Automatic Speech Recognition of Disordered Speech - Personalized Models Outperforming Human Listeners on Short Phrases.
Interspeech(2021)
摘要
This study evaluated the accuracy of personalized automatic speech recognition (ASR) for recognizing disordered speech from a large cohort of individuals with a wide range of underlying etiologies using an open vocabulary. The performance of these models was benchmarked relative to that of expert human transcribers and two different speaker-independent ASR models trained on typical speech. 432 individuals with self-reported disordered speech recorded at least 300 short phrases using a web-based application. Word error rates (WERs) were estimated for three different ASR models and for human transcribers. Metadata were collected to evaluate the potential impact of participants, atypical speech characteristics, and technical factors on recognition accuracy. Personalized models outperformed human transcribers with median and max recognition accuracy gains of 9% and 80%, respectively. The accuracies of personalized models were high (median WER: 4.6%) and better than those of speaker-independent models (median WER: 31%). The most significant improvements were for the most severely affected speakers. Low signal-to-noise ratio and fewer training utterances were associated with poor word recognition, even for speakers with mild speech impairments. Our results demonstrate the efficacy of personalized ASR models in recognizing a wide range of speech impairments and severities and using an open vocabulary.
更多查看译文
关键词
speech recognition,speech disorders,personalized models,automatic speech recognition
AI 理解论文
溯源树
样例
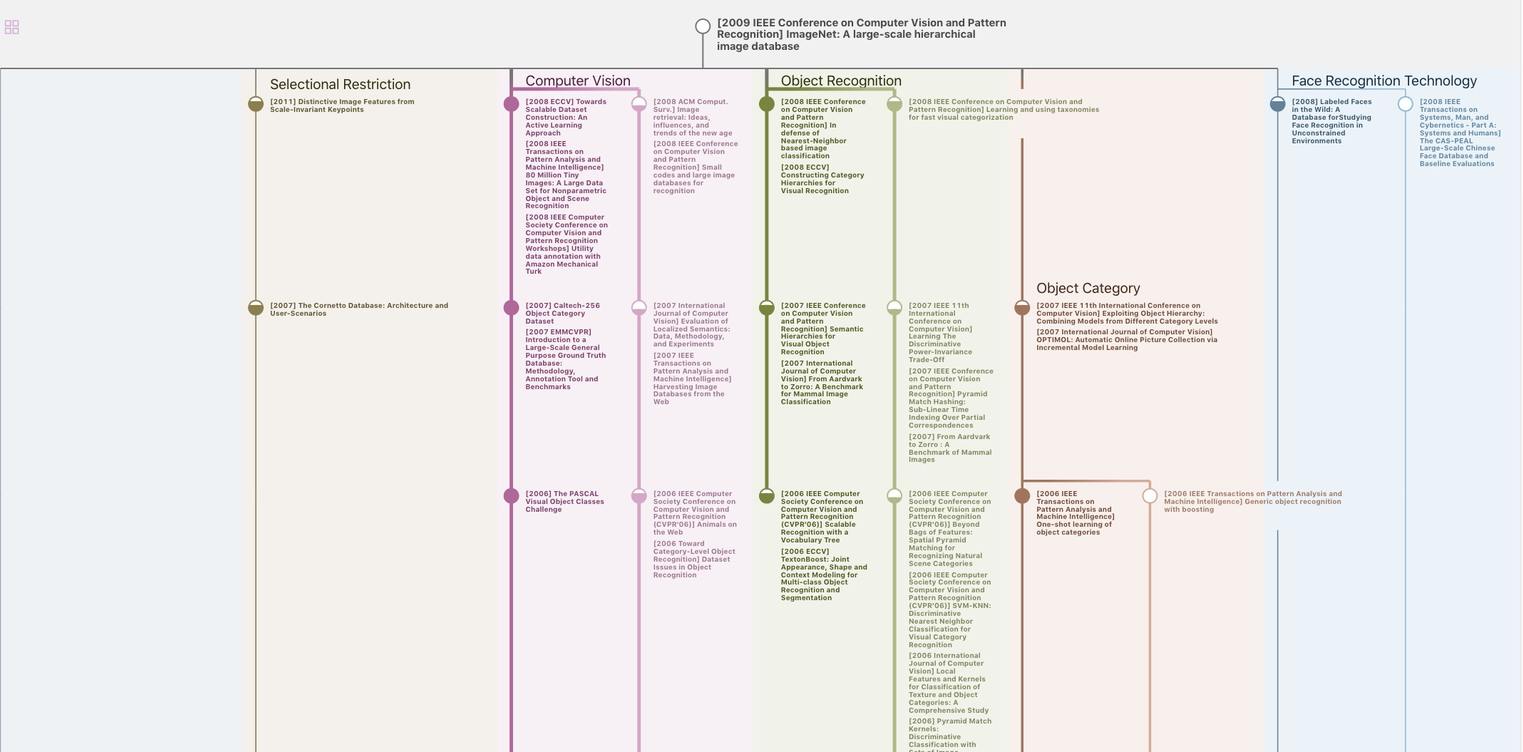
生成溯源树,研究论文发展脉络
Chat Paper
正在生成论文摘要