Do Speech-Based Collaboration Analytics Generalize Across Task Contexts?
LAK(2022)
摘要
We investigated the generalizability of language-based analytics models across two collaborative problem solving (CPS) tasks: an educational physics game and a block programming challenge. We analyzed a dataset of 95 triads (N=285) who used videoconferencing to collaborate on both tasks for an hour. We trained supervised natural language processing classifiers on automatic speech recognition transcripts to predict the human-coded CPS facets (skills) of constructing shared knowledge, negotiation / coordination, and maintaining team function. We tested three methods for representing collaborative discourse: (1) deep transfer learning (using BERT), (2) n-grams (counts of words/phrases), and (3) word categories (using the Linguistic Inquiry Word Count [LIWC] dictionary). We found that the BERT and LIWC methods generalized across tasks with only a small degradation in performance (Transfer Ratio of.93 with 1 indicating perfect transfer), while the n-grams had limited generalizability (Transfer Ratio of.86), suggesting overfitting to task-specific language. We discuss the implications of our findings for deploying language-based collaboration analytics in authentic educational environments.
更多查看译文
关键词
Natural language processing,Collaboration analytics,Collaborative problem solving
AI 理解论文
溯源树
样例
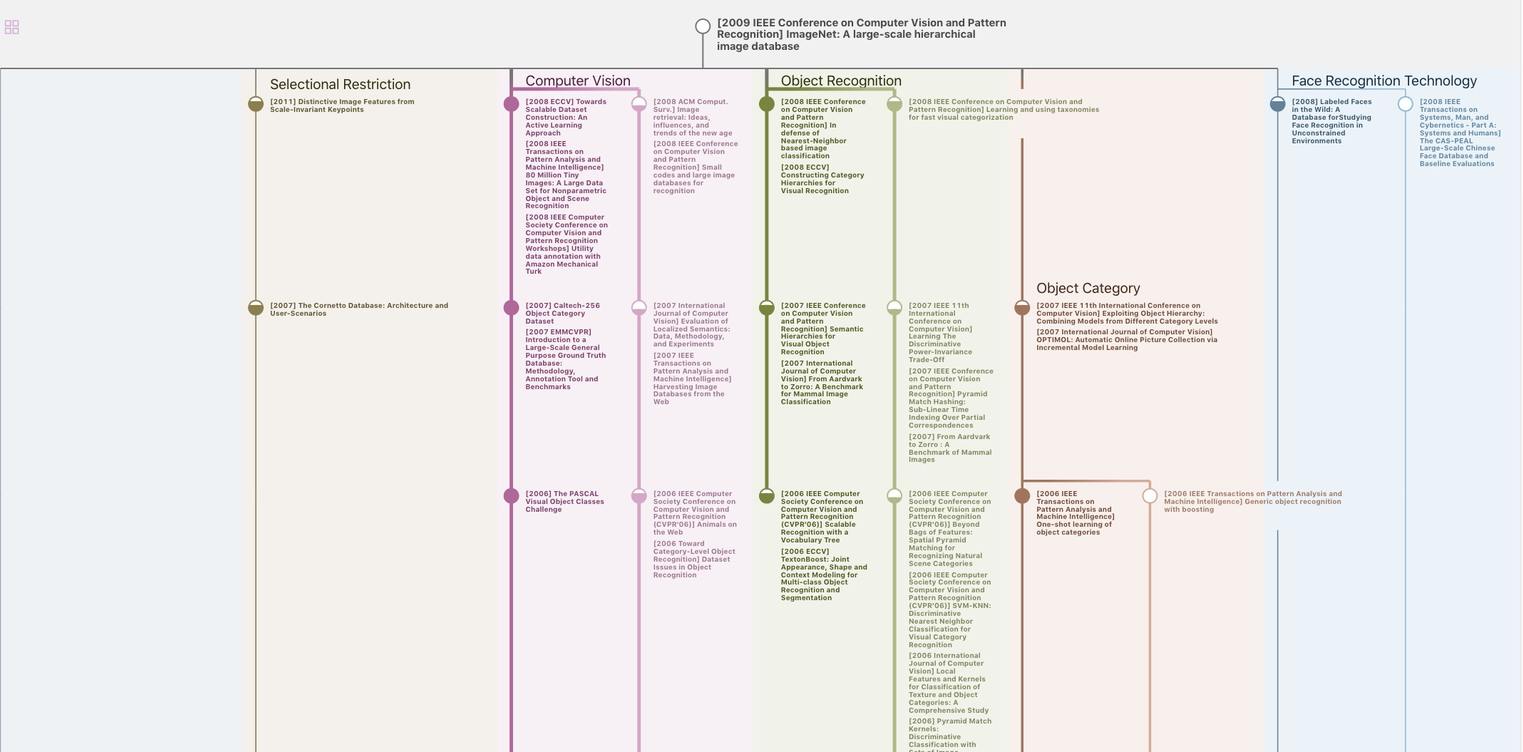
生成溯源树,研究论文发展脉络
Chat Paper
正在生成论文摘要