Self-attentional microvessel segmentation via squeeze-excitation transformer Unet
COMPUTERIZED MEDICAL IMAGING AND GRAPHICS(2022)
摘要
Automatic vessel segmentation is a key step of clinical or pre-clinical vessel bio-markers for clinical diagnosis. In previous research, the segmentation architectures are mainly based on Convolutional Neural Networks (CNN). However, due to the limitation of the receipt of field (ROF) of convolution operation, it is difficult to further improve the accuracy of the CNN-based methods. To solve this problem, a Squeeze-Excitation Transformer U-net (SETUnet) is proposed to break the ROF limitation of CNN. The proposed squeeze-excitation Transformer can introduce the self attention mechanism into the vessel segmentation task by generating a global attention mapping according to the entire vessel image. To test the performance of the proposed SETUnet, the SETUnet is trained and tested on several public vessel data-sets. The results show that the SETUnet outperforms several state-of-the-art vessel segmentation neural networks, especially on the connectivity of the segmented vessels.
更多查看译文
关键词
Microvessel segmentation, Deep neural network, Self attention mechanism, Fundus image, Optical coherence tomography
AI 理解论文
溯源树
样例
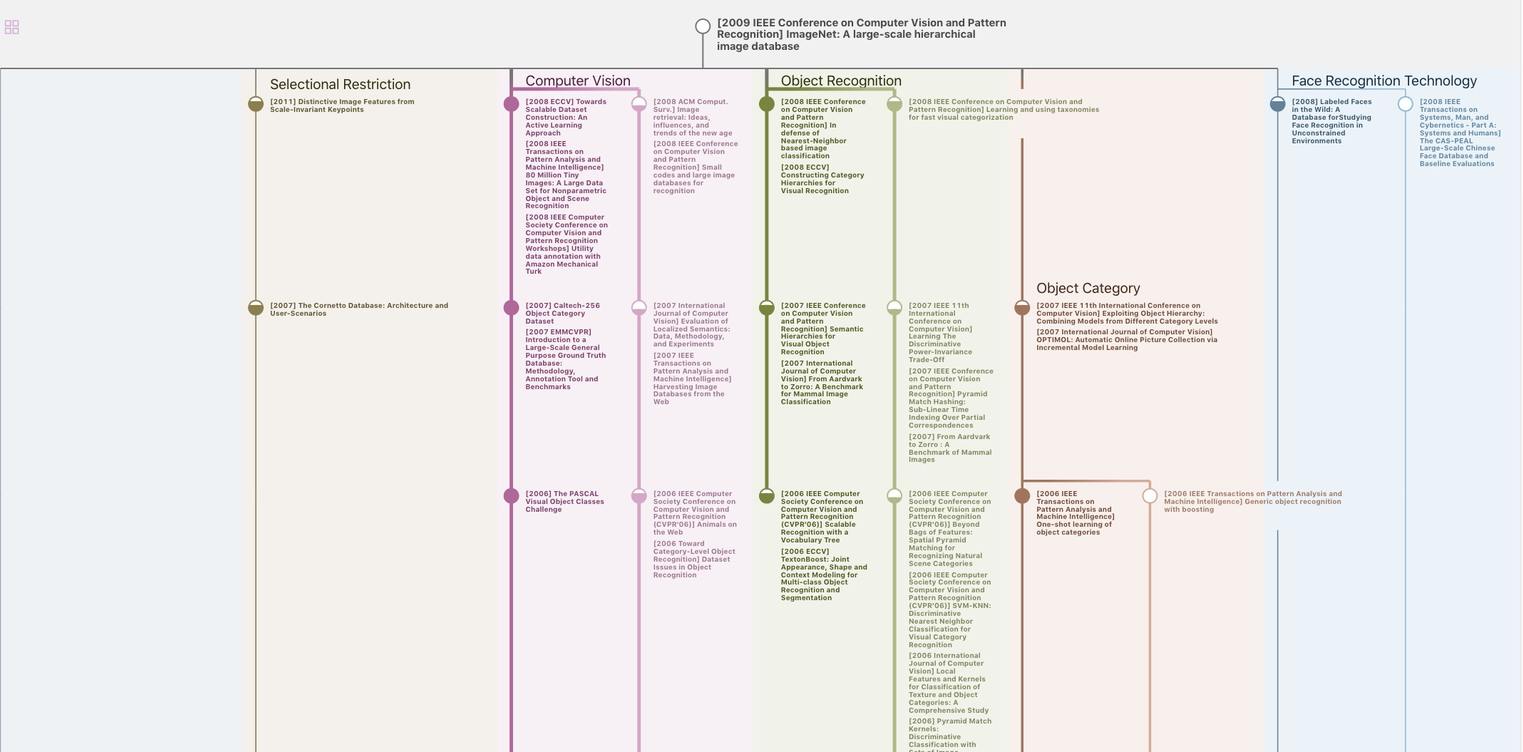
生成溯源树,研究论文发展脉络
Chat Paper
正在生成论文摘要