Parameter tuning in machine learning based on radiomics biomarkers of lung cancer
JOURNAL OF X-RAY SCIENCE AND TECHNOLOGY(2022)
摘要
BACKGROUND: Lung cancer is one of the most common cancers, and early diagnosis and intervention can improve cancer cure rate. OBJECTIVE: To improve predictive performance of radiomics features for lung cancer by tuning the machine learning model parameters. METHODS: Using a dataset involving 263 cases (125 benign and 138 malignant) acquired from our hospital, each classifier model is trained and tested using 237 and 26 cases, respectively. We initially extract 867 radiomics features of CT images for model development and then test 10 feature selections and 7 models to determine the best method. We further tune the parameter of the final model to reach the best performance. The adjusted final model is then validated using 224 cases acquired from Lung Image Database Consortium (LIDC) dataset (64 benign and 160 malignant) with the same set of selected radiomics features. RESULTS: During model development, the feature selection via concave minimization method showthe best performance of area under ROC curve (AUC=0.765), followed by l0-norm regularization (AUC=0.741) and Fisher discrimination criterion (AUC=0.734). Support vector machine (SVM) and random forest (RF) are the top two machine learning algorithms showing the best performance (AUC=0.765 and 0.734, respectively), using by the default parameter. After parameter tuning, SVM with linear kernel achieves the best performance (AUC=0.837), whereas the best tuned RF with the number of trees is 510 and yields a slightly lower performance (AUC=0.775) in 26 test samples data. During model validation, the SVM and RF models yield AUC=0.78 and 0.77, respectively. CONCLUSION: Appropriate quantitative radiomics features and accurate parameters can improve the model's performance to predict lung cancer.
更多查看译文
关键词
Lung neoplasms, machine learning, radiomics, parameter analysis, lung nodule classification
AI 理解论文
溯源树
样例
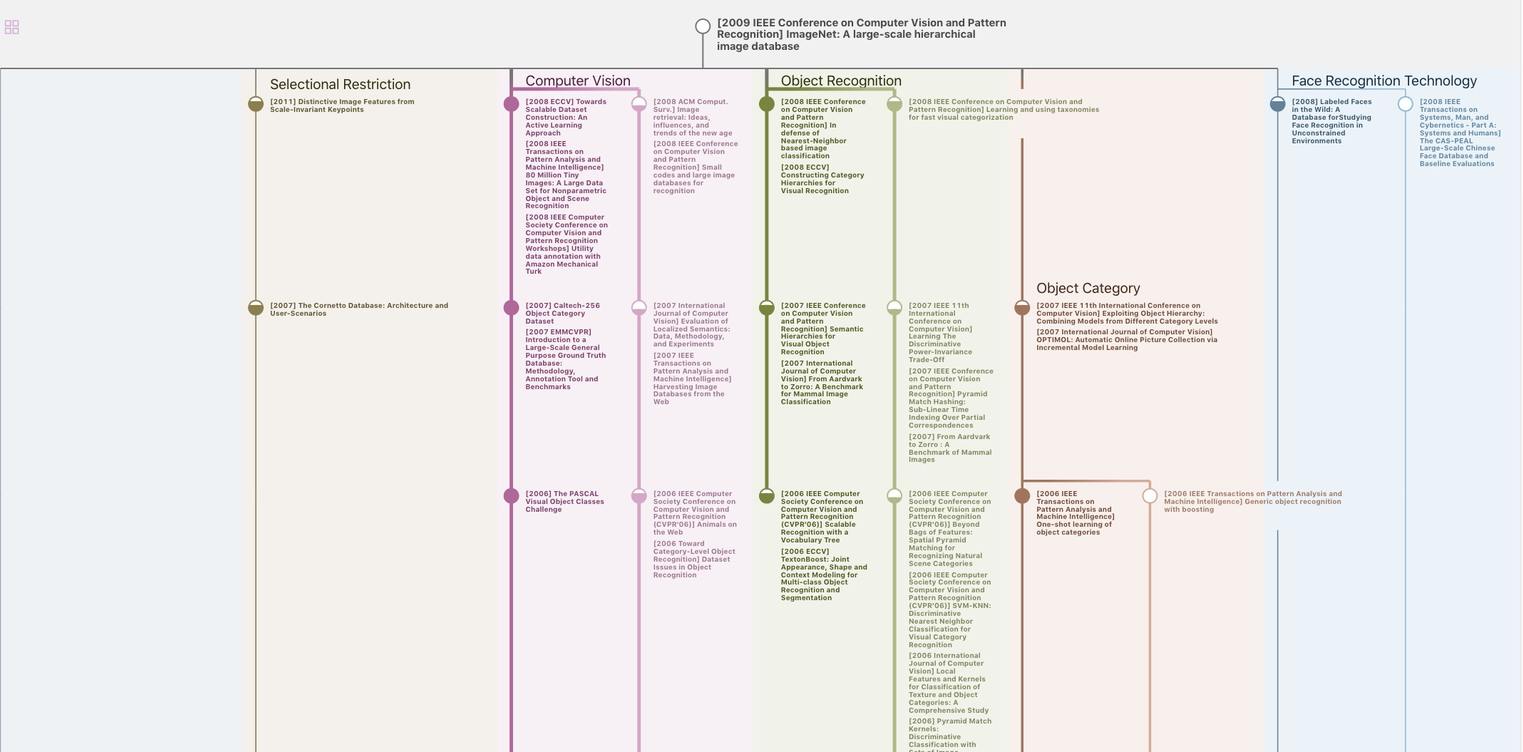
生成溯源树,研究论文发展脉络
Chat Paper
正在生成论文摘要