GraphBLAS on the Edge: Anonymized High Performance Streaming of Network Traffic
2022 IEEE High Performance Extreme Computing Conference (HPEC)(2022)
摘要
Long range detection is a cornerstone of defense in many operating domains (land, sea, undersea, air, space,…,). In the cyber domain, long range detection requires the analysis of significant network traffic from a variety of observatories and outposts. Construction of anonymized hypersparse traffic matrices on edge network devices can be a key enabler by providing significant data compression in a rapidly analyzable format that protects privacy. GraphBLAS is ideally suited for both constructing and analyzing anonymized hypersparse traffic matrices. The performance of GraphBLAS on an Accolade Technologies edge network device is demonstrated on a near worse case traffic scenario using a continuous stream of CAIDA Telescope darknet packets. The performance for varying numbers of traffic buffers, threads, and processor cores is explored. Anonymized hypersparse traffic matrices can be constructed at a rate of over 50,000,000 packets per second; exceeding a typical 400 Gigabit network link. This performance demonstrates that anonymized hypersparse traffic matrices are readily computable on edge network devices with minimal compute resources and can be a viable data product for such devices.
更多查看译文
关键词
Internet defense,packet capture,streaming graphs,hypersparse matrices
AI 理解论文
溯源树
样例
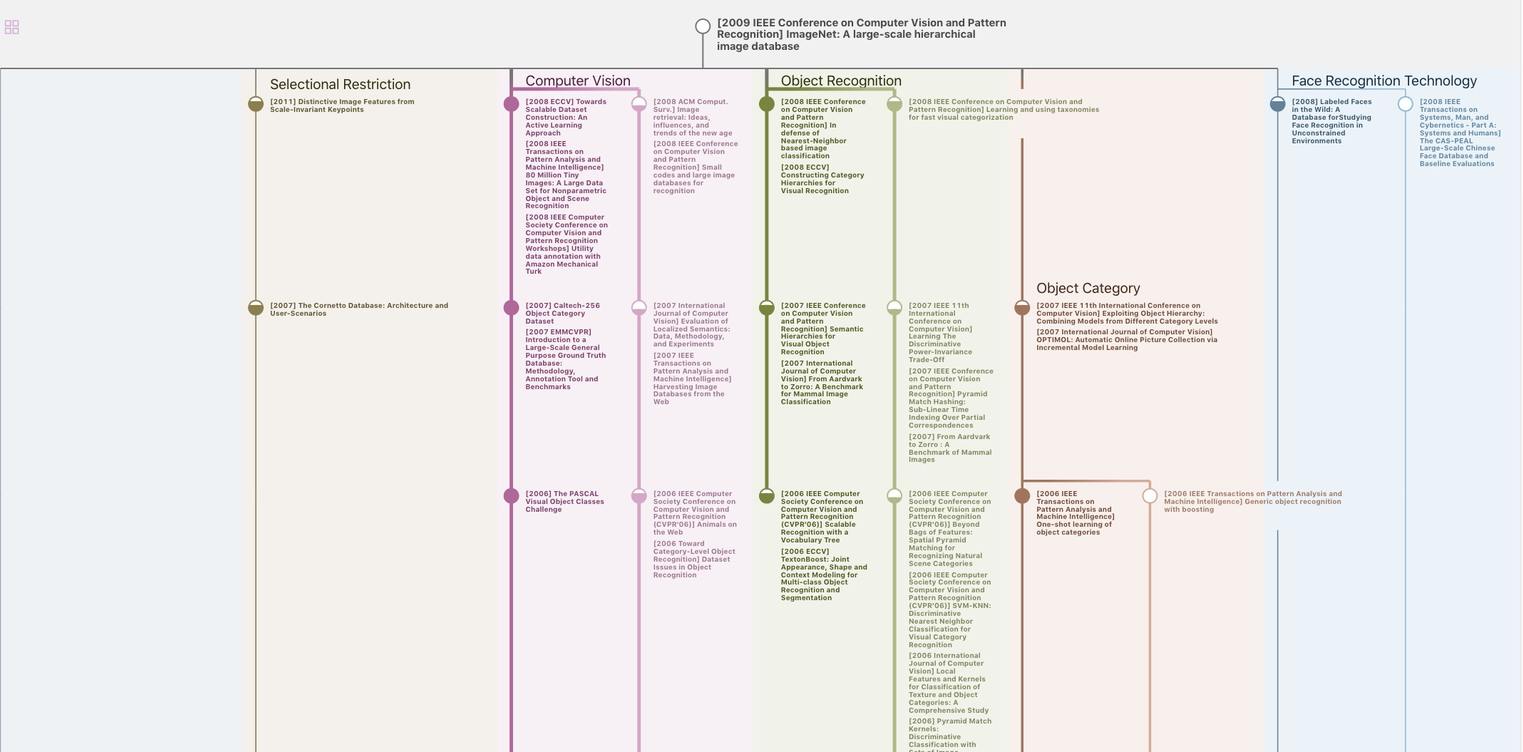
生成溯源树,研究论文发展脉络
Chat Paper
正在生成论文摘要