Classification of hyperspectral images using svm with shape-adaptive reconstruction and smoothed total variation
IEEE International Geoscience and Remote Sensing Symposium (IGARSS)(2022)
摘要
In this work, a novel algorithm called SVM with Shape-adaptive Reconstruction and Smoothed Total Variation (SaR-SVM-STV) is introduced to classify hyperspectral images, which makes full use of spatial and spectral information. The Shape-adaptive Reconstruction (SaR) is introduced to preprocess each pixel based on the Pearson Correlation between pixels in its shape-adaptive (SA) region. Support Vector Machines (SVMs) are trained to estimate the pixel-wise probability maps of each class. Then the Smoothed Total Variation (STV) model is applied to denoise and generate the final classification map. Experiments show that SaR-SVM-STV outperforms the SVM-STV method with a few training labels, demonstrating the significance of reconstructing hyperspectral images before classification.
更多查看译文
关键词
Hyperspectral Image Classification,Image Reconstruction,Support Vector Machines,Smoothed Total Variation,Semi-supervised Learning
AI 理解论文
溯源树
样例
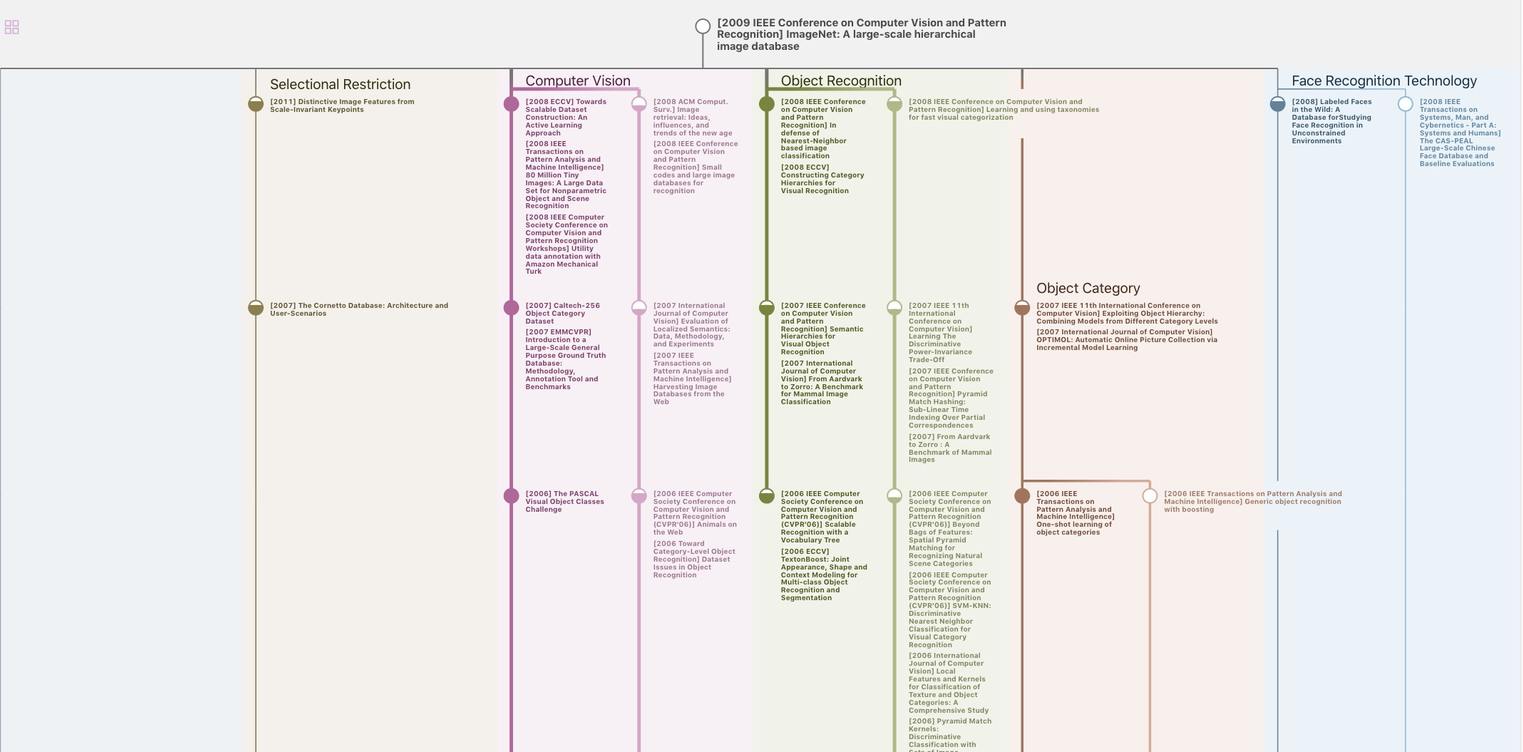
生成溯源树,研究论文发展脉络
Chat Paper
正在生成论文摘要