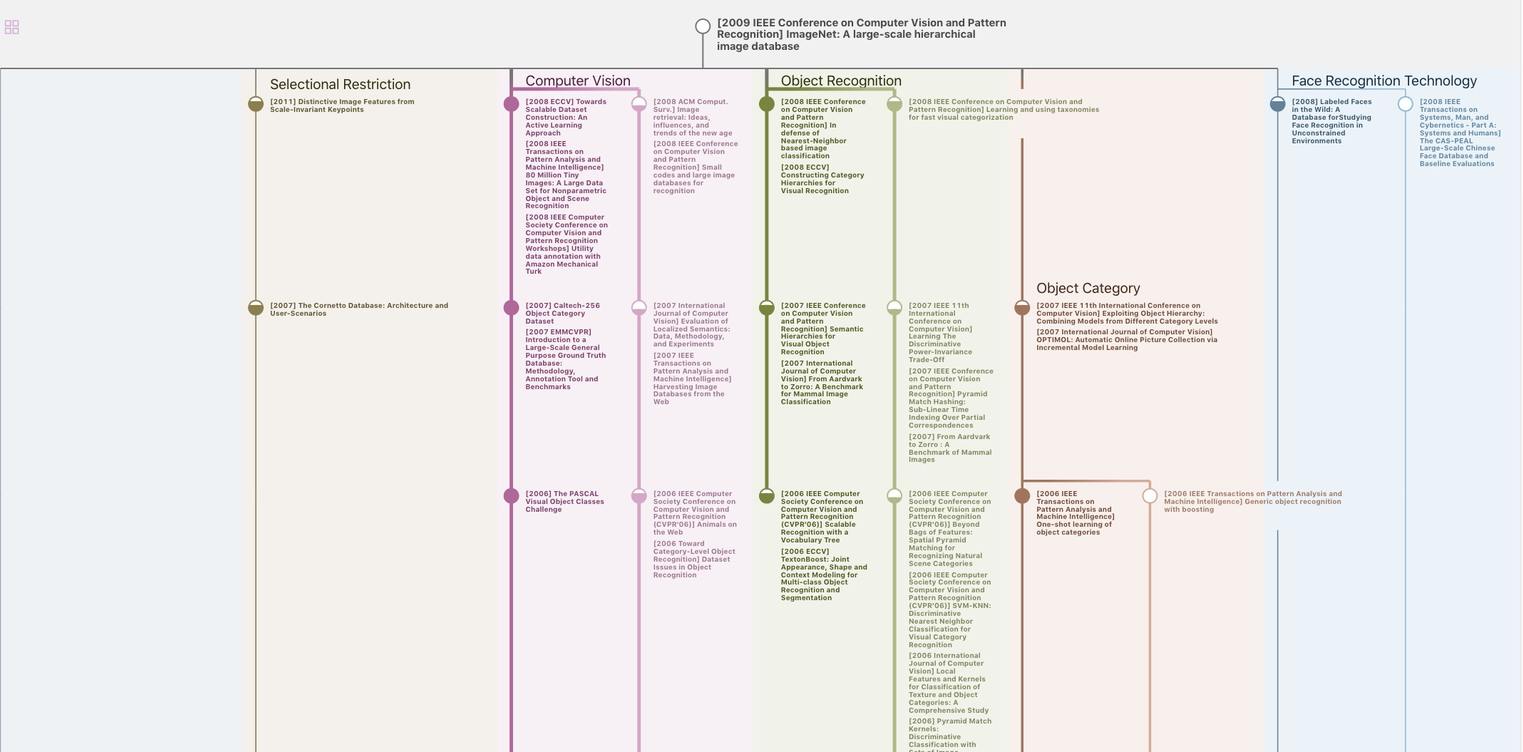
生成溯源树,研究论文发展脉络
Chat Paper
正在生成论文摘要