Investigation of bi-particle states in gate-array-controlled quantum-dot systems aided by machine learning techniques
PHYSICA SCRIPTA(2022)
摘要
Quantum computing architectures require an accurate and efficient description in terms of many-electron states. Recent implementations include quantum dot arrays, where the ground state of a multi q-bit system can be altered by voltages applied to the top gates. An extensive investigation concerning the spectra of the many-electron systems under multiple operation conditions set by external voltages typically requires a relatively large number of Hamiltonian diagonalizations, where the Coulomb interaction is considered in an exact manner. Instead of making exhaustive calculations using high throughput computing, we approach this problem by augmenting numerical diagonalizations with machine learning techniques designed to predict the many-electron eigenvalues and eigenfunctions. To this end, we employ and compare the results from linear regression methods such as multivariate least squares (MLS) as well as non-linear techniques based on kernel ridge regression (KRR), Gaussian process regression (GPR) and artificial neural networks (ANNs). The input feature vectors are assembled from readily available information comprised from a binary representation of the potential and the strength of the Coulomb interaction. Furthermore, employing a linear classifier, we establish a rule for detecting a singlet-triplet transition which may arise for certain potential configurations.
更多查看译文
关键词
quantum dots, many-body systems, exact-diagonalization, machine learning
AI 理解论文
溯源树
样例
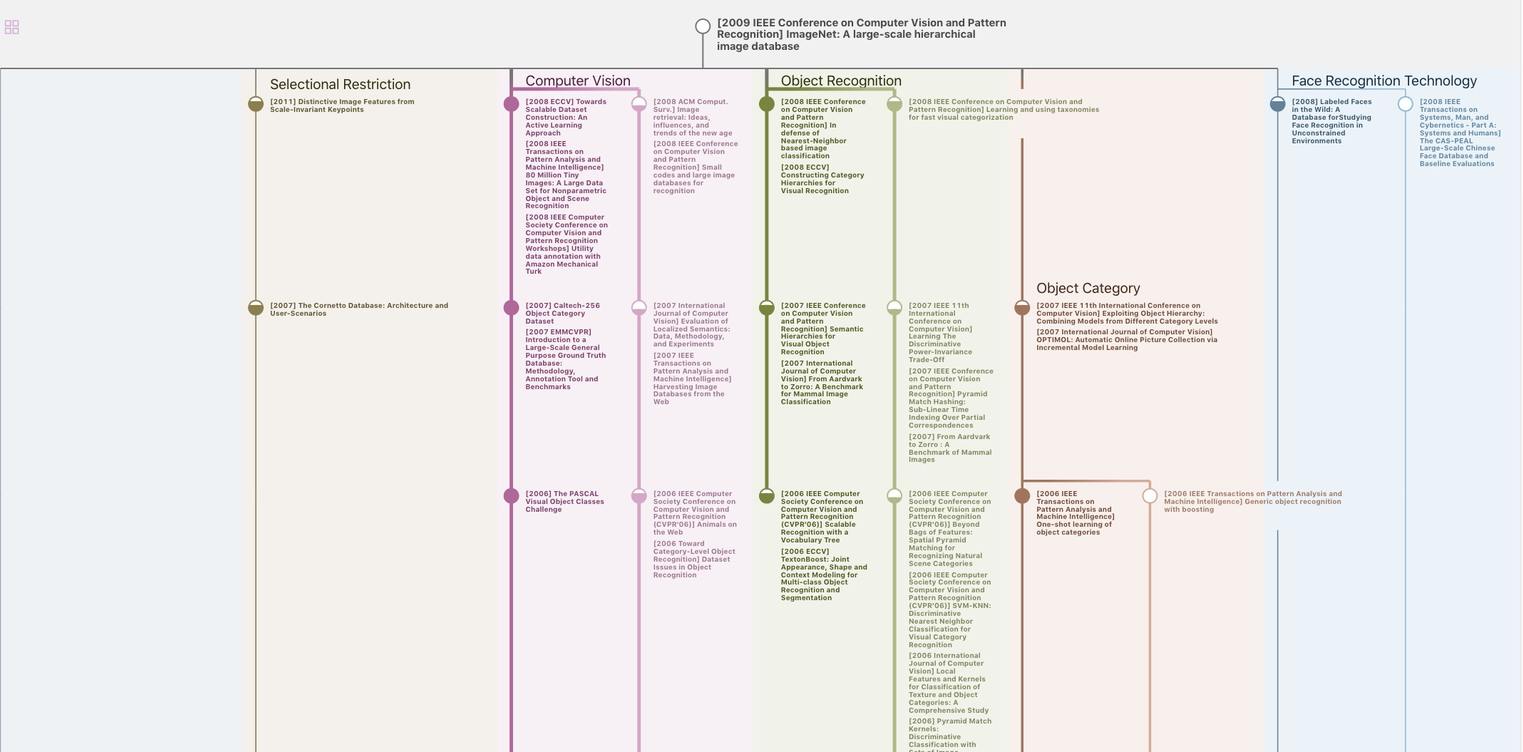
生成溯源树,研究论文发展脉络
Chat Paper
正在生成论文摘要