Striking a Balance: Alleviating Inconsistency in Pre-trained Models for Symmetric Classification Tasks
Findings(2022)
Abstract
While fine-tuning pre-trained models for downstream classification is the conventional paradigm in NLP, often task-specific nuances may not get captured in the resultant models.Specifically, for tasks that take two inputs and require the output to be invariant of the order of the inputs, inconsistency is often observed in the predicted labels or confidence scores.We highlight this model shortcoming and apply a consistency loss function to alleviate inconsistency in symmetric classification.Our results show an improved consistency in predictions for three paraphrase detection datasets without a significant drop in the accuracy scores.We examine the classification performance of six datasets (both symmetric and non-symmetric) to showcase the strengths and limitations of our approach.
MoreTranslated text
Key words
Dependency Parsing,Neural Machine Translation,Topic Modeling,Language Modeling,Syntax-based Translation Models
AI Read Science
Must-Reading Tree
Example
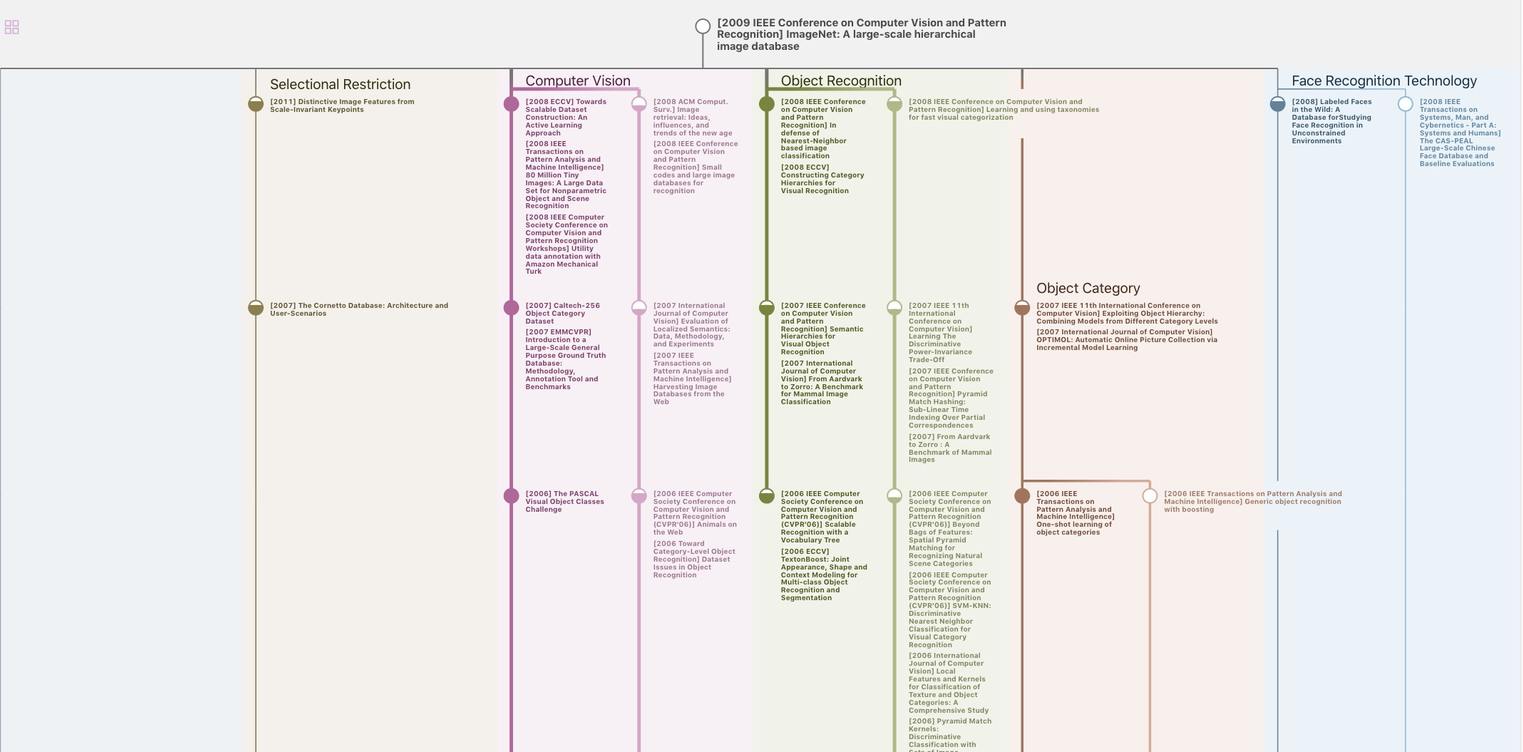
Generate MRT to find the research sequence of this paper
Chat Paper
Summary is being generated by the instructions you defined