Logit Normalization for Long-Tail Object Detection
International Journal of Computer Vision(2024)
Abstract
Real-world data with skewed distributions poses a serious challenge to existing object detectors. The unbalanced label distribution leads to a bias towards dominate labels, resulting in the worse detection performance on the rare classes than the dominant classes. More unfortunately, the label samplers in these detectors shift the training label distributions to a new skewed distribution, thereby severely limiting the effectiveness of previous prior-based methods such as Logit Adjustment (Menon et al., in ICLR. OpenReview.net, 2021). Additionally, the tremendous ratio of the background samples to the samples per foreground category further hinders the learning of classification on foreground categories. To mitigate these issues, in this paper, we propose Logit Normalization (LogN), a simple technique to self-calibrate the classification logits of detectors in a similar way to Batch Normalization (BN). LogN first leverages the consistency between logit statistics and the training label distribution to eliminate the long-tail bias of detectors in a normalized manner. Second, based on the independence between fore-background imbalance and long-tail distribution, we also introduce a background calibration for LogN, which effectively improves the overall performance by restoring the background discriminability. In general, our LogN is training- and tuning-free ( i.e. require no extra training and tuning process), model- and label distribution-agnostic ( i.e. generalization to different kinds of detectors and datasets), and also plug-and-play ( i.e. direct application without any bells and whistles). Extensive experiments on the LVIS dataset demonstrate the superior performance of LogN to the state-of-the-art methods with various detectors ( e.g. two-stage detectors, one-stage detectors, query-based detectors) and backbones ( e.g. VITs, Swin Transformers). We also provide in-depth studies on different aspects of our LogN. We also conduct experiments on multiple datasets such as Open Images and ImageNet-LT. The results show that LogN can improve performance on other object detection datasets and the image classification task. Our LogN can serve as a strong baseline for long-tail object detection and is expected to inspire future research in this field.
MoreTranslated text
Key words
Normalization,Logit calibration,Long-tail learning,Object detection
AI Read Science
Must-Reading Tree
Example
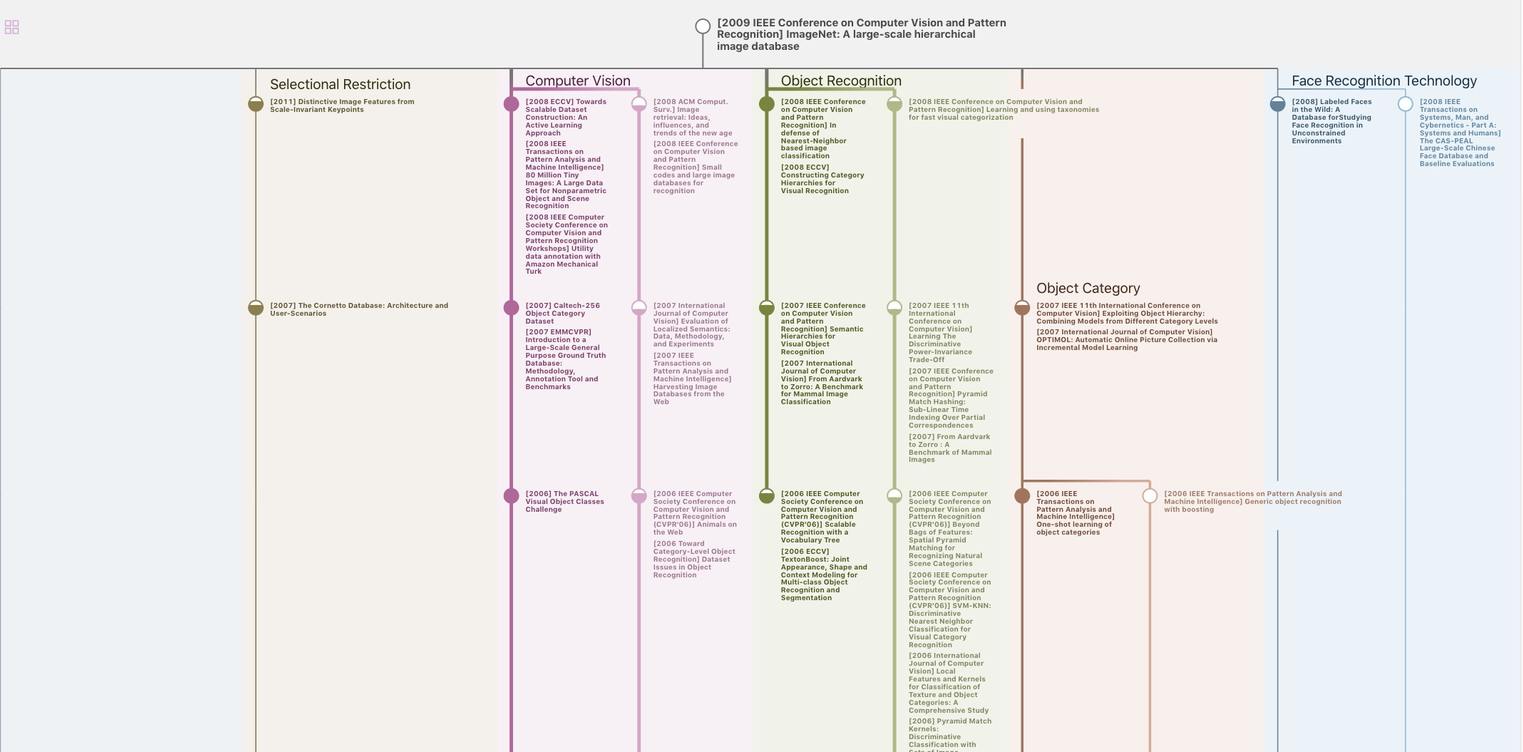
Generate MRT to find the research sequence of this paper
Chat Paper
Summary is being generated by the instructions you defined