On Adversarial Robustness of Large-scale Audio Visual Learning
arxiv(2022)
摘要
As audio-visual systems are being deployed for safety-critical tasks such as surveillance and malicious content filtering, their robustness remains an under-studied area. Existing published work on robustness either does not scale to large-scale dataset, or does not deal with multiple modalities. This work aims to study several key questions related to multi-modal learning through the lens of robustness: 1) Are multi-modal models necessarily more robust than uni-modal models? 2) How to efficiently measure the robustness of multi-modal learning? 3) How to fuse different modalities to achieve a more robust multi-modal model? To understand the robustness of the multi-modal model in a large-scale setting, we propose a density-based metric, and a convexity metric to efficiently measure the distribution of each modality in high-dimensional latent space. Our work provides a theoretical intuition together with empirical evidence showing how multi-modal fusion affects adversarial robustness through these metrics. We further devise a mix-up strategy based on our metrics to improve the robustness of the trained model. Our experiments on AudioSet and Kinetics-Sounds verify our hypothesis that multi-modal models are not necessarily more robust than their uni-modal counterparts in the face of adversarial examples. We also observe our mix-up trained method could achieve as much protection as traditional adversarial training, offering a computationally cheap alternative. Implementation: https://github.com/lijuncheng16/AudioSetDoneRight
更多查看译文
关键词
adversarial robustness,large-scale audio visual learning,audio-visual systems,large-scale dataset,multiple modalities,multimodal learning,uni-modal models,robust multimodal model,multimodal fusion,uni-modal counterparts
AI 理解论文
溯源树
样例
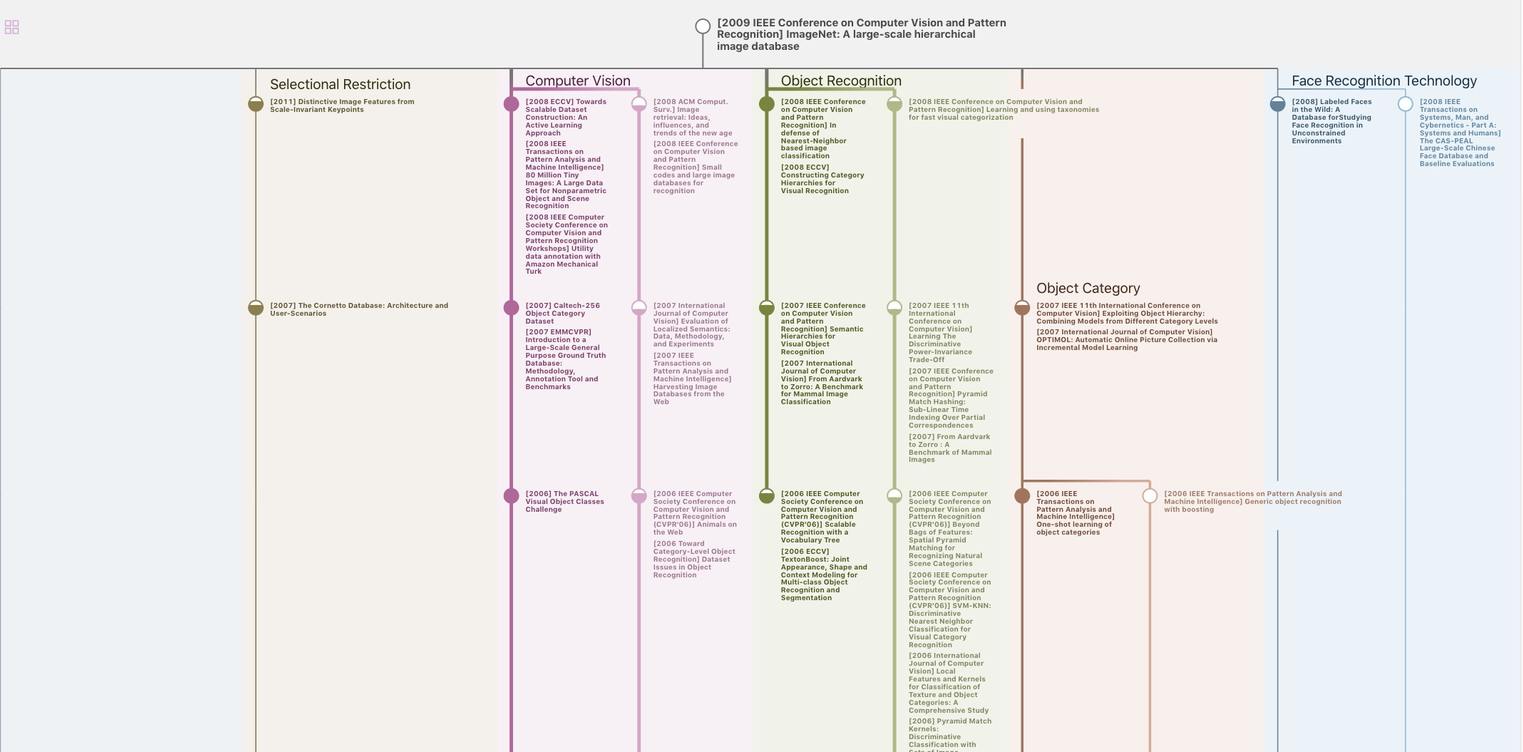
生成溯源树,研究论文发展脉络
Chat Paper
正在生成论文摘要