Quasi-orthogonality and intrinsic dimensions as measures of learning and generalisation.
IEEE International Joint Conference on Neural Network (IJCNN)(2022)
Abstract
Finding best architectures of learning machines, such as deep neural networks, is a well-known technical and theoretical challenge. Recent work by Mellor et al (2021) showed that there may exist correlations between the accuracies of trained networks and the values of some easily computable measures defined on randomly initialised networks which may enable to search tens of thousands of neural architectures without training. Mellor et al used the Hamming distance evaluated over all ReLU neurons as such a measure. Motivated by these findings, in our work, we ask the question of the existence of other and perhaps more principled measures which could be used as determinants of success of a given neural architecture. In particular, we examine, if the dimensionality and quasi-orthogonality of neural networks' feature space could be correlated with the network's performance after training. We showed, using the setup as in Mellor et al, that dimensionality and quasi-orthogonality may jointly serve as network's performance discriminants. In addition to offering new opportunities to accelerate neural architecture search, our findings suggest important relationships between the networks' final performance and properties of their randomly initialised feature spaces: data dimension and quasi-orthogonality.
MoreTranslated text
Key words
deep learning,neural architectural search,orthogonality,dimensionality
AI Read Science
Must-Reading Tree
Example
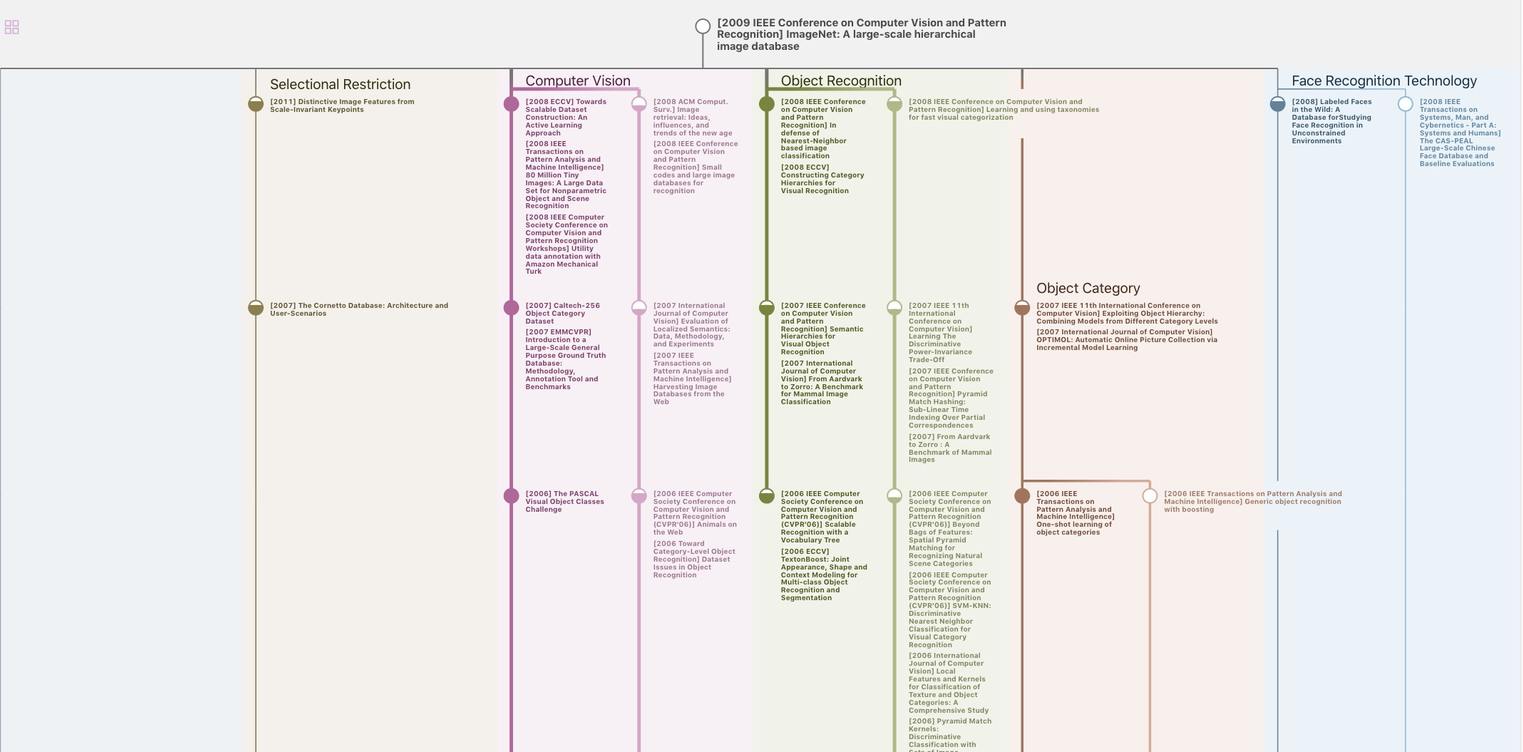
Generate MRT to find the research sequence of this paper
Chat Paper
Summary is being generated by the instructions you defined