Adversarially Training MCMC with Non-Volume-Preserving Flows
ENTROPY(2022)
摘要
Recently, flow models parameterized by neural networks have been used to design efficient Markov chain Monte Carlo (MCMC) transition kernels. However, inefficient utilization of gradient information of the target distribution or the use of volume-preserving flows limits their performance in sampling from multi-modal target distributions. In this paper, we treat the training procedure of the parameterized transition kernels in a different manner and exploit a novel scheme to train MCMC transition kernels. We divide the training process of transition kernels into the exploration stage and training stage, which can make full use of the gradient information of the target distribution and the expressive power of deep neural networks. The transition kernels are constructed with non-volume-preserving flows and trained in an adversarial form. The proposed method achieves significant improvement in effective sample size and mixes quickly to the target distribution. Empirical results validate that the proposed method is able to achieve low autocorrelation of samples and fast convergence rates, and outperforms other state-of-the-art parameterized transition kernels in varieties of challenging analytically described distributions and real world datasets.
更多查看译文
关键词
Hamiltonian Monte Carlo, flow models, Markov chain Monte Carlo, statistical pattern recognition, Bayesian machine learning
AI 理解论文
溯源树
样例
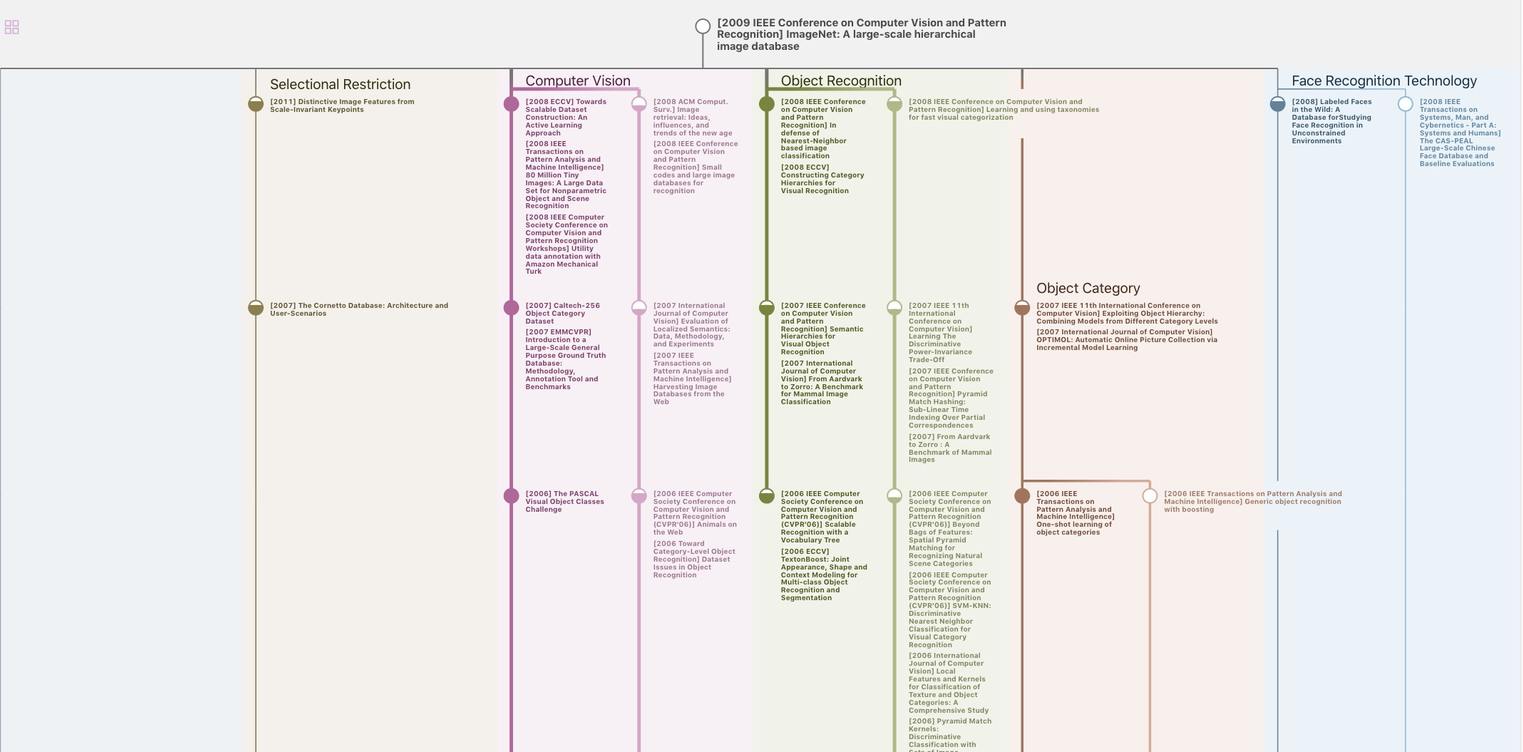
生成溯源树,研究论文发展脉络
Chat Paper
正在生成论文摘要