A methodological framework for AI-assisted diagnosis of active aortitis using radiomic analysis of FDG PET–CT images: Initial analysis
Journal of Nuclear Cardiology(2022)
摘要
Background The aim of this study was to explore the feasibility of assisted diagnosis of active (peri-)aortitis using radiomic imaging biomarkers derived from [ 18 F]-Fluorodeoxyglucose Positron Emission Tomography–Computed Tomography (FDG PET–CT) images. Methods The aorta was manually segmented on FDG PET–CT in 50 patients with aortitis and 25 controls. Radiomic features (RF) ( n = 107), including SUV (Standardized Uptake Value) metrics, were extracted from the segmented data and harmonized using the ComBat technique. Individual RFs and groups of RFs (i.e., signatures) were used as input in Machine Learning classifiers. The diagnostic utility of these classifiers was evaluated with area under the receiver operating characteristic curve (AUC) and accuracy using the clinical diagnosis as the ground truth. Results Several RFs had high accuracy, 84% to 86%, and AUC scores 0.83 to 0.97 when used individually. Radiomic signatures performed similarly, AUC 0.80 to 1.00. Conclusion A methodological framework for a radiomic-based approach to support diagnosis of aortitis was outlined. Selected RFs, individually or in combination, showed similar performance to the current standard of qualitative assessment in terms of AUC for identifying active aortitis. This framework could support development of a clinical decision-making tool for a more objective and standardized assessment of aortitis.
更多查看译文
关键词
Large-vessel vasculitis,FDG PET/CT,Radiomic feature analysis,Diagnosis,Giant cell arteritis
AI 理解论文
溯源树
样例
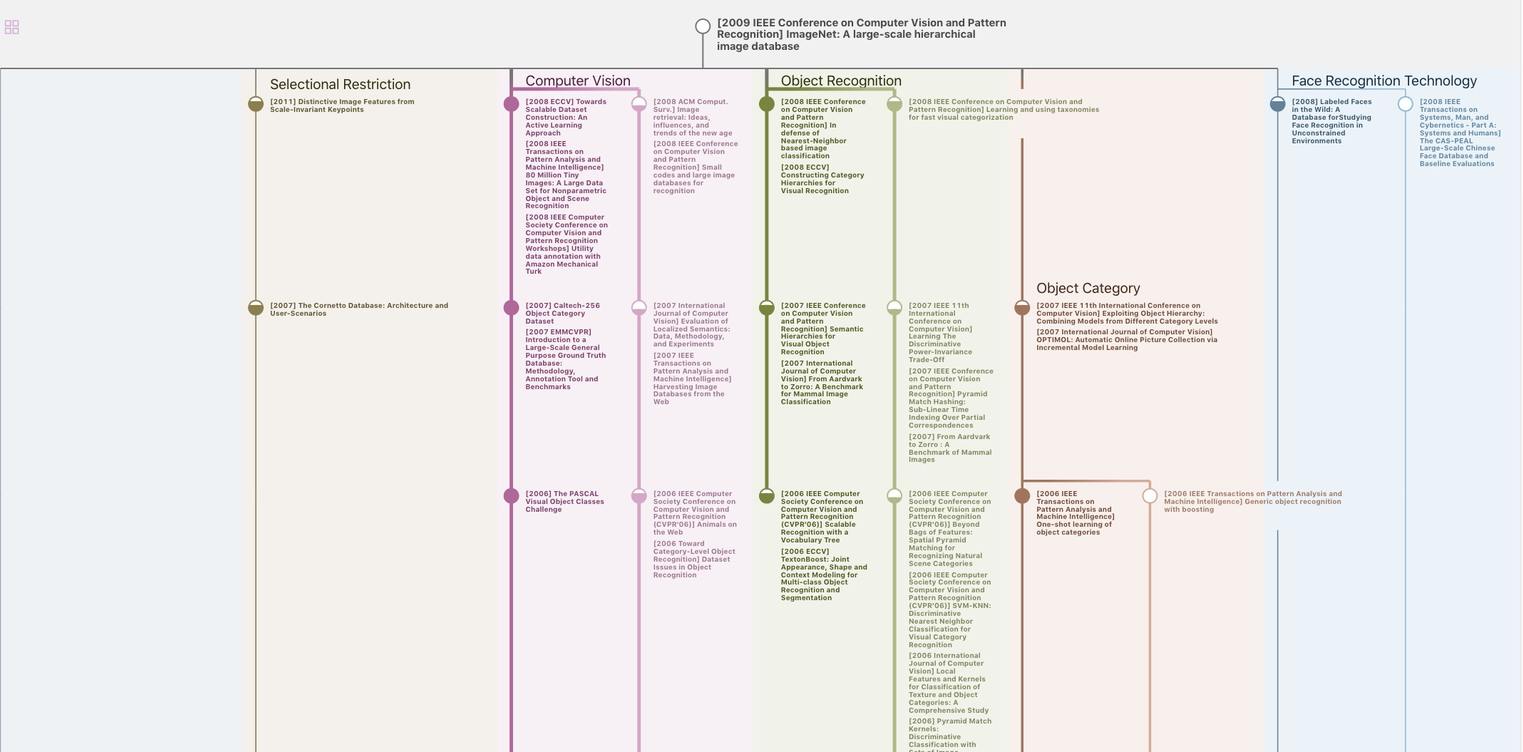
生成溯源树,研究论文发展脉络
Chat Paper
正在生成论文摘要