Non-Technical Loss Detection Using Deep Reinforcement Learning for Feature Cost Efficiency and Imbalanced Dataset
IEEE ACCESS(2022)
摘要
One of the problems of the electricity grid system is electricity loss due to energy theft, which is known as non-technical loss (NTL). The sustainability and stability of the grid system are threatened by the unexpected electricity losses. Energy theft detection based on data analysis is one of the solutions to alleviate the drawbacks of NTL. The main problem of data-based NTL detection is that collected electricity usage dataset is imbalanced. In this paper, we approach the NTL detection problem using deep reinforcement learning (DRL) to solve the data imbalanced problem of NTL. The advantage of the proposed method is that the classification method is adopted to use the partial input features without pre-processing method for input feature selection. Moreover, extra pre-processing steps to balance the dataset are unnecessary to detect NTL compared to the conventional NTL detection algorithms. From the simulation results, the proposed method provides better performances compared to the conventional algorithms under various simulation environments.
更多查看译文
关键词
Neural networks, Mathematical models, Feature extraction, Data models, Classification algorithms, Power system stability, Licenses, Deep reinforcement learning, non-technical loss (NTL), energy theft, feature cost efficiency, data imbalanced problem
AI 理解论文
溯源树
样例
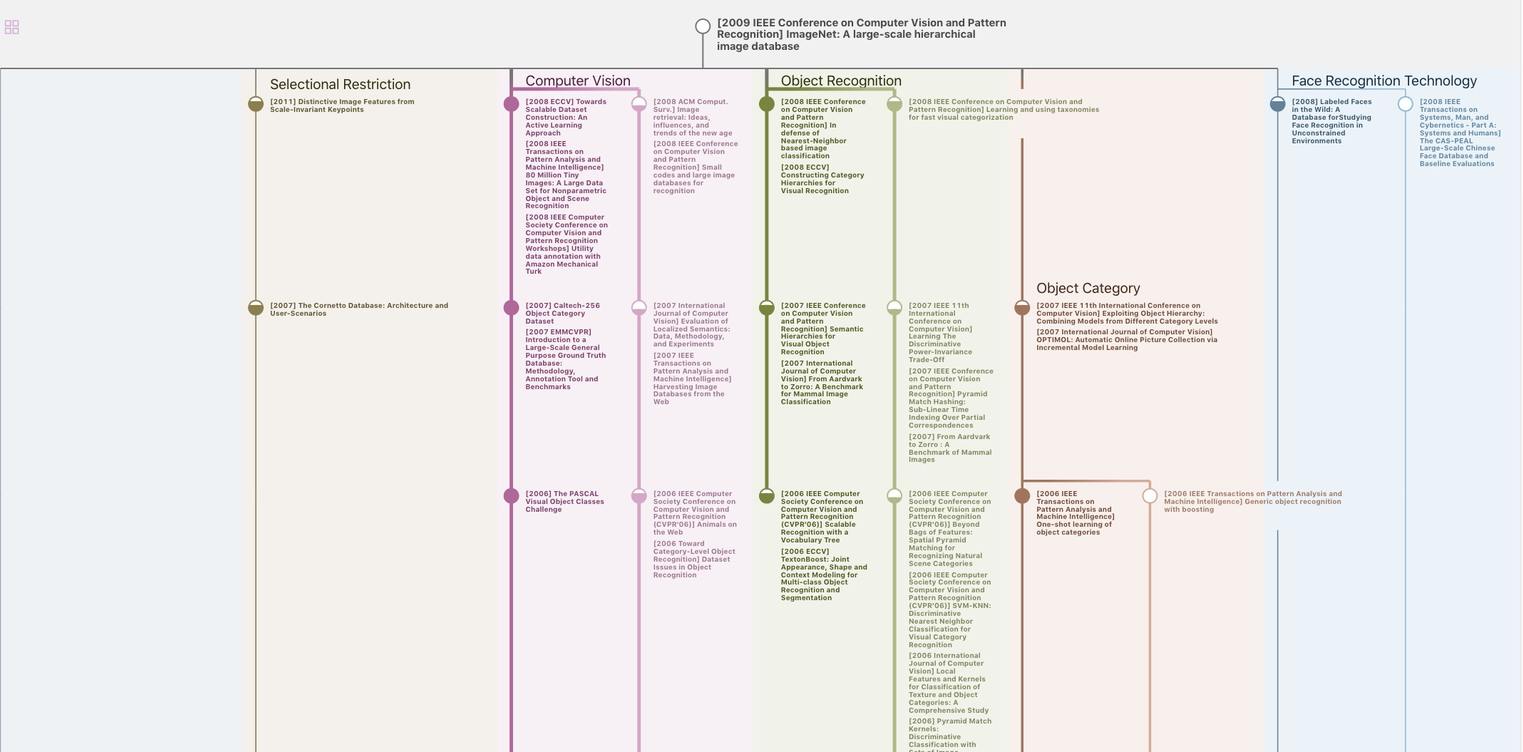
生成溯源树,研究论文发展脉络
Chat Paper
正在生成论文摘要