Enabling Hand Gesture Customization on Wrist-Worn Devices
Conference on Human Factors in Computing Systems(2022)
摘要
ABSTRACTWe present a framework for gesture customization requiring minimal examples from users, all without degrading the performance of existing gesture sets. To achieve this, we first deployed a large-scale study (N=500+) to collect data and train an accelerometer-gyroscope recognition model with a cross-user accuracy of 95.7% and a false-positive rate of 0.6 per hour when tested on everyday non-gesture data. Next, we design a few-shot learning framework which derives a lightweight model from our pre-trained model, enabling knowledge transfer without performance degradation. We validate our approach through a user study (N=20) examining on-device customization from 12 new gestures, resulting in an average accuracy of 55.3%, 83.1%, and 87.2% on using one, three, or five shots when adding a new gesture, while maintaining the same recognition accuracy and false-positive rate from the pre-existing gesture set. We further evaluate the usability of our real-time implementation with a user experience study (N=20). Our results highlight the effectiveness, learnability, and usability of our customization framework. Our approach paves the way for a future where users are no longer bound to pre-existing gestures, freeing them to creatively introduce new gestures tailored to their preferences and abilities.
更多查看译文
关键词
Gesture customization, transfer learning, few-shot learning
AI 理解论文
溯源树
样例
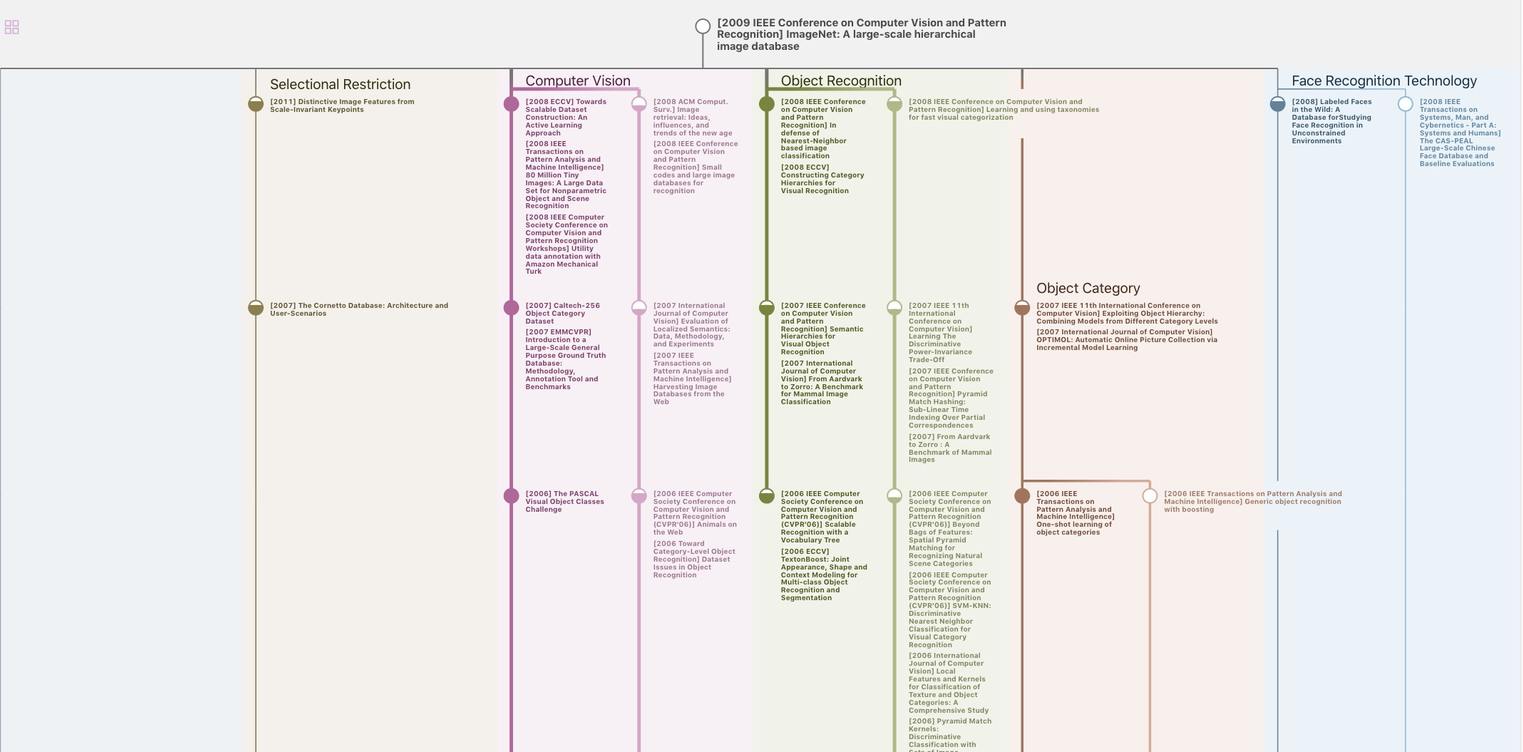
生成溯源树,研究论文发展脉络
Chat Paper
正在生成论文摘要