Metaphors in Pre-Trained Language Models: Probing and Generalization Across Datasets and Languages
PROCEEDINGS OF THE 60TH ANNUAL MEETING OF THE ASSOCIATION FOR COMPUTATIONAL LINGUISTICS (ACL 2022), VOL 1: (LONG PAPERS)(2022)
摘要
Human languages are full of metaphorical expressions. Metaphors help people understand the world by connecting new concepts and domains to more familiar ones. Large pre-trained language models (PLMs) are therefore assumed to encode metaphorical knowledge useful for NLP systems. In this paper, we investigate this hypothesis for PLMs, by probing metaphoricity information in their encodings, and by measuring the cross-lingual and cross-dataset generalization of this information. We present studies in multiple metaphor detection datasets and in four languages (i.e., English, Spanish, Russian, and Farsi). Our extensive experiments suggest that contextual representations in PLMs do encode metaphorical knowledge, and mostly in their middle layers. The knowledge is transferable between languages and datasets, especially when the annotation is consistent across training and testing sets. Our findings give helpful insights for both cognitive and NLP scientists.
更多查看译文
关键词
metaphors,language models,languages,generalization
AI 理解论文
溯源树
样例
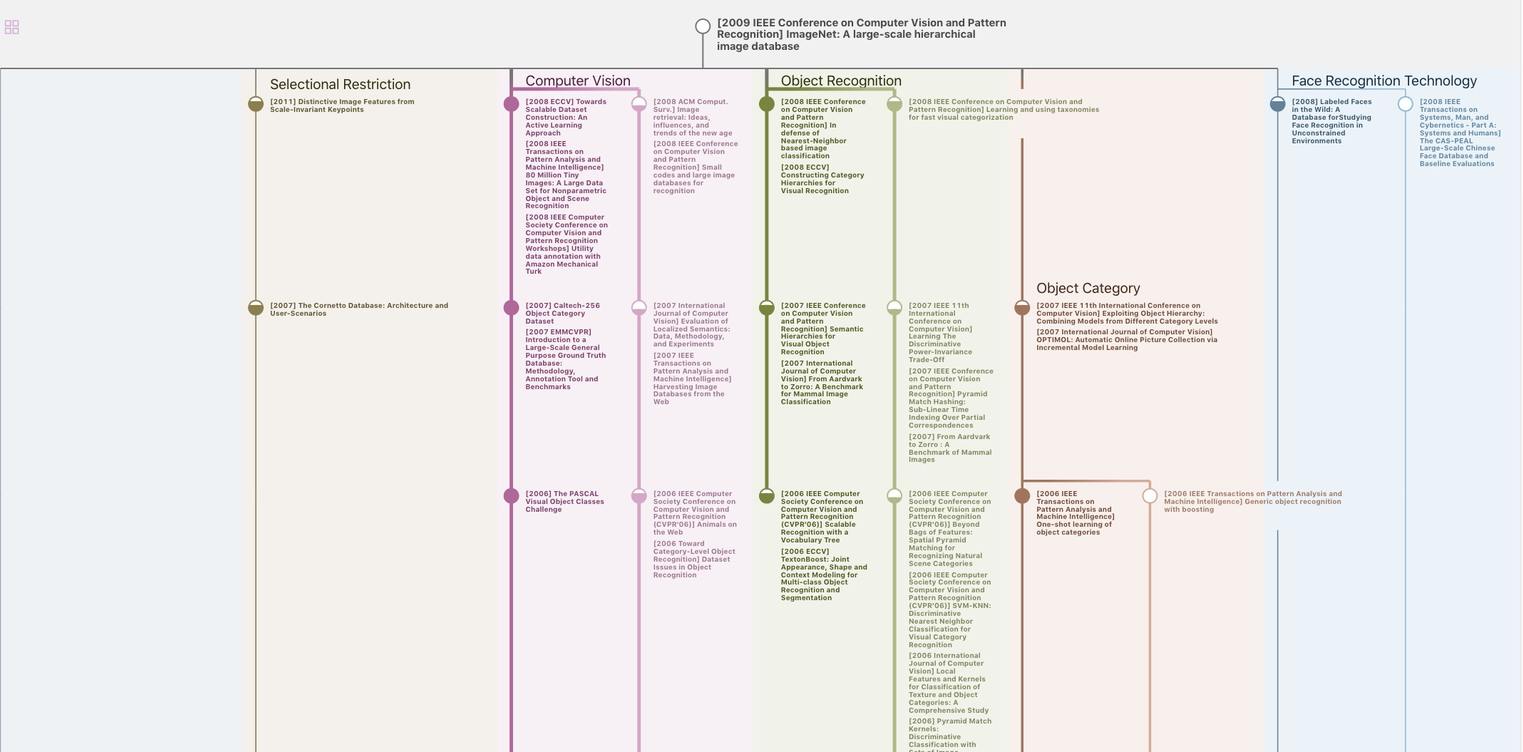
生成溯源树,研究论文发展脉络
Chat Paper
正在生成论文摘要