KR-LISTA - Re-Thinking Unrolling for Covariance-Driven Sparse Inverse Problems.
ACSCC(2021)
摘要
This paper considers the problem of joint support recovery in Multiple Measurement Vector (MMV) models. We show that by exploiting correlation priors, one can boost the performance and computational cost of unrolled data-driven techniques for joint support recovery. We propose a novel unrolling of the Iterative Shrinkage Thresholding Algorithm (ISTA) for correlation-aware support recovery, which preserves the special "Khatri-Rao" structure that underlies the model. The proposed network, termed as "KR-LISTA", provides a parameter-efficient unrolling which enables training with limited data. Our numerical simulations demonstrate the effectiveness of KR-LISTA at test time for different values of SNR, and support sizes which were not provided to the network during training. In addition, KR-LISTA is also seen to be effective even in presence of model uncertainties.
1
更多查看译文
关键词
Joint Support Recovery,Multiple Measurement Vector,Correlation-awareness,Iterative Shrinkage Thresholding Algorithm (ISTA),Learned ISTA (LISTA),Khatri-Rao Product
AI 理解论文
溯源树
样例
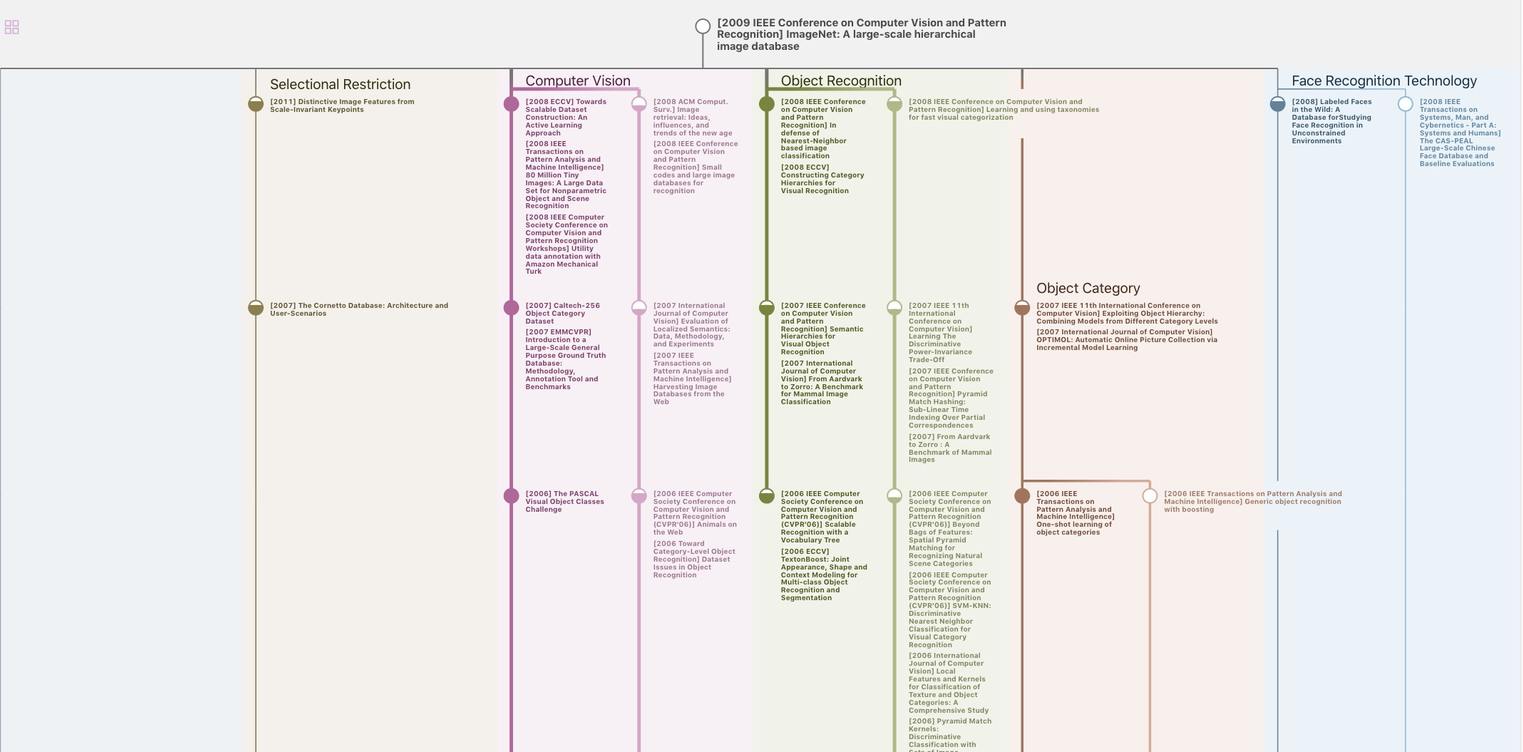
生成溯源树,研究论文发展脉络
Chat Paper
正在生成论文摘要