Exploring the hysteresis properties of nanocrystalline permanent magnets using deep learning
arxiv(2022)
摘要
We demonstrate the use of model order reduction and neural networks for estimating the hysteresis properties of nanocrystalline permanent magnets from microstructure. With a data-driven approach, we learn the demagnetization curve from data-sets created by grain growth and micromagnetic simulations. We show that the granular structure of a magnet can be encoded within a low-dimensional latent space. Latent codes are constructed using a variational autoencoder. The mapping of structure code to hysteresis properties is a multi-target regression problem. We apply deep neural network and use parameter sharing, in order to predict anchor points along the demagnetization curves from the magnet's structure code. The method is applied to study the magnetic properties of nanocrystalline permanent magnets. We show how new grain structures can be generated by interpolation between two points in the latent space and how the magnetic properties of the resulting magnets can be predicted.
更多查看译文
关键词
nanocrystalline permanent magnets,hysteresis properties,deep learning
AI 理解论文
溯源树
样例
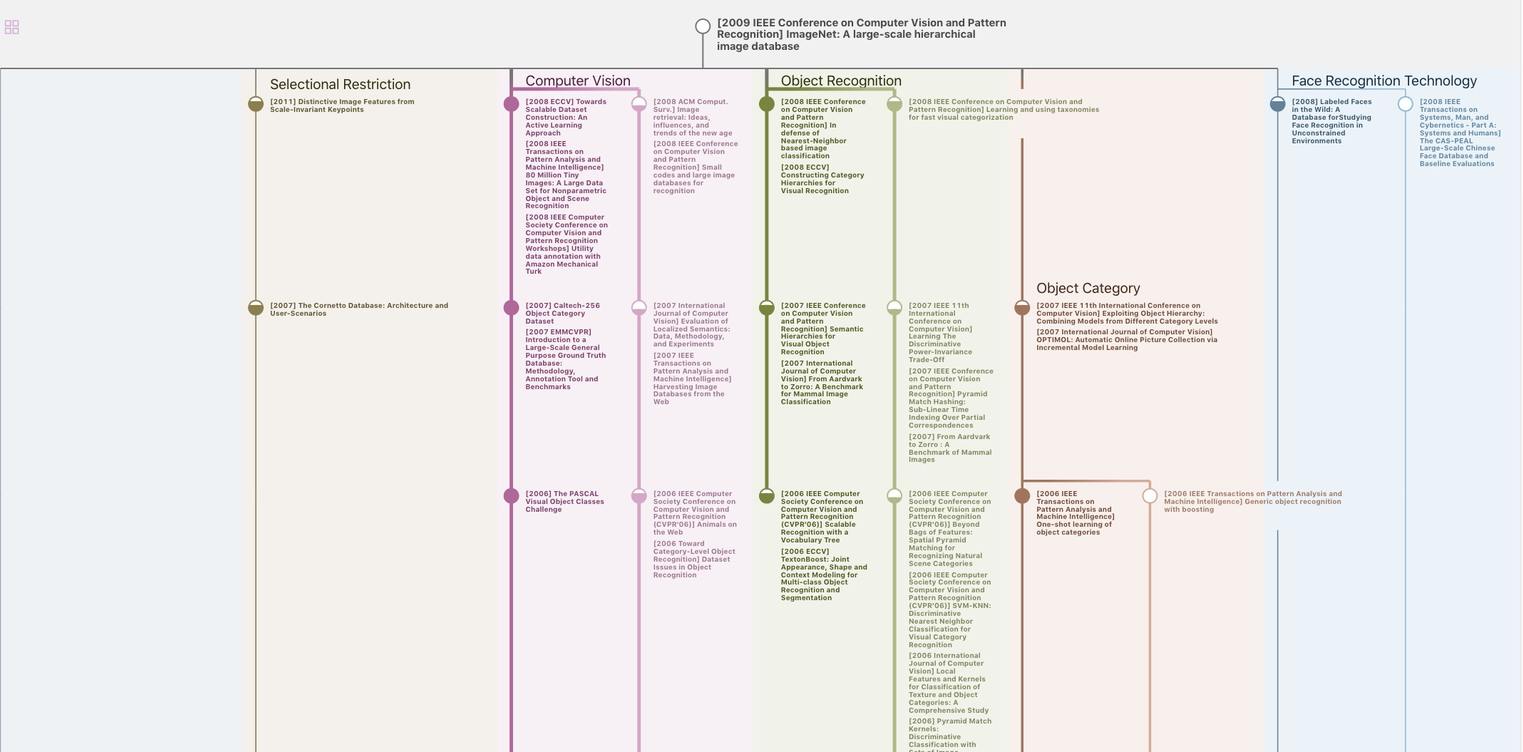
生成溯源树,研究论文发展脉络
Chat Paper
正在生成论文摘要