MR-GMMapping: Communication Efficient Multi-Robot Mapping System via Gaussian Mixture Model
IEEE ROBOTICS AND AUTOMATION LETTERS(2022)
摘要
Collaborative perception in unknown environments is a critical task for multi-robot systems. Without external positioning, multi-robot mapping systems have relied on the transfer of place recognition (PR) descriptors or sensor data for the relative pose estimation (RelPose) and share their local maps for relative localization. Thus, in a communication limited environment, data transmission can become a significant communication bottleneck in the multi-robot mapping system. To address this limitation, we propose MR-GMMapping, a Multi-Robot GMM-based mapping system in which robots perform relative localization only with GMM submaps to reduce data transmission and storage. For mapping, we propose GMM submap construction strategy with an adaptive model selection method, which makes robots dynamically select the appropriate number of Gaussian components. For applications, we realize fully GMM-submap-based PR, RelPose, and local planner. Robots are able to perform relative localization without the aid of other forms of maps or information, which makes them favorable for environments with communication constraints. Experiments show that our GMM Submap extraction strategy improves 11% translation precision and 30% rotation precision in RelPose, compared to RelPose on point clouds and GMM frames. Our experiments also show the feasibility of the GMM-based local planner and a 98% data transmission reduction compared to point cloud maps. MR-GMMapping is published as an open source ROS project at https://github.com/efc-robot/gmm_map_ python.git.
更多查看译文
关键词
Multi-robot SLAM, mapping
AI 理解论文
溯源树
样例
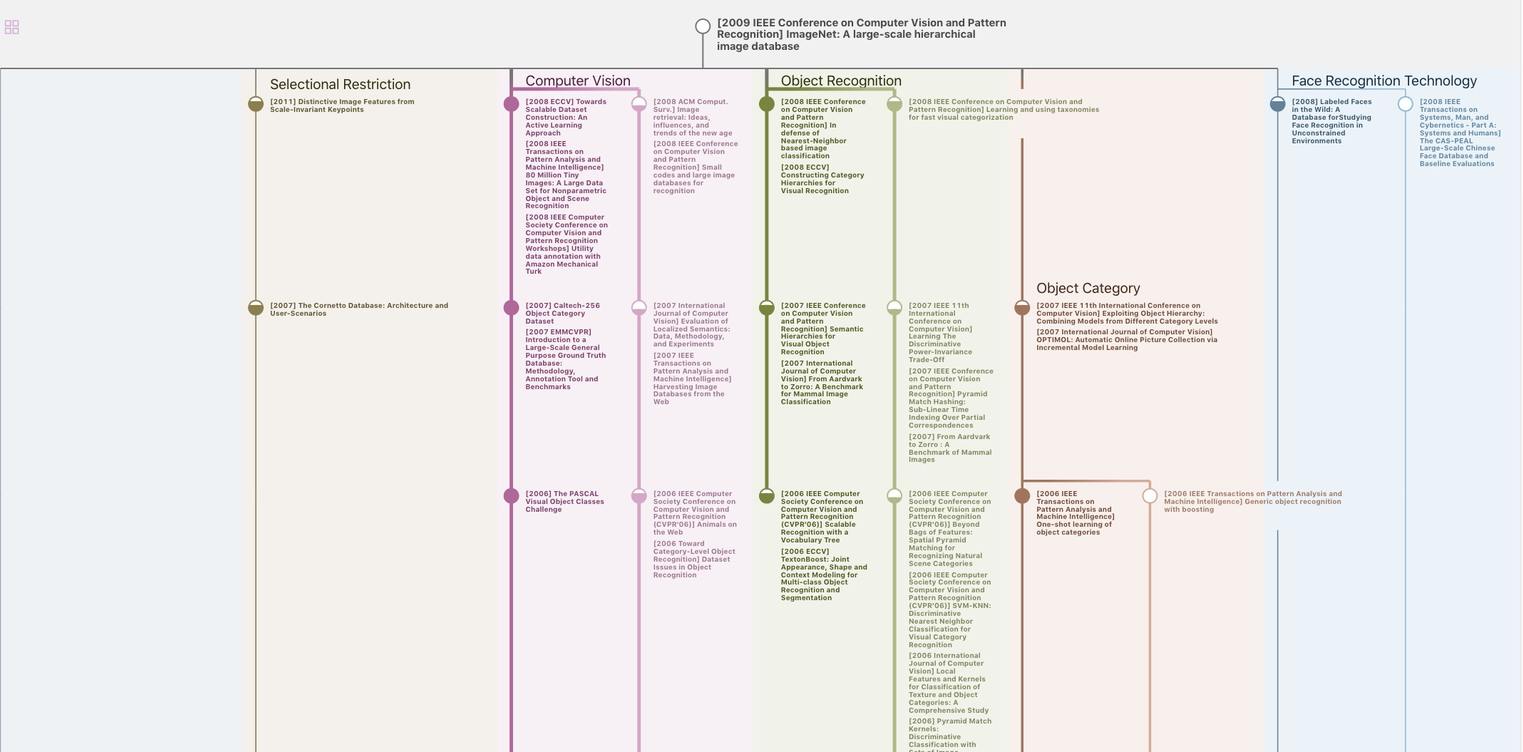
生成溯源树,研究论文发展脉络
Chat Paper
正在生成论文摘要