DAMNETS: A Deep Autoregressive Model for Generating Markovian Network Time Series
arxiv(2022)
摘要
In this work, we introduce DAMNETS, a deep generative model for Markovian network time series. Time series of networks are found in many fields such as trade or payment networks in economics, contact networks in epidemiology or social media posts over time. Generative models of such data are useful for Monte-Carlo estimation and data set expansion, which is of interest for both data privacy and model fitting. Using recent ideas from the Graph Neural Network (GNN) literature, we introduce a novel GNN encoder-decoder structure in which an encoder GNN learns a latent representation of the input graph, and a decoder GNN uses this representation to simulate the network dynamics. We show using synthetic data sets that DAMNETS can replicate features of network topology across time observed in the real world, such as changing community structure and preferential attachment. DAMNETS outperforms competing methods on all of our measures of sample quality over several real and synthetic data sets.
更多查看译文
AI 理解论文
溯源树
样例
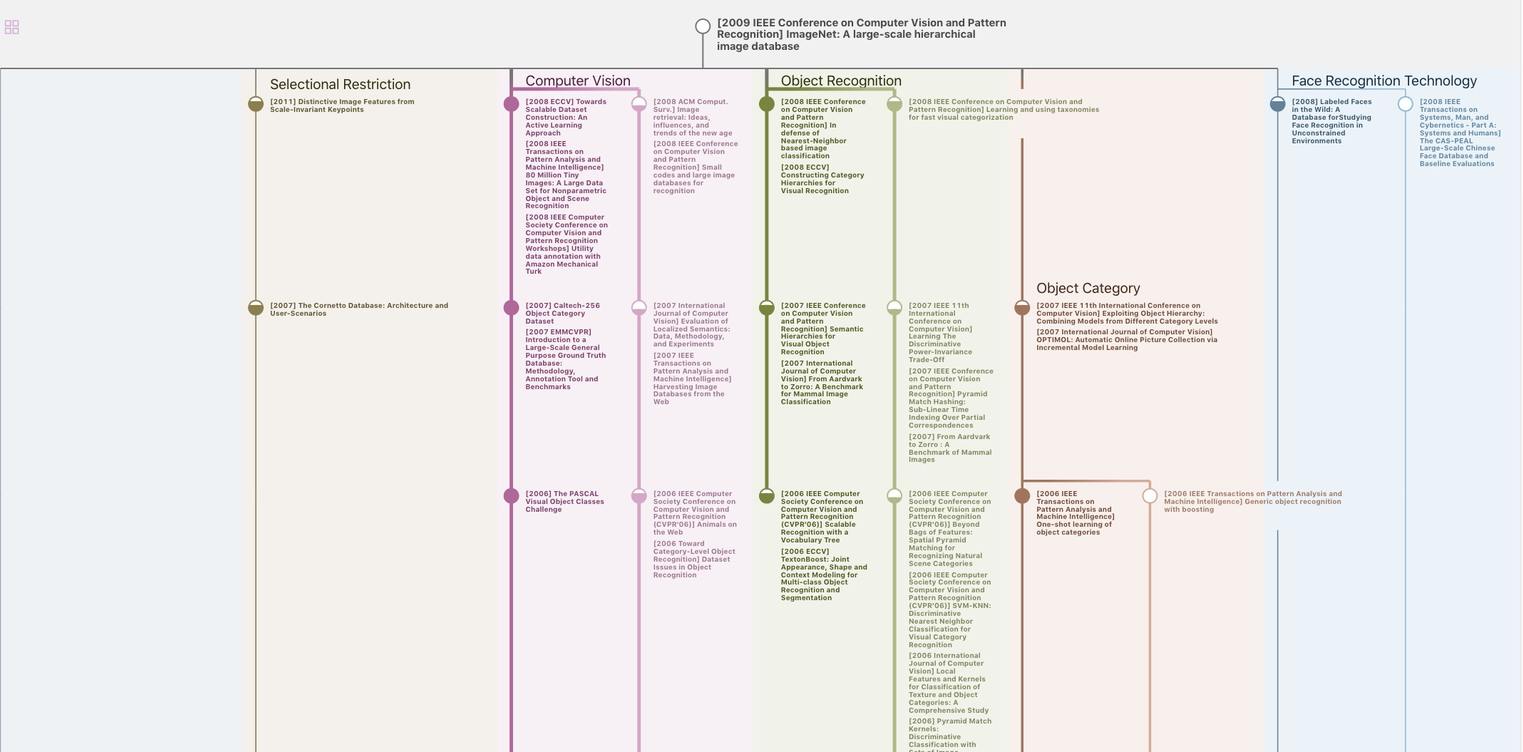
生成溯源树,研究论文发展脉络
Chat Paper
正在生成论文摘要