Efficient Fully Distributed Federated Learning with Adaptive Local Links
arxiv(2022)
摘要
Nowadays, data-driven, machine and deep learning approaches have provided unprecedented performance in various complex tasks, including image classification and object detection, and in a variety of application areas, like autonomous vehicles, medical imaging and wireless communications. Traditionally, such approaches have been deployed, along with the involved datasets, on standalone devices. Recently, a shift has been observed towards the so-called Edge Machine Learning, in which centralized architectures are adopted that allow multiple devices with local computational and storage resources to collaborate with the assistance of a centralized server. The well-known federated learning approach is able to utilize such architectures by allowing the exchange of only parameters with the server, while keeping the datasets private to each contributing device. In this work, we propose a fully distributed, diffusion-based learning algorithm that does not require a central server and propose an adaptive combination rule for the cooperation of the devices. By adopting a classification task on the MNIST dataset, the efficacy of the proposed algorithm over corresponding counterparts is demonstrated via the reduction of the number of collaboration rounds required to achieve an acceptable accuracy level in non- IID dataset scenarios.
更多查看译文
关键词
federated learning,adaptive local links
AI 理解论文
溯源树
样例
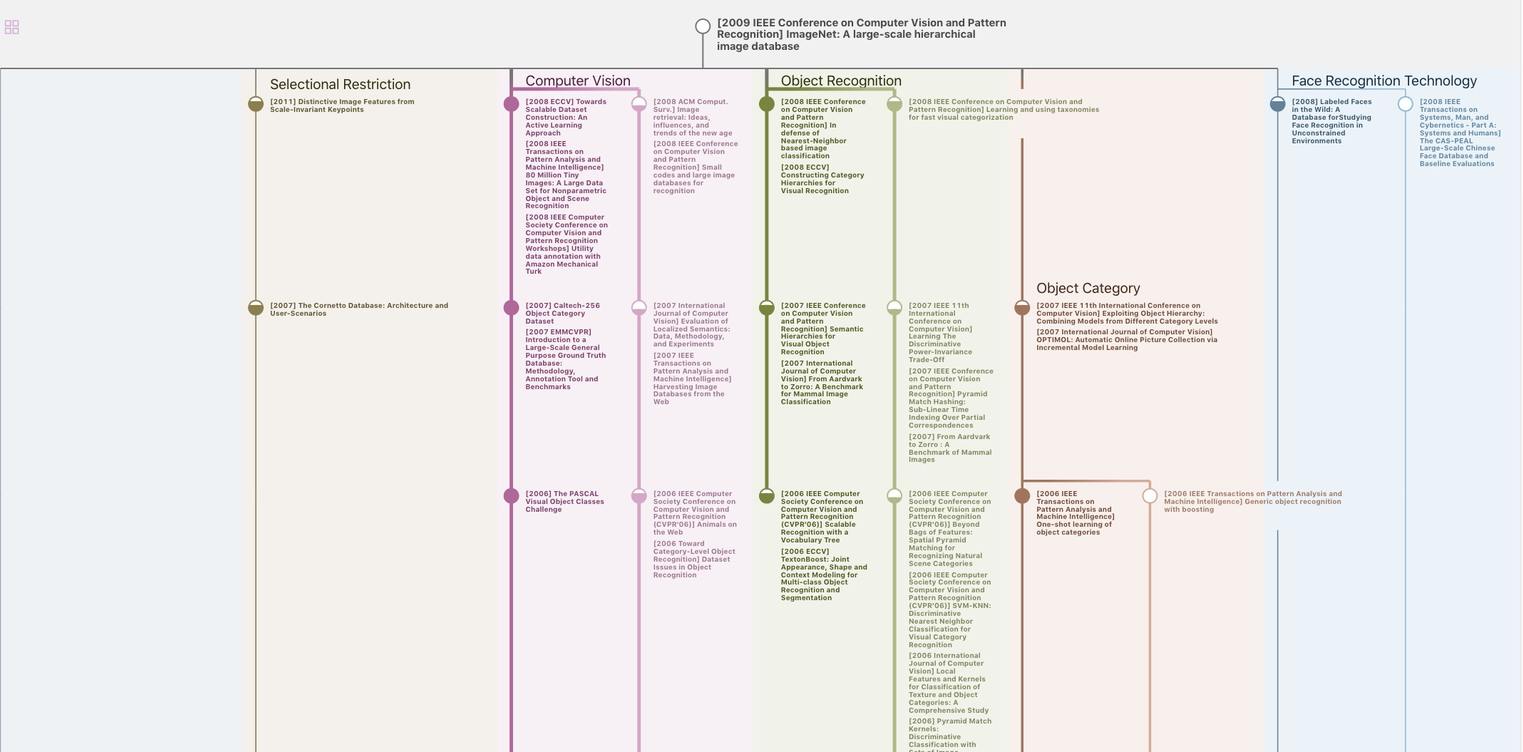
生成溯源树,研究论文发展脉络
Chat Paper
正在生成论文摘要