MERLIN -- Malware Evasion with Reinforcement LearnINg
arXiv (Cornell University)(2022)
摘要
In addition to signature-based and heuristics-based detection techniques, Machine learning (ML) is being widely used to generalize to new never-before-seen malicious software (malware). However, it has been demonstrated that ML models can be fooled by tricking the classifier into returning the incorrect label. These studies usually rely on a prediction score that is fragile to gradient-based attacks for instance. In the context of a more realistic situation where an attacker has very little information about the outputs of a malware detection engine, modest evasion rates are achieved. In this paper, we propose a method using Reinforcement Learning with DQN and REINFORCE algorithms to challenge two state-of-the-art Machine Learning based detection engines (MalConv \& EMBER) and a commercial AV classified by Gartner as a leader in 2021. Our stateful method combines several actions modifying a Windows Portable Execution (PE) file without breaking its functionalities. Our method also identifies which actions perform better and compiles a detailed vulnerability report to help mitigate the evasion. We demonstrate that REINFORCE achieves very good evasion rates even on a commercial AV with low provided information.
更多查看译文
关键词
malware evasion,reinforcement learning
AI 理解论文
溯源树
样例
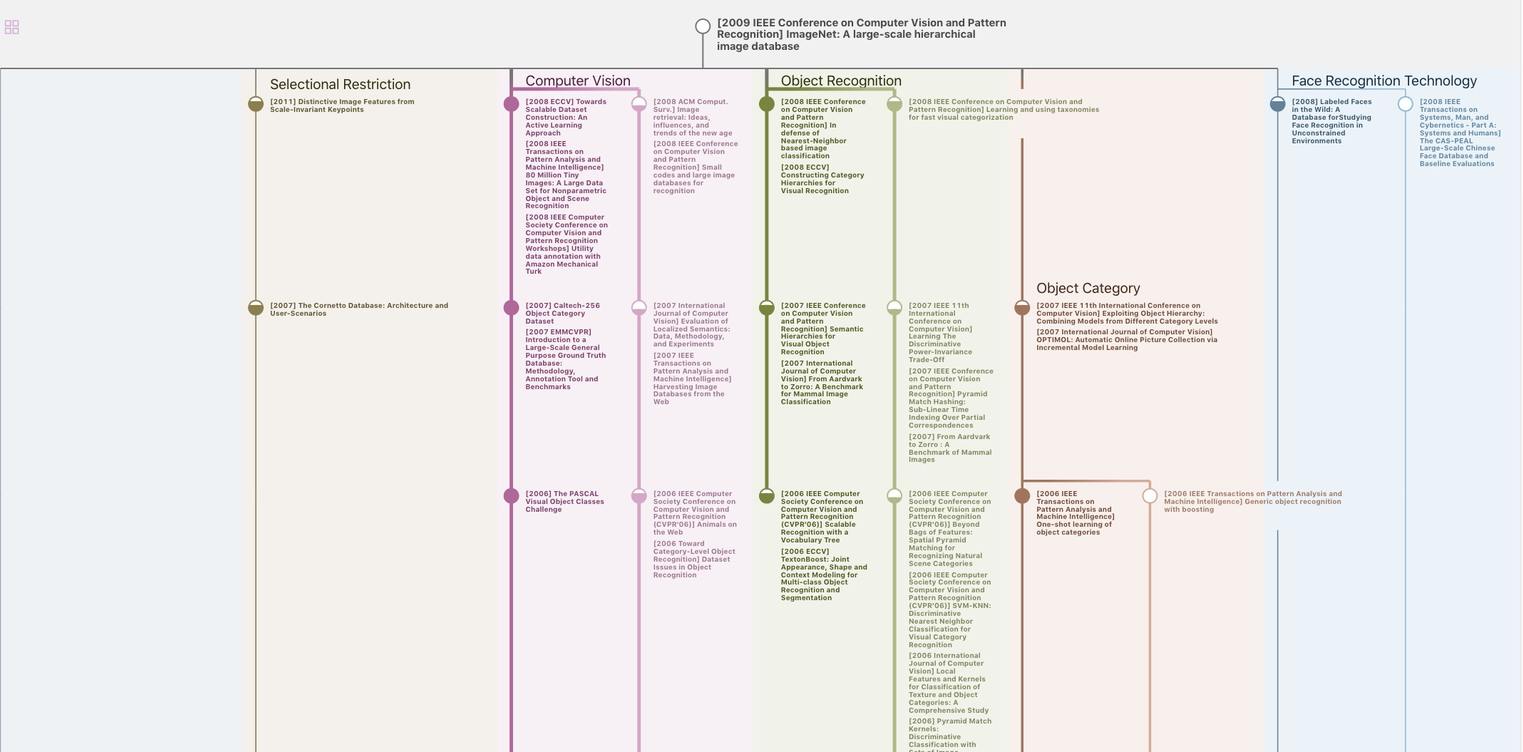
生成溯源树,研究论文发展脉络
Chat Paper
正在生成论文摘要