Accelerating Bayesian Optimization for Biological Sequence Design with Denoising Autoencoders.
International Conference on Machine Learning(2022)
摘要
Bayesian optimization (BayesOpt) is a gold standard for query-efficient continuous optimization. However, its adoption for drug design has been hindered by the discrete, high-dimensional nature of the decision variables. We develop a new approach (LaMBO) which jointly trains a denoising autoencoder with a discriminative multi-task Gaussian process head, allowing gradient-based optimization of multi-objective acquisition functions in the latent space of the autoencoder. These acquisition functions allow LaMBO to balance the explore-exploit tradeoff over multiple design rounds, and to balance objective tradeoffs by optimizing sequences at many different points on the Pareto frontier. We evaluate LaMBO on two small-molecule design tasks, and introduce new tasks optimizing in silico and in vitro properties of large-molecule fluorescent proteins. In our experiments LaMBO outperforms genetic optimizers and does not require a large pretraining corpus, demonstrating that BayesOpt is practical and effective for biological sequence design.
更多查看译文
关键词
bayesian optimization,biological sequence design,denoising
AI 理解论文
溯源树
样例
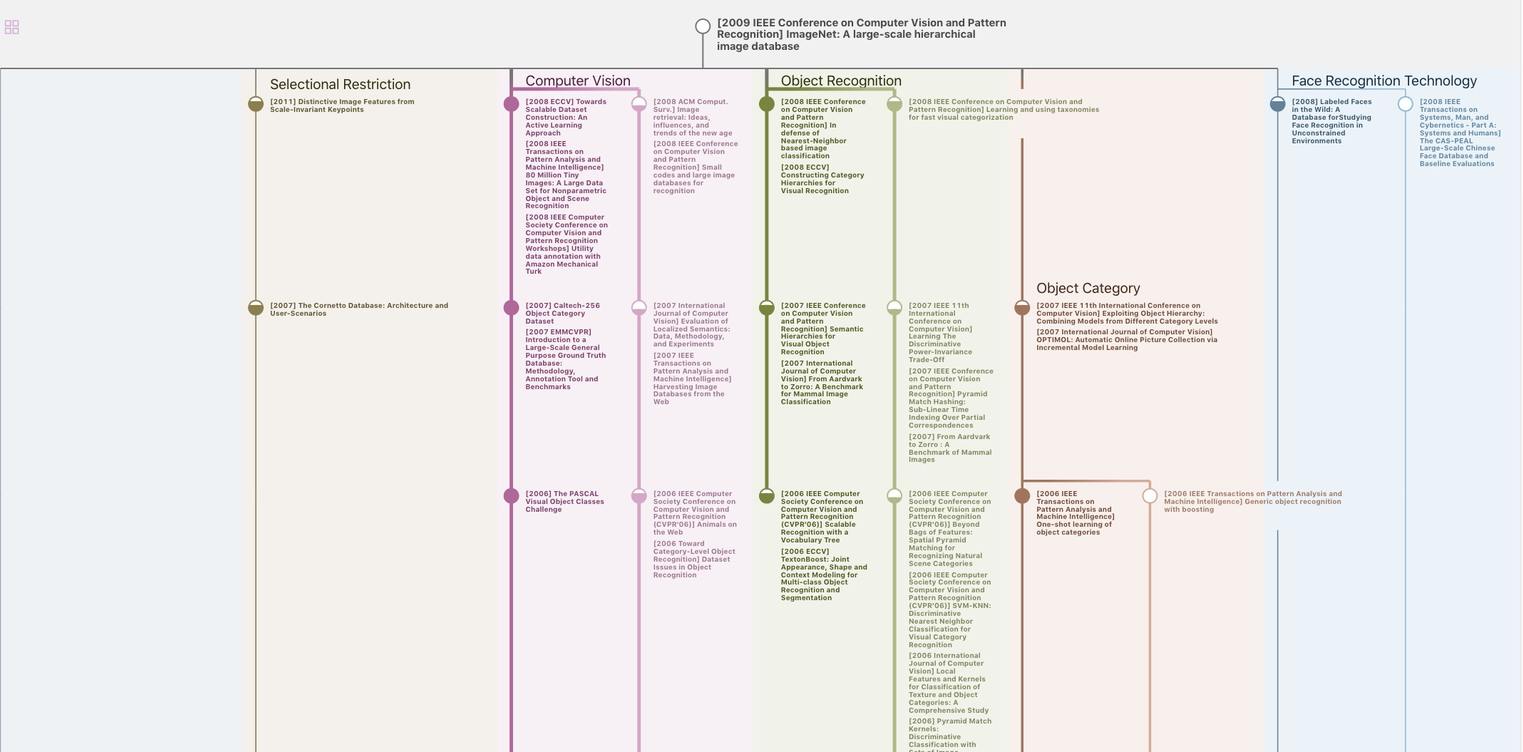
生成溯源树,研究论文发展脉络
Chat Paper
正在生成论文摘要