SimVQA: Exploring Simulated Environments for Visual Question Answering
Proceedings of the IEEE/CVF Conference on Computer Vision and Pattern Recognition (CVPR)(2022)
摘要
Existing work on VQA explores data augmentation to achieve better generalization by perturbing the images in the dataset or modifying the existing questions and answers. While these methods exhibit good performance, the diversity of the questions and answers are constrained by the available image set. In this work we explore using synthetic computer-generated data to fully control the visual and language space, allowing us to provide more diverse scenarios. We quantify the effect of synthetic data in real-world VQA benchmarks and to which extent it produces results that generalize to real data. By exploiting 3D and physics simulation platforms, we provide a pipeline to generate synthetic data to expand and replace type-specific questions and answers without risking the exposure of sensitive or personal data that might be present in real images. We offer a comprehensive analysis while expanding existing hyper-realistic datasets to be used for VQA. We also propose Feature Swapping (F-SWAP) -- where we randomly switch object-level features during training to make a VQA model more domain invariant. We show that F-SWAP is effective for enhancing a currently existing VQA dataset of real images without compromising on the accuracy to answer existing questions in the dataset.
更多查看译文
关键词
visual question answering,simulated environments
AI 理解论文
溯源树
样例
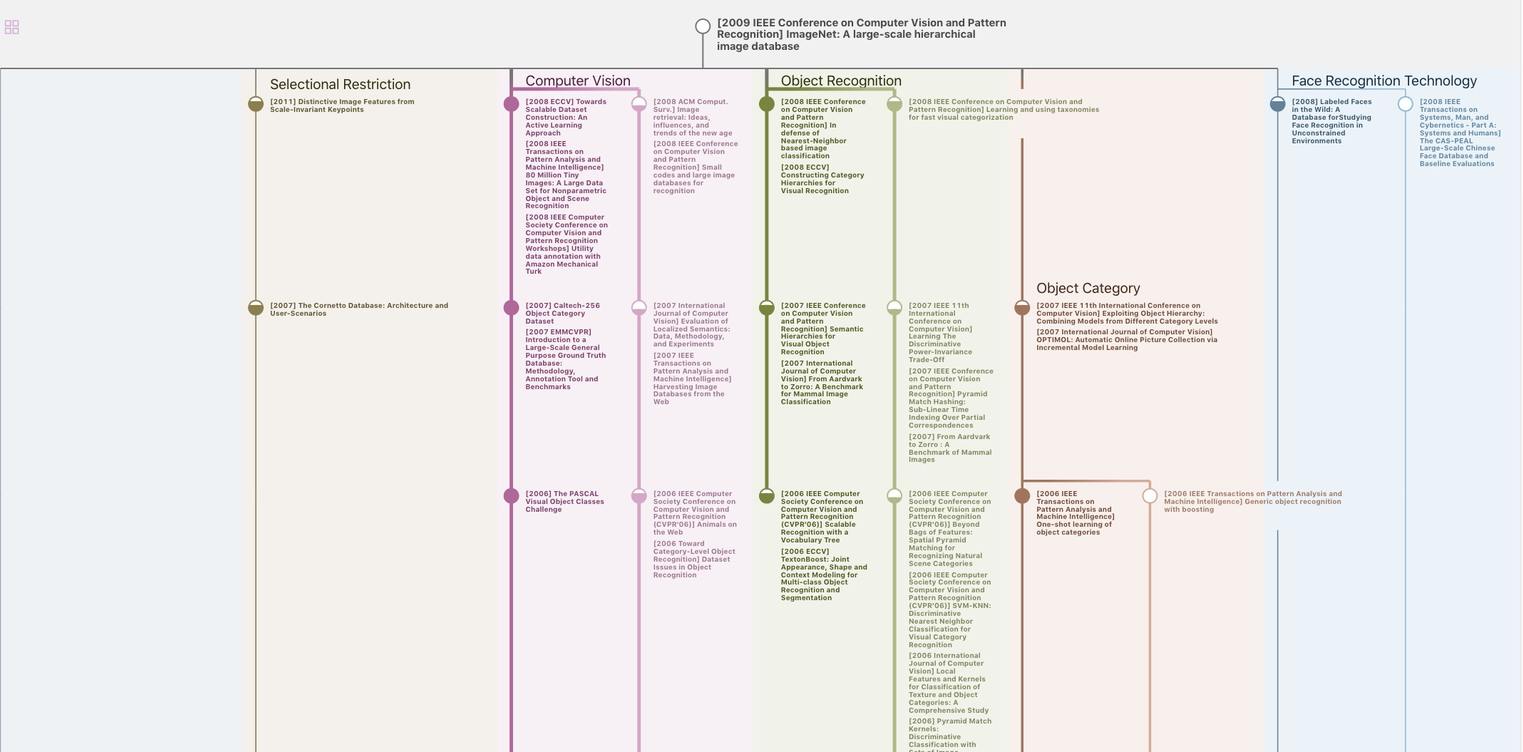
生成溯源树,研究论文发展脉络
Chat Paper
正在生成论文摘要