Simultaneous Identification and Denoising of Dynamical Systems
arxiv(2023)
摘要
In recent years there has been a push to discover the governing equations dynamical systems directly from measurements of the state, often motivated by systems that are too complex to directly model. Although there has been substantial work put into such a discovery, doing so in the case of large noise has proved challenging. Here we develop an algorithm for Simultaneous Identification and Denoising of a Dynamical System (SIDDS). We infer the noise in the state measurements by requiring that the denoised data satisfies the dynamical system with an equality constraint. This is unlike existing work where the mismatch in the dynamical system is treated as a penalty in the objective. We assume the dynamics is represented in a pre-defined basis and develop a sequential quadratic programming approach to solve the SIDDS problem featuring a direct solution of KKT system with a specialized preconditioner. In addition, we show how we can include sparsity promoting regularization using an iteratively reweighted least squares approach. The resulting algorithm leads to estimates of the dynamical system that approximately achieve the Cram\'er-Rao lower bound and, with sparsity promotion, can correctly identify the sparsity structure for higher levels of noise than existing techniques. Moreover, because SIDDS decouples the data from the evolution of the dynamical system, we show how to modify the problem to accurately identify systems from low sample rate measurements. The inverse problem approach and solution framework used by SIDDS has the potential to be expanded to related problems identifying governing equations from noisy data.
更多查看译文
关键词
dynamical systems,denoising,identification
AI 理解论文
溯源树
样例
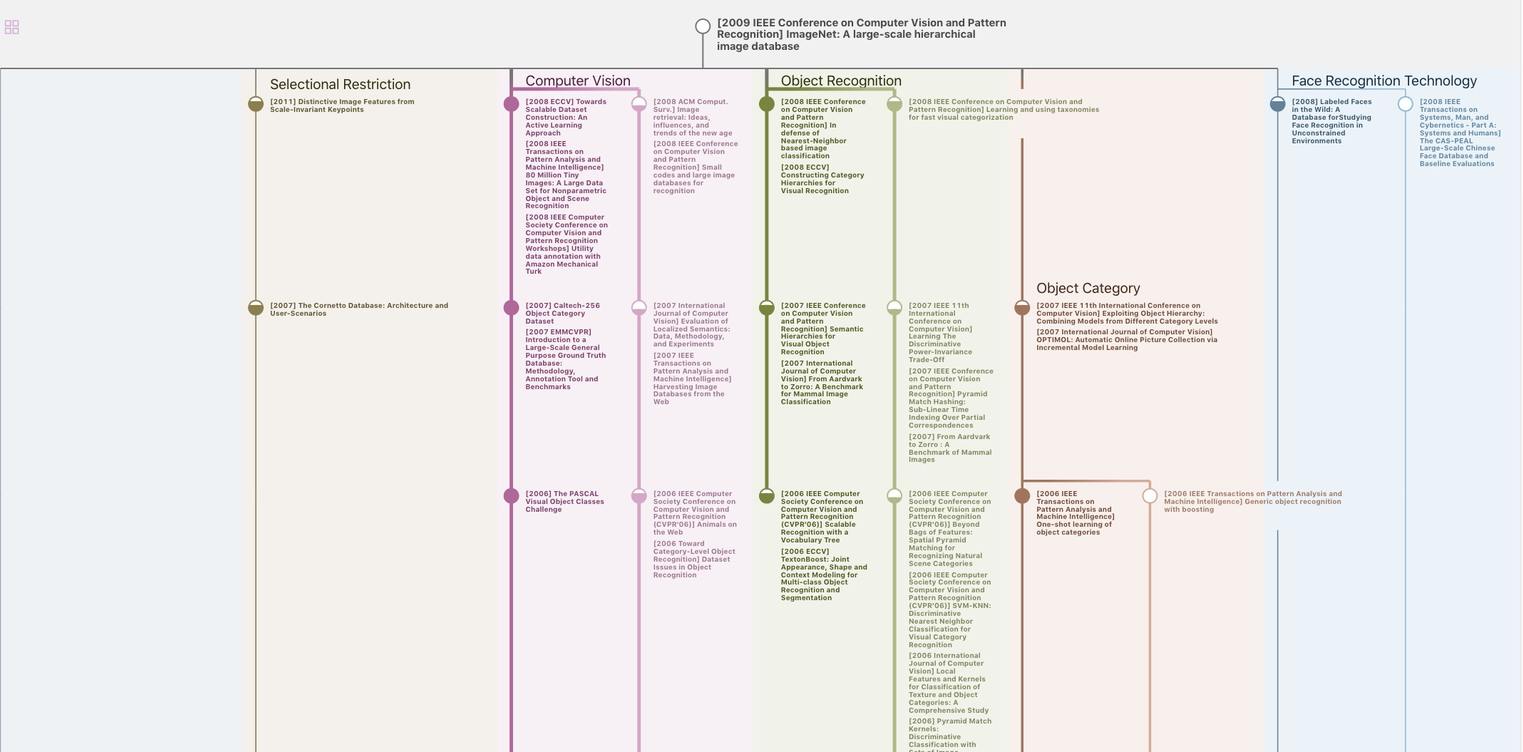
生成溯源树,研究论文发展脉络
Chat Paper
正在生成论文摘要