EnHDC: Ensemble Learning for Brain-Inspired Hyperdimensional Computing
IEEE Embedded Systems Letters(2023)
Abstract
Recently, brain-inspired hyperdimensional computing (HDC) becomes an emerging computational scheme that has achieved success in various domains, such as human activity recognition, voice recognition, and bio-medical signal classification. HDC mimics the brain cognition and leverages high-dimensional vectors (e.g., 10 000 dimensions) with fully distributed holographic representation and (pseudo-)randomness. Ensemble learning is a classical learning method utilizing a group of weak learners to form a strong learner, which aims to increase the accuracy of the model. This letter presents a systematic effort in exploring ensemble learning in the context of HDC and proposes an ensemble HDC model referred to as EnHDC. EnHDC uses a majority voting-based mechanism to synergistically integrate the prediction outcomes of multiple base HDC classifiers. To enhance the diversity of base classifiers, we vary the encoding mechanisms, dimensions, and data width settings among base classifiers. By applying EnHDC on a wide range of applications, results show that EnHDC can achieve on average 3.2% accuracy improvement over a single HDC classifier. Further, we show that EnHDC with reduced dimensionality can achieve similar or even surpass the accuracy of baseline HDC with higher dimensionality. This leads to a 20% reduction of storage requirement of the HDC model, which can enhance the efficiency of HDC enabled on low-power computing platforms.
MoreTranslated text
Key words
Encoding,Training,Brain modeling,Computational modeling,Speech recognition,Biological system modeling,Indexes,Ensemble learning,hyperdimensional computing (HDC),memory-efficient computing
AI Read Science
Must-Reading Tree
Example
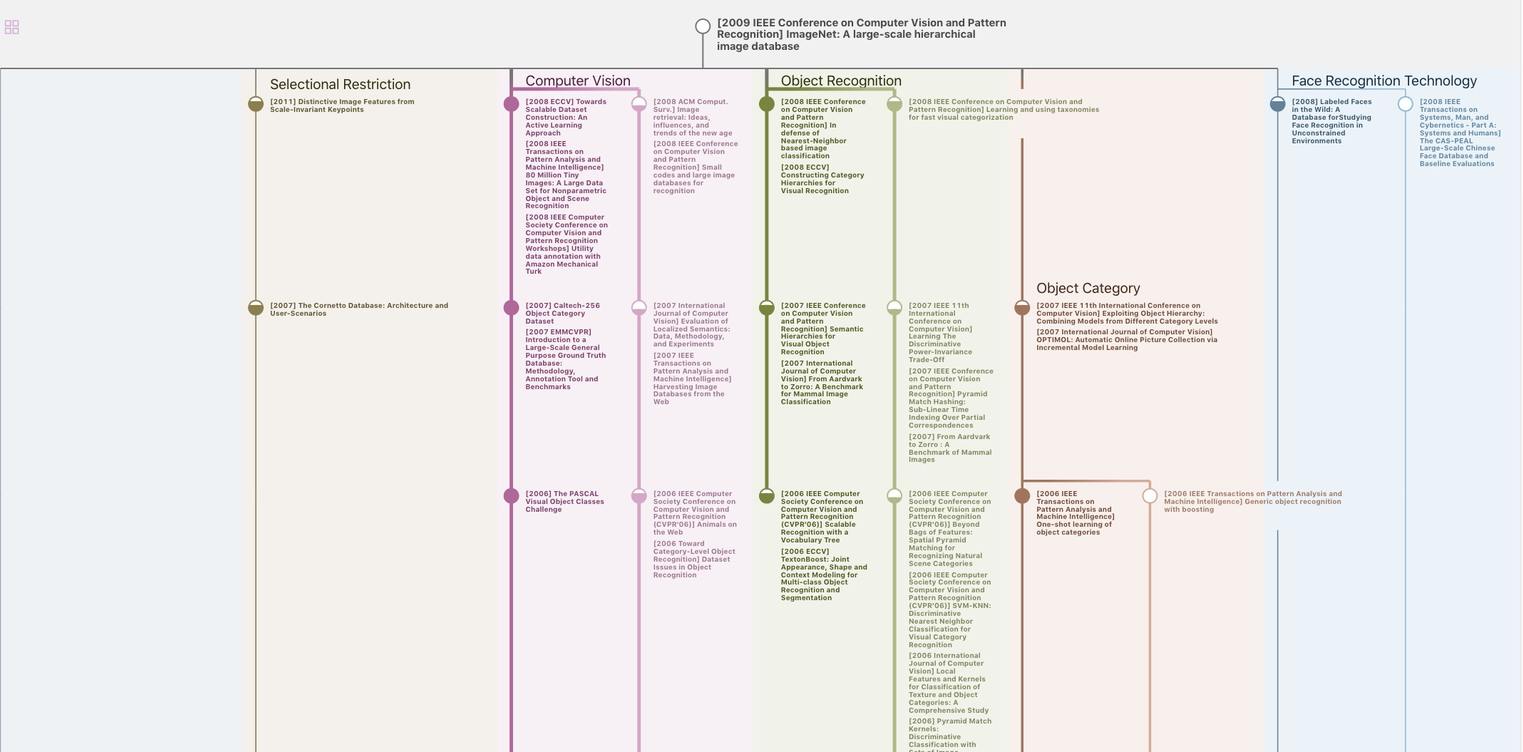
Generate MRT to find the research sequence of this paper
Chat Paper
Summary is being generated by the instructions you defined