Privacy-Preserving Personalized Fitness Recommender System P(3)FitRec:76. A Multi-level Deep Learning Approach
arxiv(2023)
摘要
Recommender systems have been successfully used in many domains with the help of machine learning algorithms. However, such applications tend to use multi-dimensional user data, which has raised widespread concerns about the breach of users' privacy. Meanwhile, wearable technologies have enabled users to collect fitness-related data through embedded sensors to monitor their conditions or achieve personalized fitness goals. In this article, we propose a novel privacy-aware personalized fitness recommender system. We introduce a multi-level deep learning framework that learns important features from a large-scale real fitness dataset that is collected from wearable Internet of Things (IoT) devices to derive intelligent fitness recommendations. Unlike most existing approaches, our approach achieves personalization by inferring the fitness characteristics of users from sensory data, minimizing the need for explicitly collecting user identity or biometric information, such as name, age, height, and weight. Our proposed models and algorithms predict (a) personalized exercise distance recommendations to help users to achieve target calories, (b) personalized speed sequence recommendations to adjust exercise speed given the nature of the exercise and the chosen route, and (c) personalized heart rate sequence to guide the user of the potential health status for future exercises. Our experimental evaluation on a real-world Fitbit dataset demonstrated high accuracy in predicting exercise distance, speed sequence, and heart rate sequence compared with similar studies.1 Furthermore, our approach is novel compared with existing studies, as it does not require collecting and using users' sensitive information. Thus, it preserves the users' privacy.
更多查看译文
关键词
Personalization,fitness,recommender system,deep learning,sensors
AI 理解论文
溯源树
样例
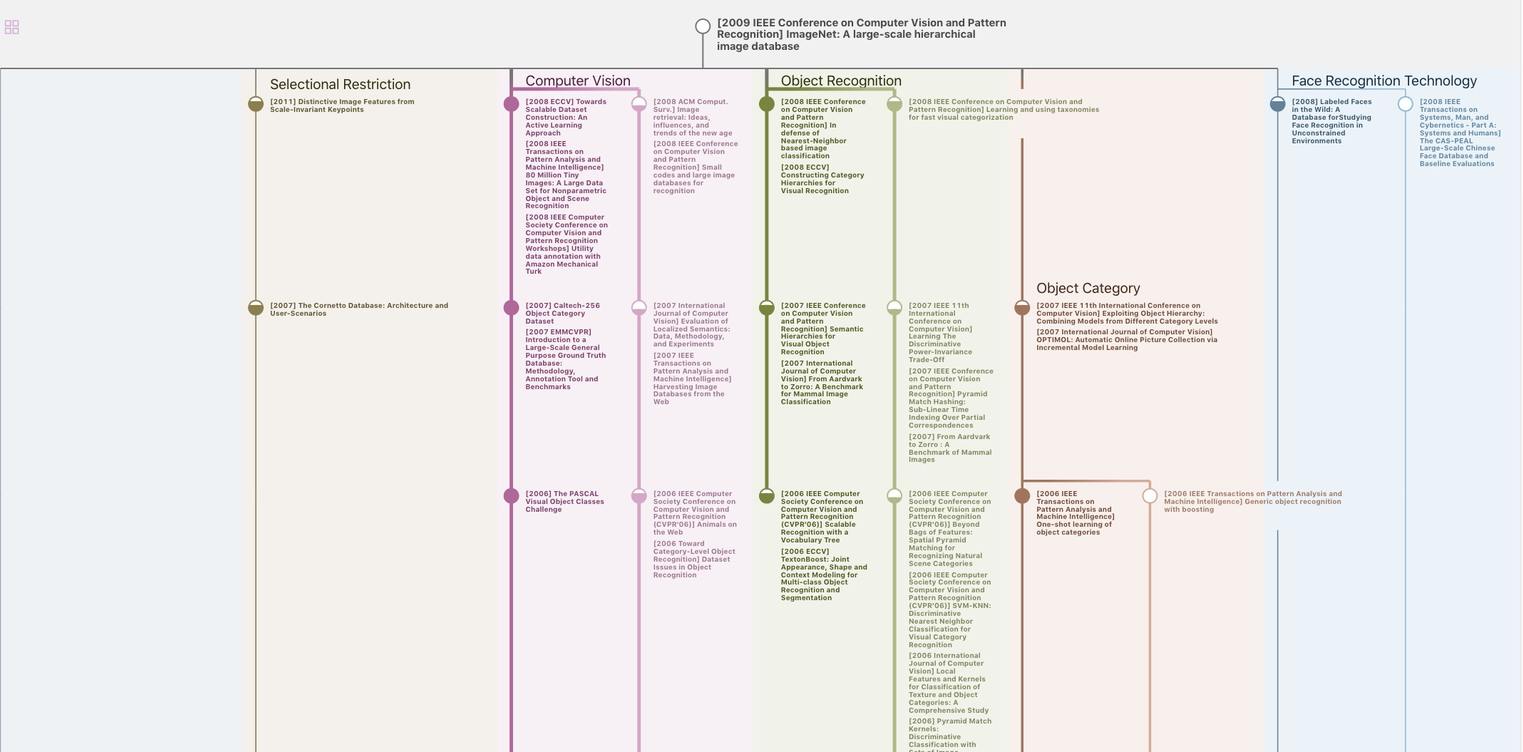
生成溯源树,研究论文发展脉络
Chat Paper
正在生成论文摘要