Semisupervised Cross-Scale Graph Prototypical Network for Hyperspectral Image Classification
IEEE transactions on neural networks and learning systems(2023)
摘要
In practice, the acquirement of labeled samples for hyperspectral image (HSI) is time-consuming and labor-intensive. It frequently induces the trouble of model overfitting and performance degradation for the supervised methodologies in HSI classification (HSIC). Fortunately, semisupervised learning can alleviate this deficiency, and graph convolutional network (GCN) is one of the most effective semisupervised approaches, which propagates the node information from each other in a transductive manner. In this study, we propose a cross-scale graph prototypical network (X-GPN) to achieve semisupervised high-quality HSIC. Specifically, considering the multiscale appearance of the land covers in the same remotely captured scene, we involve the neighborhoods of different scales to construct the adjacency matrices and simultaneously design a multibranch framework to investigate the abundant spectral–spatial features through graph convolutions. Furthermore, to exploit the complementary information between different scales, we simply employ the standard 1-D convolution to excavate the dependence of the intranode and concatenate the output with the features generated from other scales. Intuitively, different branches for various samples should have different importance to predict their categories. Thus, we develop a self-branch attentional addition (SBAA) module to adaptively highlight the most critical features produced by multiple branches. In addition, different from previous GCN for HSIC, we devise an innovative prototypical layer comprising a distance-based cross-entropy (DCE) loss function and a novel temporal entropy-based regularizer (TER), which can enhance the discrimination and representativeness of the node features and prototypes actively. Extensive experiments demonstrate that the proposed X-GPN is superior to the classic and state-of-the-art (SOTA) methods in terms of the classification performance.
更多查看译文
关键词
Convolution,Prototypes,Hyperspectral imaging,Feature extraction,Chebyshev approximation,Training,Telecommunications,Cross-scale,graph convolutional network (GCN),hyperspectral image (HSI) classification,semisupervised learning (SSL)
AI 理解论文
溯源树
样例
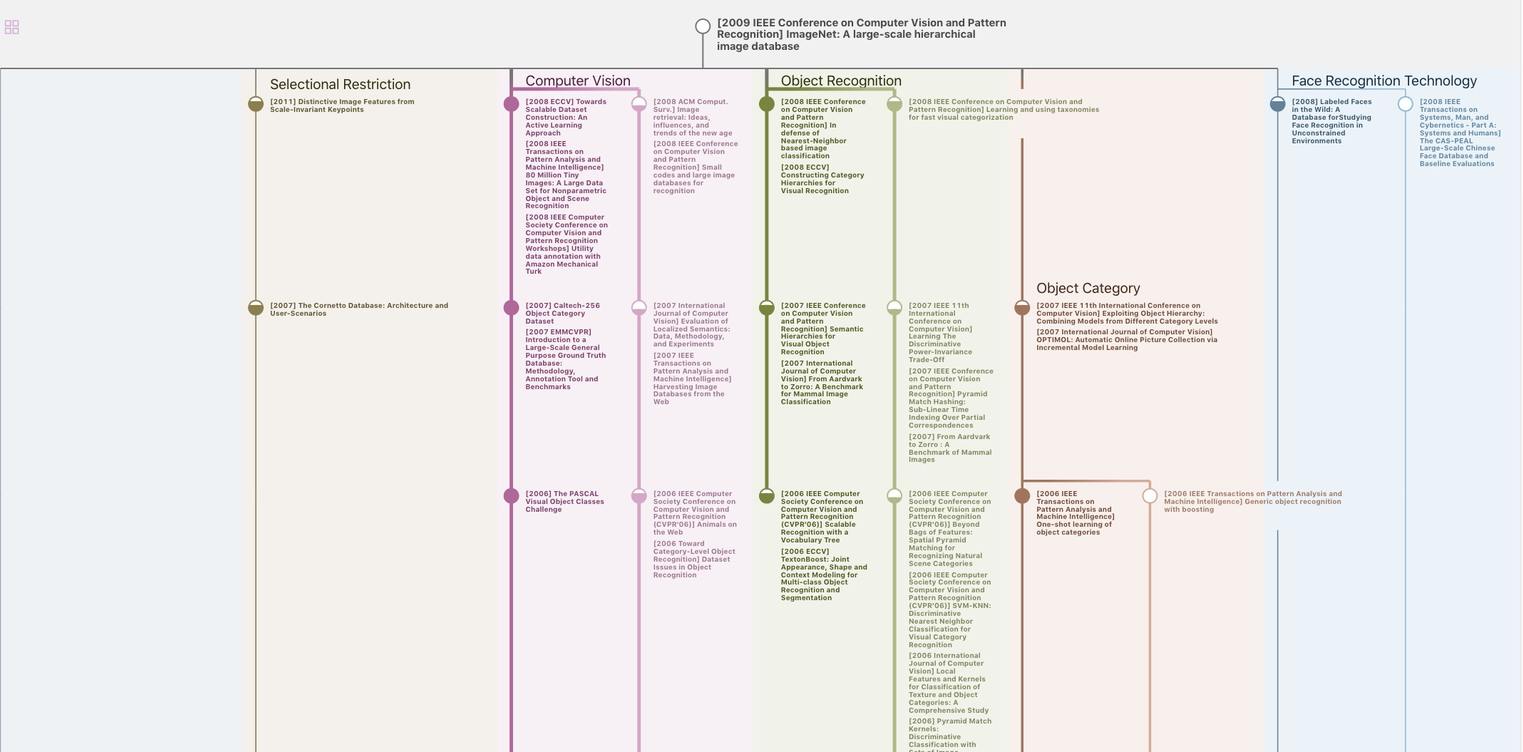
生成溯源树,研究论文发展脉络
Chat Paper
正在生成论文摘要