Visual Prompt Tuning.
European Conference on Computer Vision(2022)
摘要
The current modus operandi in adapting pre-trained models involves updating all the backbone parameters, i.e., full fine-tuning. This paper introduces Visual Prompt Tuning (VPT) as an efficient and effective alternative to full fine-tuning for large-scale Transformer models in vision. Taking inspiration from recent advances in efficiently tuning large language models, VPT introduces only a small amount (less than 1% of model parameters) of trainable parameters in the input space while keeping the model backbone frozen. Via extensive experiments on a wide variety of downstream recognition tasks, we show that VPT achieves significant performance gains compared to other parameter efficient tuning protocols. Most importantly, VPT even outperforms full fine-tuning in many cases across model capacities and training data scales, while reducing per-task storage cost. Code is available at github.com/kmnp/vpt.
更多查看译文
关键词
prompt,visual
AI 理解论文
溯源树
样例
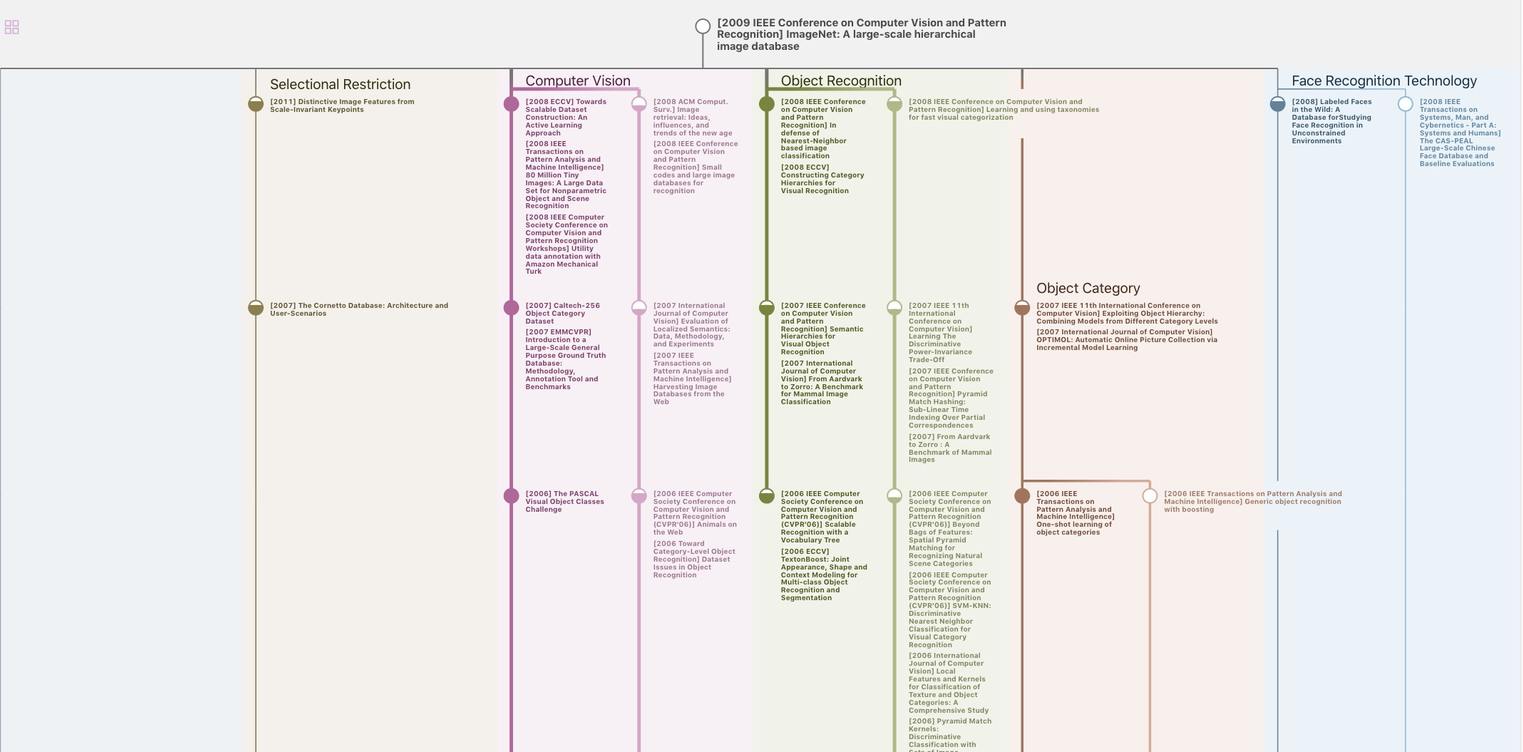
生成溯源树,研究论文发展脉络
Chat Paper
正在生成论文摘要