Randomized Linear Programming for Tabular Average-Cost Multi-agent Reinforcement Learning.
ACSCC(2021)
摘要
We focus on multi-agent reinforcement learning in tabular average-cost settings: a team of agents sequentially interacts with the environment and observes localized incentives. The setting we focus on is one in which the global reward is a sum of all local rewards, the joint policy factorizes into agents' marginals, and full observability. To date, exceptionally few global optimality guarantees exist for this simple setting, as most results, asymptotic or non-asymptotic, yield convergence to stationarity under parameterized settings for possibly large/continuous spaces. To strengthen performance guarantees in MARL, we focus on linear programming (LP) reformulations of RL for which stochastic primal-dual method has recently been shown to achieve optimal sample complexity in the centralized tabular case. We develop multi-agent LP extensions, whereby agents solve their local saddle point problems and then compose their variable estimates with weighted averaging steps to diffuse information between agents across time. We establish that the number of samples required to attain near-globally optimal solutions matches tight dependencies on the cardinality of the state and action spaces, and exhibits classical scalings with the size of the team in accordance with multi-agent optimization. Experiments then demonstrate the merits of this approach for cooperative navigation problems.
更多查看译文
关键词
average-cost multiagent reinforcement learning,average-cost settings,localized incentives,global reward,local rewards,joint policy,observability,parameterized settings,optimal sample complexity,centralized tabular case,multiagent LP extensions,local saddle point problems,weighted averaging steps,near-globally optimal solutions,multiagent optimization,global optimality guarantees
AI 理解论文
溯源树
样例
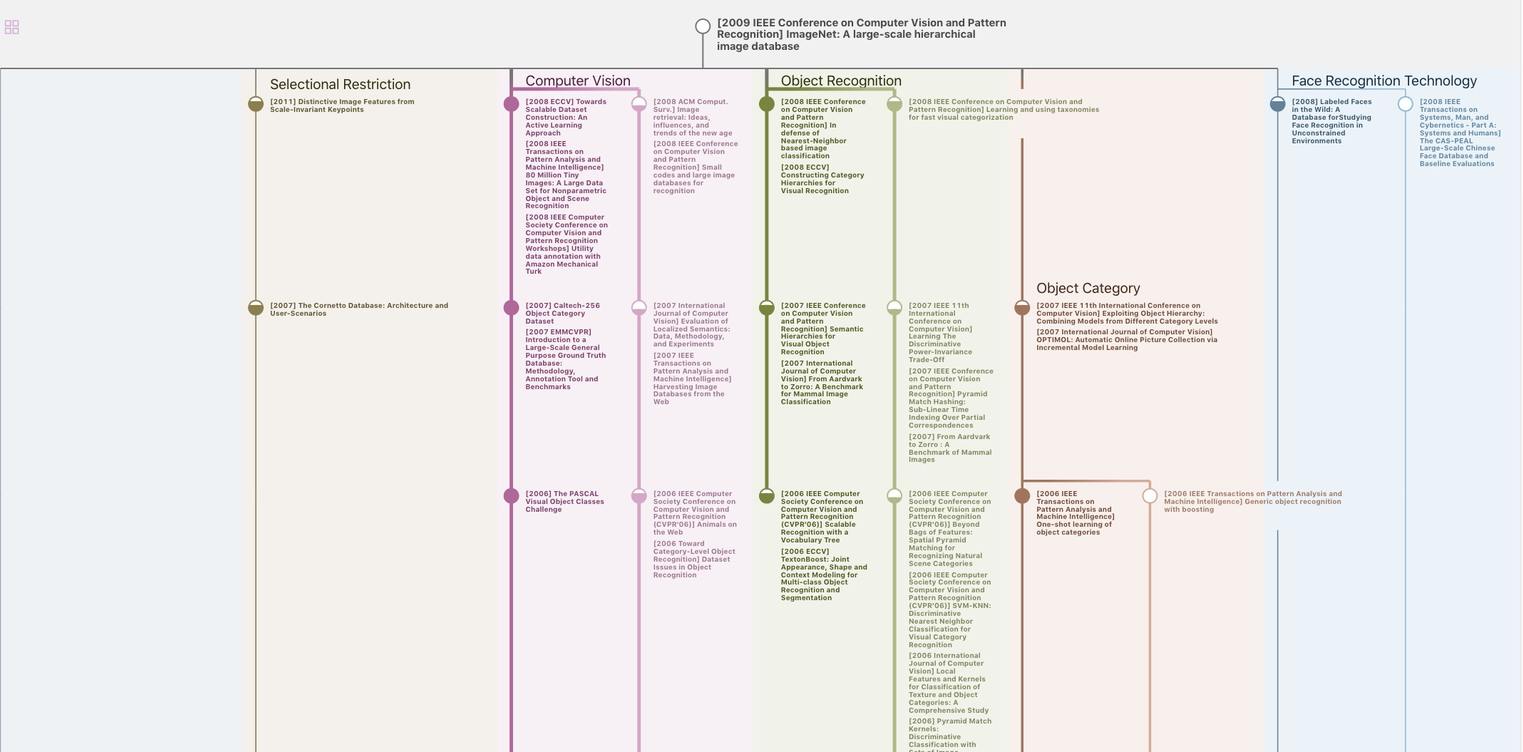
生成溯源树,研究论文发展脉络
Chat Paper
正在生成论文摘要