Training Hybrid Models on Noisy Transliterated Transcripts for Code-Switched Speech Recognition.
Interspeech(2021)
摘要
In this paper, we describe the JHU-GoVivace submission for subtask 2 (code-switching task) of the Multilingual and Code switching ASR challenges for low resource Indian languages. We built a hybrid HMM-DNN system with several improvements over the provided baseline in terms of lexical, language, and acoustic modeling. For lexical modeling, we investigate using unified pronunciations and phonesets derived from the baseline lexicon and publicly available Wikipron lexicons in Bengali and Hindi to expand the pronunciation lexicons. We explore several neural network architectures, along with supervised pretraining and multilingual training for acoustic modeling. We also describe how we used large externally crawled web text for language modeling. Since the challenge data contain artefacts such as misalignments, various data cleanup methods are explored, including acoustic-driven pronunciation learning to help discover Indian-accented pronunciations for English words as well as transcribed punctuation. As a result of these efforts, our best systems achieve transliterated WERs of 19.5% and 23.2% on the non-duplicated development sets for Hindi-English and Bengali-English, respectively.
更多查看译文
关键词
speech recognition,code-switching,transliteration,pronunciation modeling
AI 理解论文
溯源树
样例
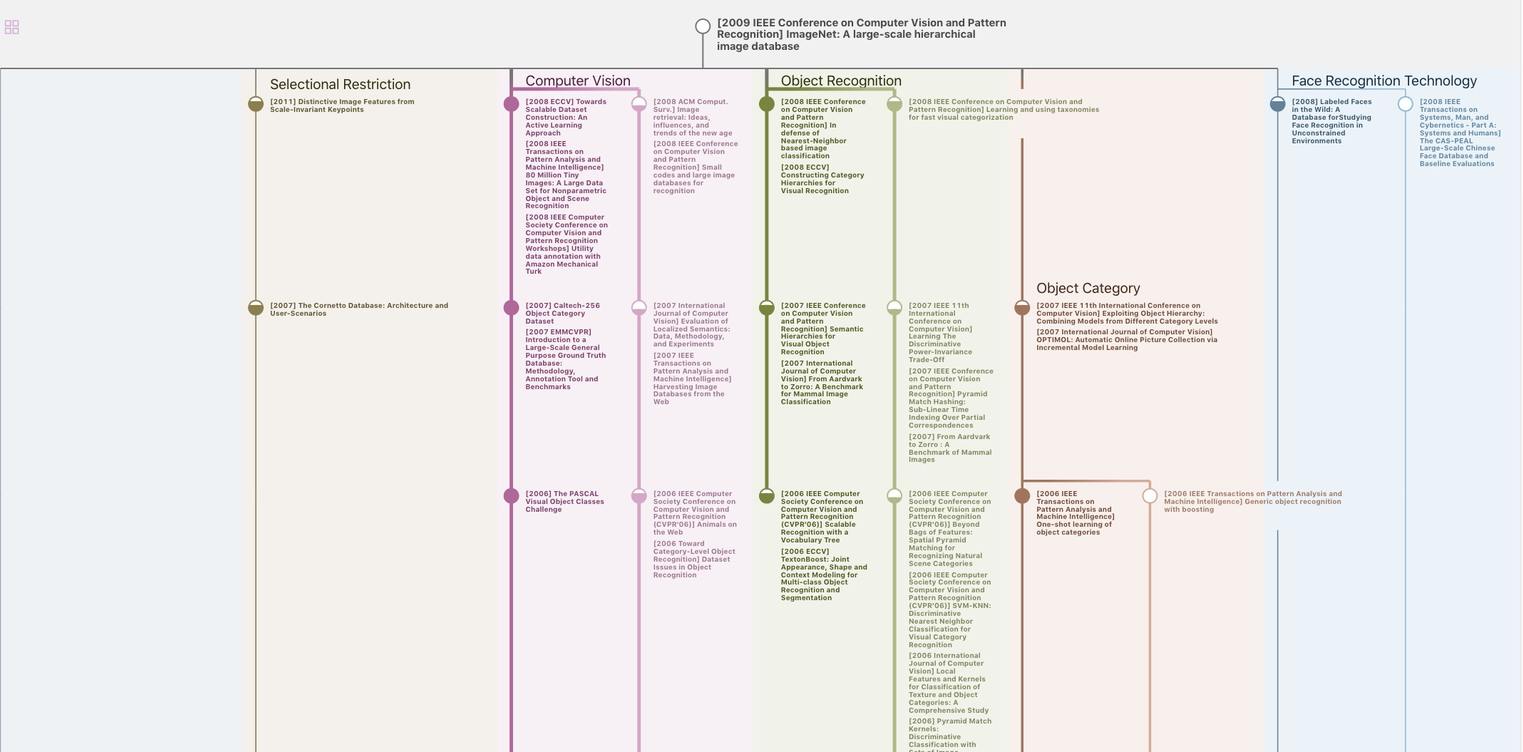
生成溯源树,研究论文发展脉络
Chat Paper
正在生成论文摘要