Transformer Inertial Poser: Real-time Human Motion Reconstruction from Sparse IMUs with Simultaneous Terrain Generation.
SIGGRAPH Asia(2022)
摘要
Real-time human motion reconstruction from a sparse set of (e.g. six) wearable IMUs provides a non-intrusive and economic approach to motion capture. Without the ability to acquire position information directly from IMUs, recent works took data-driven approaches that utilize large human motion datasets to tackle this under-determined problem. Still, challenges remain such as temporal consistency, drifting of global and joint motions, and diverse coverage of motion types on various terrains. We propose a novel method to simultaneously estimate full-body motion and generate plausible visited terrain from only six IMU sensors in real-time. Our method incorporates 1. a conditional Transformer decoder model giving consistent predictions by explicitly reasoning prediction history, 2. a simple yet general learning target named "stationary body points" (SBPs) which can be stably predicted by the Transformer model and utilized by analytical routines to correct joint and global drifting, and 3. an algorithm to generate regularized terrain height maps from noisy SBP predictions which can in turn correct noisy global motion estimation. We evaluate our framework extensively on synthesized and real IMU data, and with real-time live demos, and show superior performance over strong baseline methods.
更多查看译文
关键词
Wearable Devices, Inertial Measurement Units, Human Motion
AI 理解论文
溯源树
样例
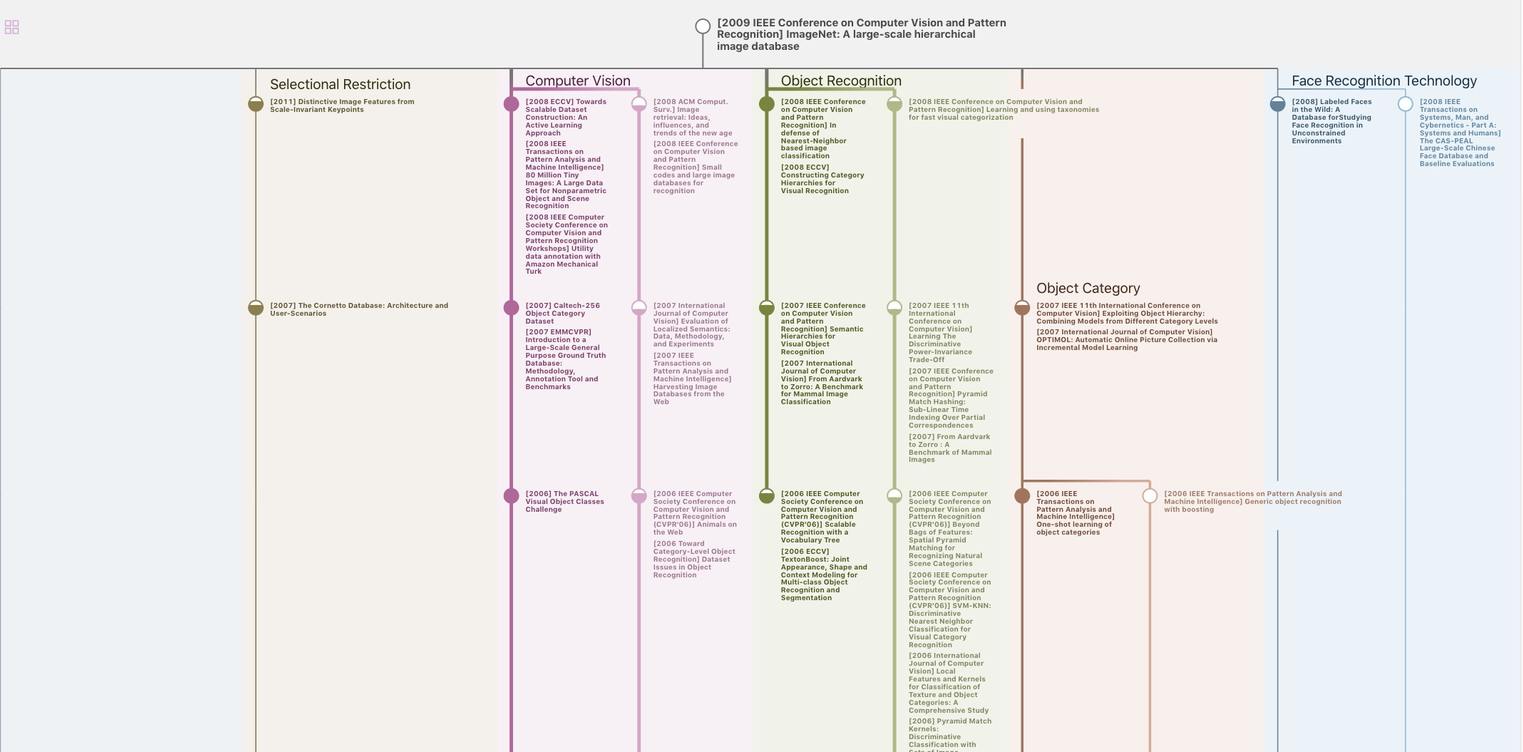
生成溯源树,研究论文发展脉络
Chat Paper
正在生成论文摘要