Streaming parallel transducer beam search with fast slow cascaded encoders
Conference of the International Speech Communication Association (INTERSPEECH)(2022)
摘要
Streaming ASR with strict latency constraints is required in many speech recognition applications. In order to achieve the required latency, streaming ASR models sacrifice accuracy compared to non-streaming ASR models due to lack of future input context. Previous research has shown that streaming and non-streaming ASR for RNN Transducers can be unified by cascading causal and non-causal encoders. This work improves upon this cascaded encoders framework by leveraging two streaming non-causal encoders with variable input context sizes that can produce outputs at different audio intervals (e.g. fast and slow). We propose a novel parallel time-synchronous beam search algorithm for transducers that decodes from fast-slow encoders, where the slow encoder corrects the mistakes generated from the fast encoder. The proposed algorithm, achieves up to 20% WER reduction with a slight increase in token emission delays on the public Librispeech dataset and in-house datasets. We also explore techniques to reduce the computation by distributing processing between the fast and slow encoders. Lastly, we explore sharing the parameters in the fast encoder to reduce the memory footprint. This enables low latency processing on edge devices with low computation cost and a low memory footprint.
更多查看译文
关键词
Speech recognition, RNN-T, beam search
AI 理解论文
溯源树
样例
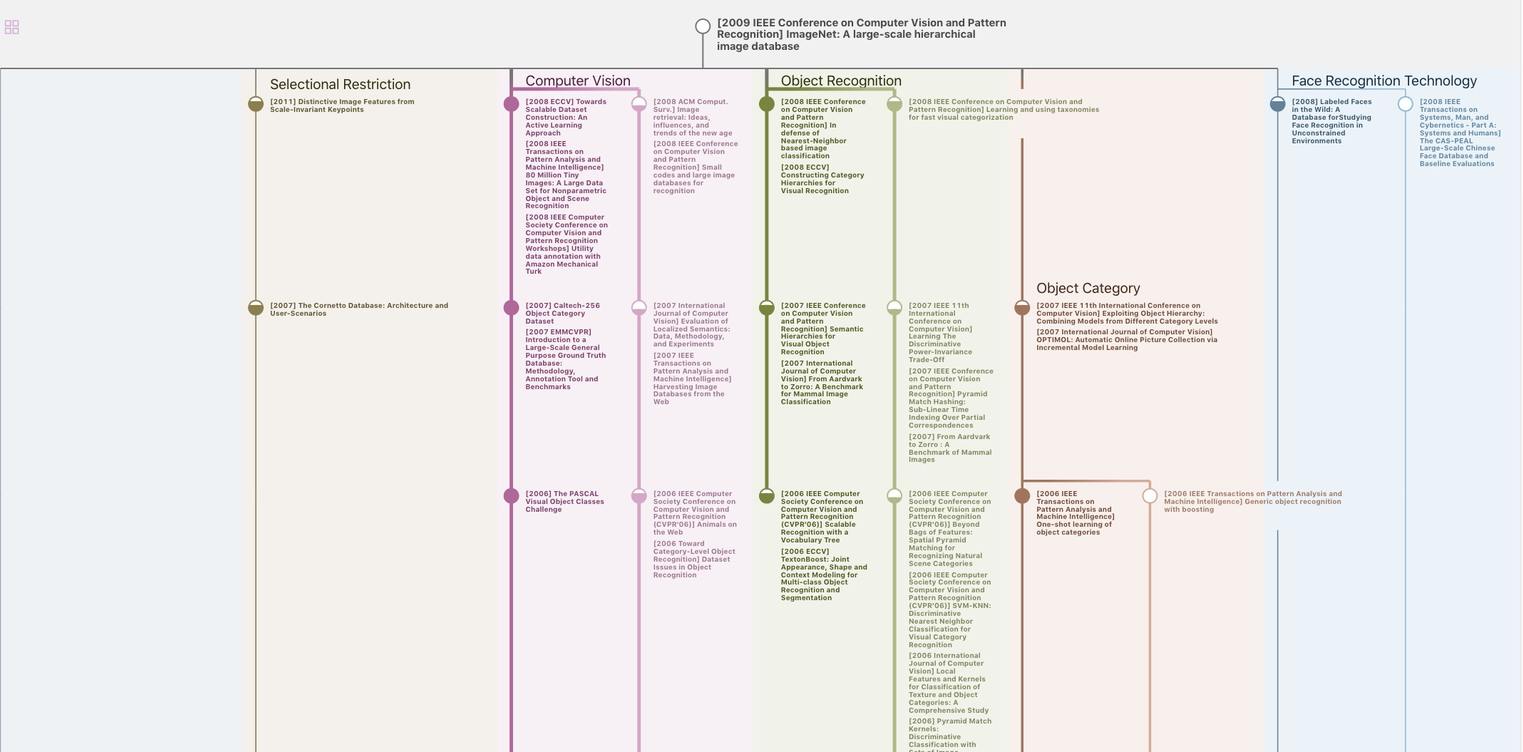
生成溯源树,研究论文发展脉络
Chat Paper
正在生成论文摘要