The Impact of Intent Distribution Mismatch on Semi-Supervised Spoken Language Understanding.
Interspeech(2021)
摘要
With the expanding role of voice-controlled devices, bootstrapping spoken language understanding models from little labeled data becomes essential. Semi-supervised learning is a common technique to improve model performance when labeled data is scarce. In a real-world production system, the labeled data and the online test data often may come from different distributions. In this work, we use semi-supervised learning based on pseudo-labeling with an auxiliary task on incoming unlabeled noisy data, which is closer to the test distribution. We demonstrate empirically that our approach can mitigate negative effects arising from training with non-representative labeled data as well as the negative impacts of noises in the data, which are introduced by pseudo-labeling and automatic speech recognition.
更多查看译文
AI 理解论文
溯源树
样例
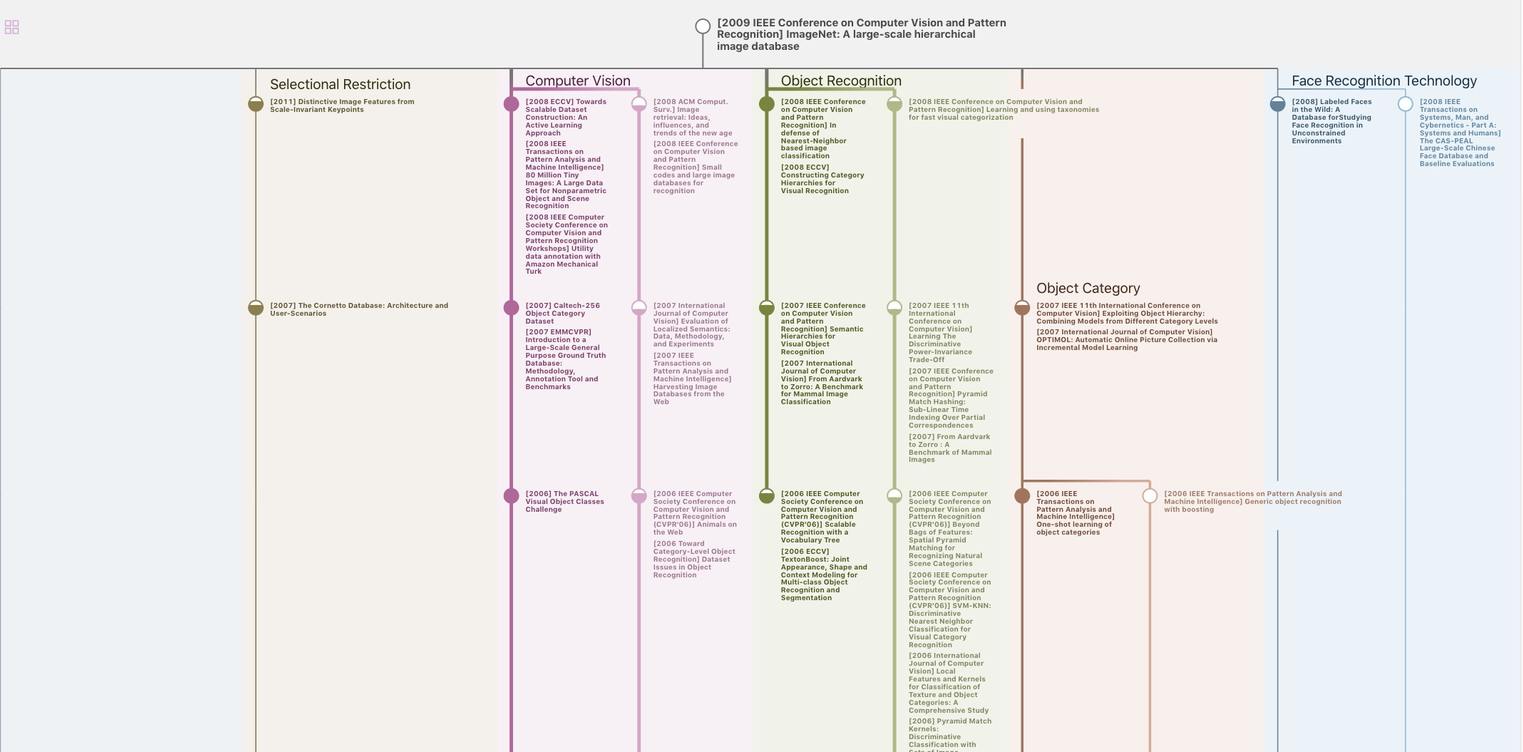
生成溯源树,研究论文发展脉络
Chat Paper
正在生成论文摘要