Fast-Converging Simulated Annealing for Ising Models Based on Integral Stochastic Computing
IEEE transactions on neural networks and learning systems(2023)
摘要
Probabilistic bits (p-bits) have recently been presented as a spin (basic computing element) for the simulated annealing (SA) of Ising models. In this brief, we introduce fast-converging SA based on p-bits designed using integral stochastic computing. The stochastic implementation approximates a p-bit function, which can search for a solution to a combinatorial optimization problem at lower energy than conventional p-bits. Searching around the global minimum energy can increase the probability of finding a solution. The proposed stochastic computing-based SA method is compared with conventional SA and quantum annealing (QA) with a D-Wave Two quantum annealer on the traveling salesman, maximum cut (MAX-CUT), and graph isomorphism (GI) problems. The proposed method achieves a convergence speed a few orders of magnitude faster while dealing with an order of magnitude larger number of spins than the other methods.
更多查看译文
关键词
Stochastic processes,Computational modeling,Optimization,Urban areas,Probabilistic logic,Integrated circuit modeling,Convergence,Combinatorial optimization,graph isomorphism (GI) problem,Hamiltonian,Ising model,quantum annealing (QA),simulated annealing (SA),stochastic computing,traveling salesman problem (TSP)
AI 理解论文
溯源树
样例
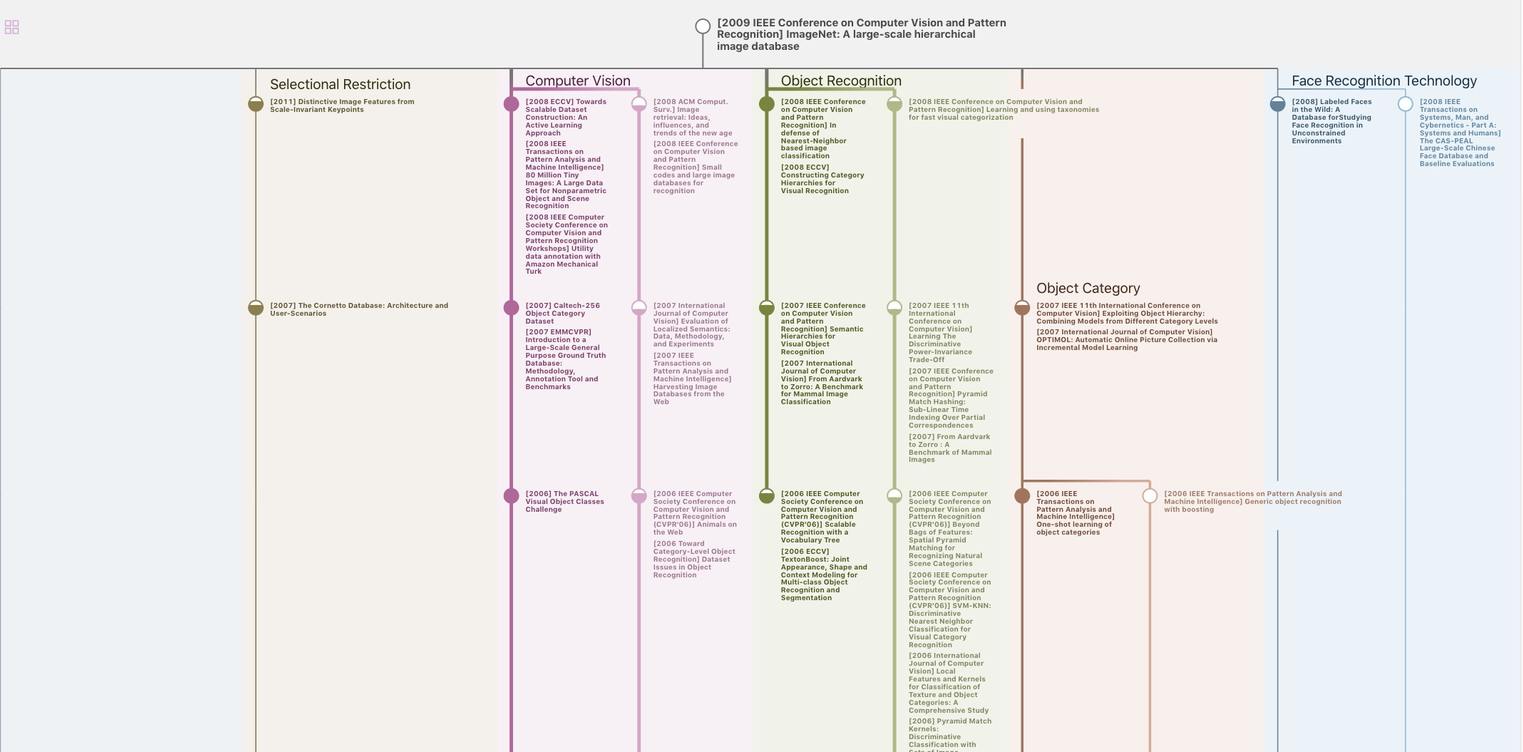
生成溯源树,研究论文发展脉络
Chat Paper
正在生成论文摘要