IGRF-RFE: a hybrid feature selection method for MLP-based network intrusion detection on UNSW-NB15 dataset
J. Big Data(2023)
Abstract
The effectiveness of machine learning models can be significantly averse to redundant and irrelevant features present in the large dataset which can cause drastic performance degradation. This paper proposes IGRF-RFE: a hybrid feature selection method tasked for multi-class network anomalies using a multilayer perceptron (MLP) network. IGRF-RFE exploits the qualities of both a filter method for its speed and a wrapper method for its relevance search. In the first phase of our approach, we use a combination of two filter methods, information gain (IG) and random forest (RF) respectively, to reduce the feature subset search space. By combining these two filter methods, the influence of less important features but with the high-frequency values selected by IG is more effectively managed by RF resulting in more relevant features to be included in the feature subset search space. In the second phase of our approach, we use a machine learning-based wrapper method that provides a recursive feature elimination (RFE) to further reduce feature dimensions while taking into account the relevance of similar features. Our experimental results obtained based on the UNSW-NB15 dataset confirmed that our proposed method can improve the accuracy of anomaly detection as it can select more relevant features while reducing the feature space. The results show that the feature is reduced from 42 to 23 while the multi-classification accuracy of MLP is improved from 82.25% to 84.24%.
MoreTranslated text
Key words
network intrusion detection,hybrid feature selection method,feature selection,igrf-rfe,mlp-based
AI Read Science
Must-Reading Tree
Example
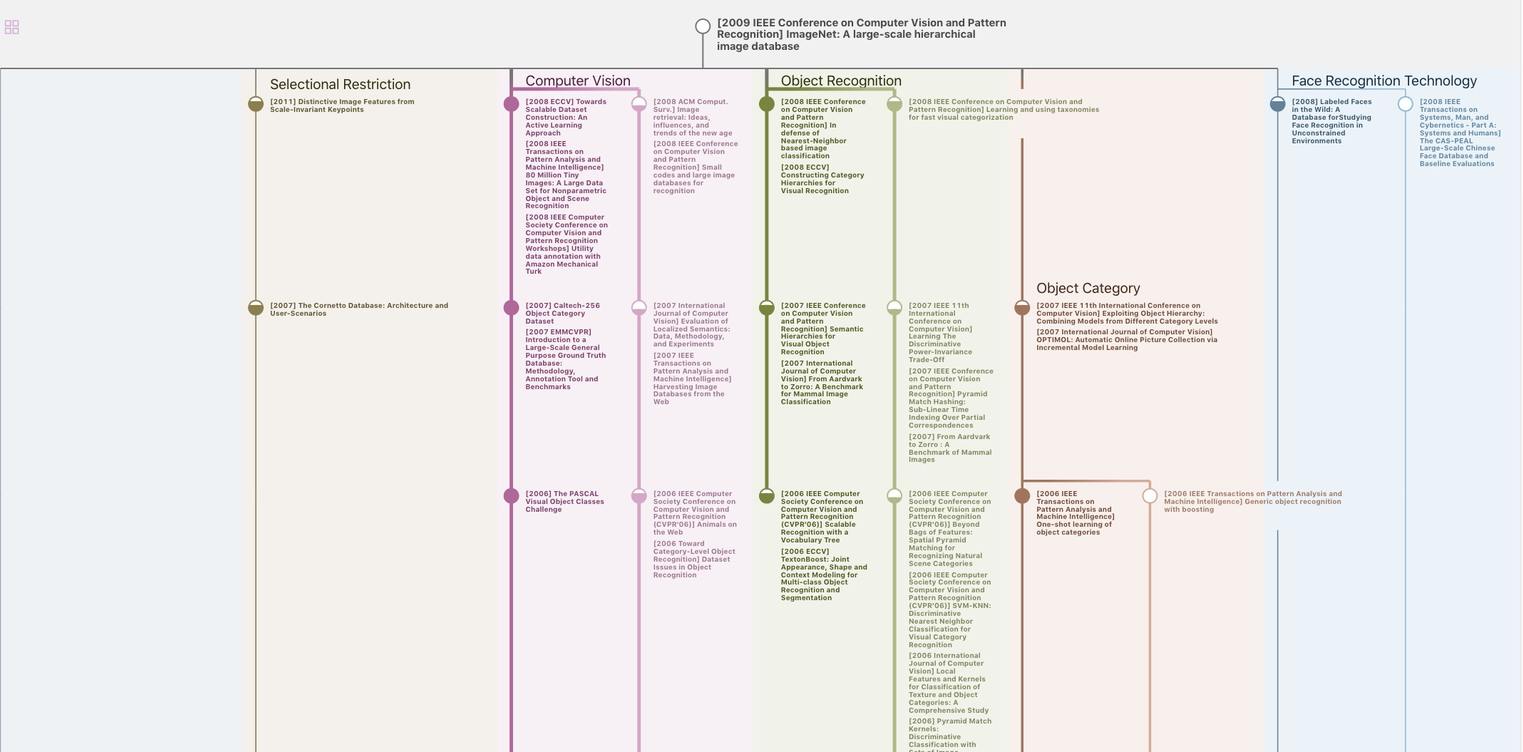
Generate MRT to find the research sequence of this paper
Chat Paper
Summary is being generated by the instructions you defined